Meningiomas: Preoperative predictive histopathological grading based on radiomics of MRI
Magnetic Resonance Imaging(2021)
摘要
Purpose: We aimed to develop a radiomics model to predict the histopathological grading of meningiomas by magnetic resonance imaging (MRI) before surgery.Methods: We recruited 131 patients with pathological diagnosis of meningiomas. All the patients had undergone MRI before surgery on a 3.0 T MRI scanner to obtain T1 fluidattenuated inversion recovery (T1 FLAIR) images, T2-weighted images (T2WI) and T1 FLAIR with contrast enhancement (CE-T1 FLAIR) images covering the whole brain. The removing features with low variance, univariate feature selection, and least absolute shrinkage and selection operator (LASSO) were used to select radiomics features. Six classifiers were used to train the models (logistic regression (LR), k-nearest neighbor (KNN), decision tree (DT), support vector machine (SVM), random forests (RF), and XGBoost), and then 24 models were established using a random verification method to differentiate low-grade from high-grade meningiomas. The performance was assessed by receiver-operating characteristic (ROC) analysis, the f1-score, sensitivity, and specificity.Results: The radiomics features were significantly associated with the histopathological grading. Quantitative imaging features (n = 1409) were extracted, and nine features were selected to predict the grades of meningiomas. The best performance of the radiomics model for the degree of differentiation was obtained by SVM (area under the curve (AUC), 0.956; 95% confidence interval (CI), 0.83-1.00; sensitivity, 0.87; specificity, 0.92; f1-score, 0.90).Conclusion: The radiomics models are of great value in predicting the histopathological grades of meningiomas, and have broad prospects in radiology and clinics.
更多查看译文
关键词
Radiomics,Grading,Meningiomas,MRI
AI 理解论文
溯源树
样例
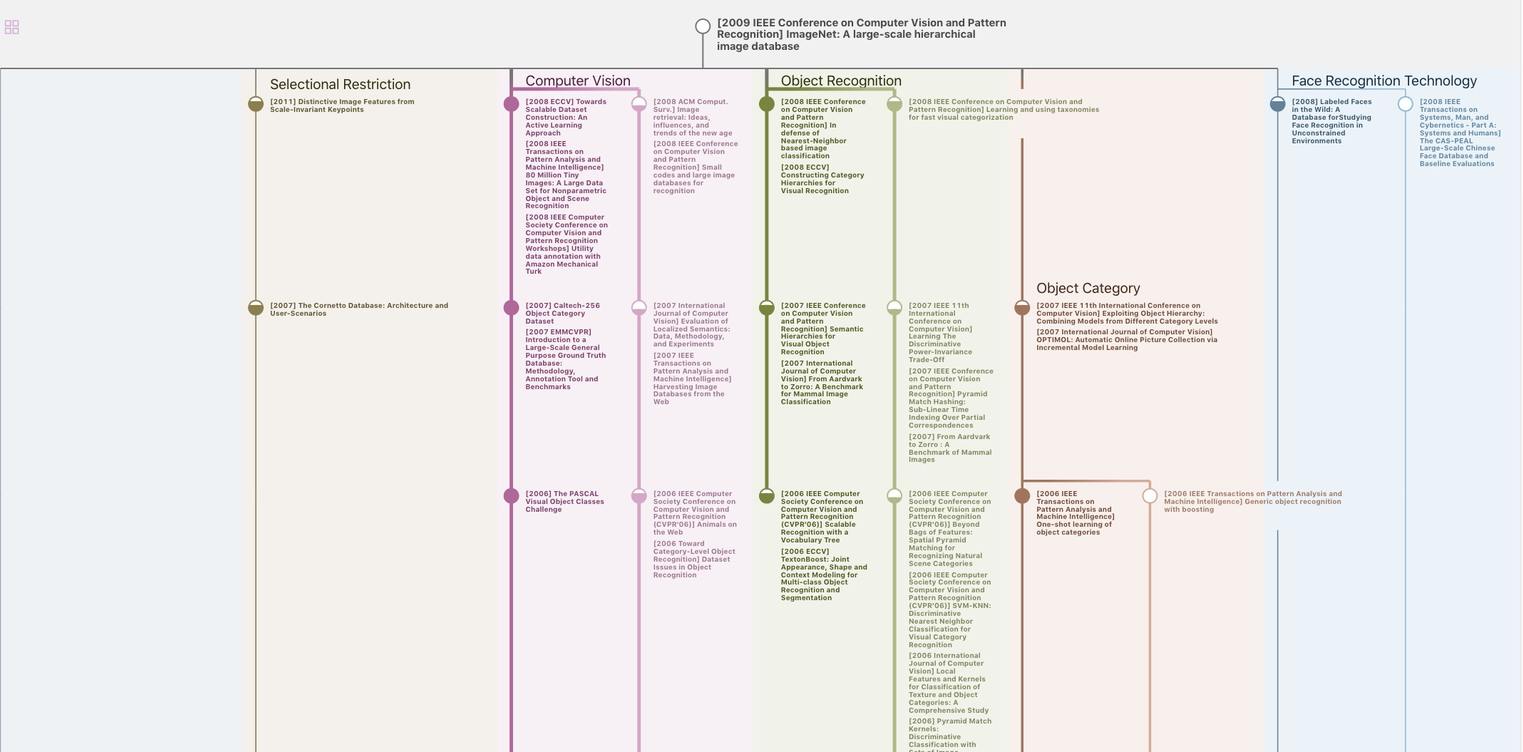
生成溯源树,研究论文发展脉络
Chat Paper
正在生成论文摘要