Cascade Attentive Dropout for Weakly Supervised Object Detection
arxiv(2023)
摘要
Weakly supervised object detection (WSOD) aims to classify and locate objects with only image-level supervision. Many WSOD approaches adopt multiple instance learning as the initial model, which is prone to converge to the most discriminative object regions while ignoring the whole object, and therefore reduce the model detection performance. In this paper, a novel cascade attentive dropout strategy is proposed to alleviate the part domination problem, together with an improved global context module. We purposely discard attentive elements in both channel and space dimensions, and capture the inter-pixel and inter-channel dependencies to induce the model to better understand the global context. Extensive experiments have been conducted on the challenging PASCAL VOC 2007 benchmarks, which achieve 49.8% mAP and 66.0% CorLoc, outperforming state-of-the-arts.
更多查看译文
关键词
Weakly supervised object detection, Convolutional neural network, Dropout
AI 理解论文
溯源树
样例
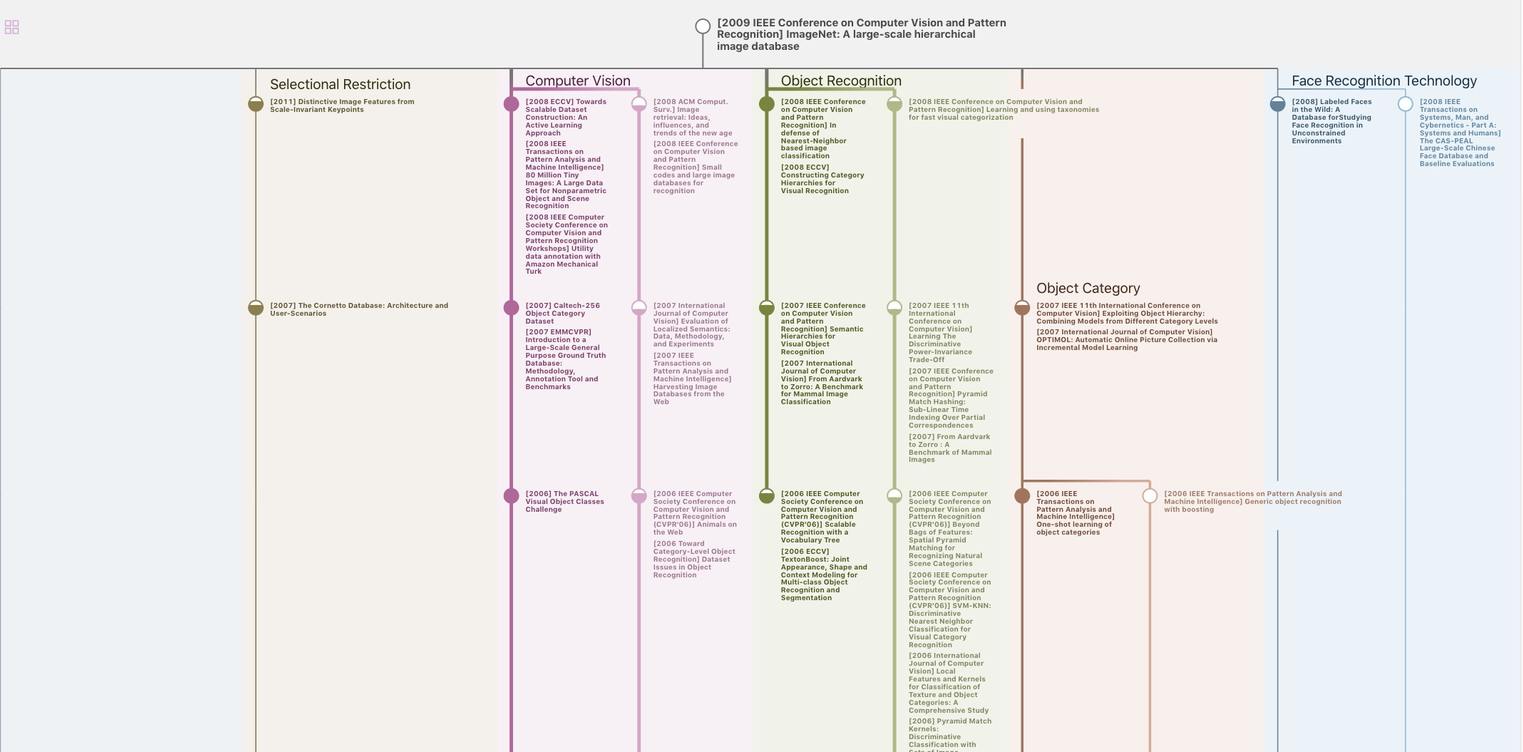
生成溯源树,研究论文发展脉络
Chat Paper
正在生成论文摘要