Experimental Determination And Data-Driven Prediction Of Homotypic Transmembrane Domain Interfaces
COMPUTATIONAL AND STRUCTURAL BIOTECHNOLOGY JOURNAL(2020)
摘要
Interactions between their transmembrane domains (TMDs) frequently support the assembly of single-pass membrane proteins to non-covalent complexes. Yet, the TMD-TMD interactome remains largely uncharted. With a view to predicting homotypic TMD-TMD interfaces from primary structure, we performed a systematic analysis of their physical and evolutionary properties. To this end, we generated a dataset of 50 self-interacting TMDs. This dataset contains interfaces of nine TMDs from bitopic human proteins (Ire1, Armcx6, Tie1, ATP1B1, PTPRO, PTPRU, PTPRG, DDR1, and Siglec7) that were experimentally identified here and combined with literature data. We show that interfacial residues of these homotypic TMD-TMD interfaces tend to be more conserved, coevolved and polar than non-interfacial residues. Further, we suggest for the first time that interface positions are deficient in beta-branched residues, and likely to be located deep in the hydrophobic core of the membrane. Overrepresentation of the GxxxG motif at interfaces is strong, but that of (small)xxx(small) motifs is weak. The multiplicity of these features and the individual character of TMD-TMD interfaces, as uncovered here, prompted us to train a machine learning algorithm. The resulting prediction method, THOIPA (www.thoipa.org), excels in the prediction of key interface residues from evolutionary sequence data. (C) 2020 The Author(s). Published by Elsevier B.V. on behalf of Research Network of Computational and Structural Biotechnology.
更多查看译文
关键词
Protein-protein interaction, TMD interactions, Machine learning, Transmembrane, GxxxG, Co-evolution
AI 理解论文
溯源树
样例
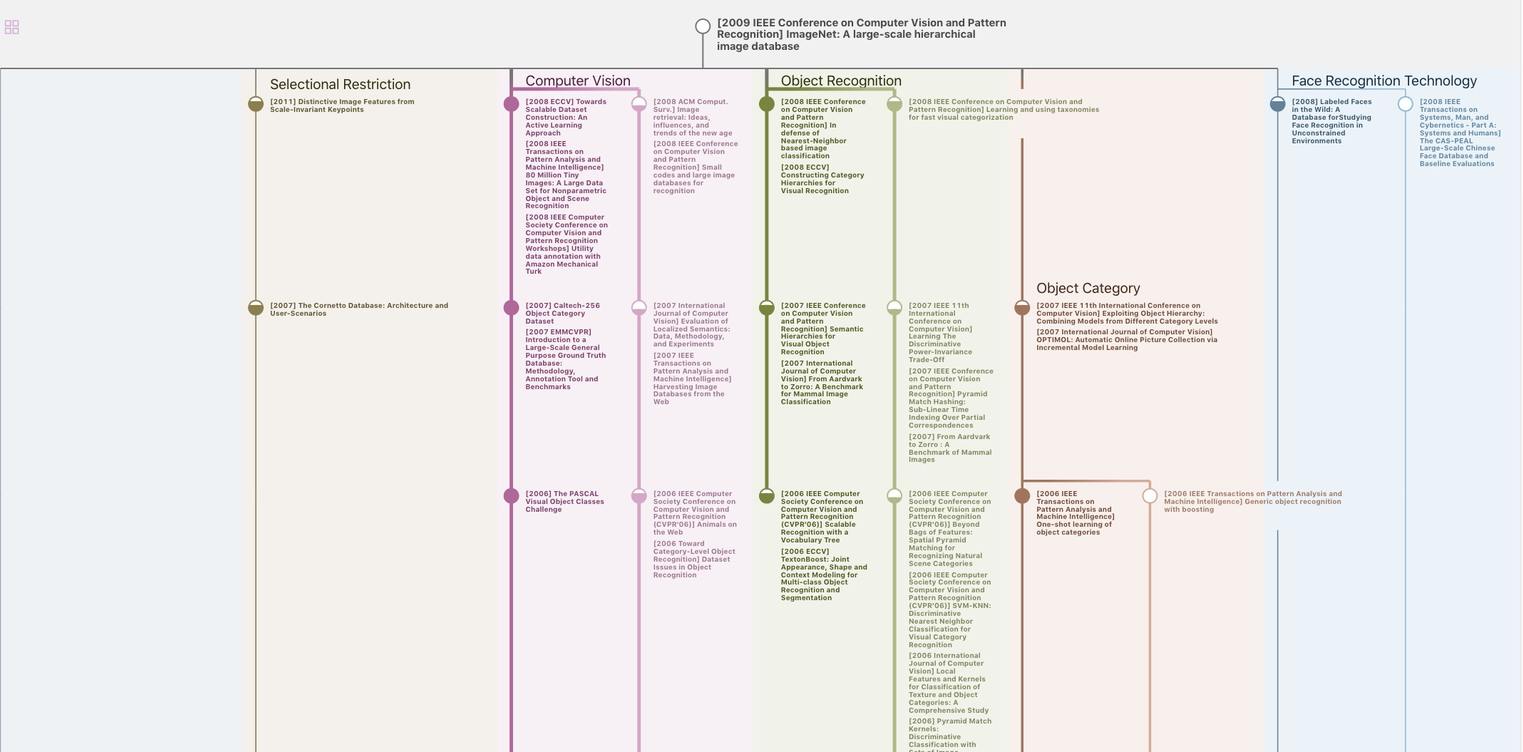
生成溯源树,研究论文发展脉络
Chat Paper
正在生成论文摘要