Coupled Mass-Spectrometry-Based Lipidomics Machine Learning Approach For Early Detection Of Clear Cell Renal Cell Carcinoma
JOURNAL OF PROTEOME RESEARCH(2021)
摘要
A discovery-based lipid profiling study of serum samples from a cohort that included patients with clear cell renal cell carcinoma (ccRCC) stages I, II, III, and IV (n = 112) and controls (n = 52) was performed using ultraperformance liquid chromatography coupled to quadrupole-time-of-flight mass spectrometry and machine learning techniques. Multivariate models based on support vector machines and the LASSO variable selection method yielded two discriminant lipid panels for ccRCC detection and early diagnosis. A 16-lipid panel allowed discriminating ccRCC patients from controls with 95.7% accuracy in a training set under cross-validation and 77.1% accuracy in an independent test set. A second model trained to discriminate early (I and II) from late (III and IV) stage ccRCC yielded a panel of 26 compounds that classified stage I patients from an independent test set with 82.1% accuracy. Thirteen species, including cholic acid, undecylenic acid, lauric acid, LPC(16:0/0:0), and PC(18:2/18:2), identified with level 1 exhibited significantly lower levels in samples from ccRCC patients compared to controls. Moreover, 3 alpha-hydroxy-5 alpha-androstan-17-one 3-sulfate, cis-5-dodecenoic acid, arachidonic acid, cis-13-docosenoic acid, PI(16:0/18:1), PC(16:0/18:2), and PC(O-16:0/20:4) contributed to discriminate early from late ccRCC stage patients. The results are auspicious for early ccRCC diagnosis after validation of the panels in larger and different cohorts.
更多查看译文
关键词
lipidomics, clear cell renal cell carcinoma, biomarkers, ultraperformance liquid chromatography, mass spectrometry, machine learning, support vector machines, LASSO
AI 理解论文
溯源树
样例
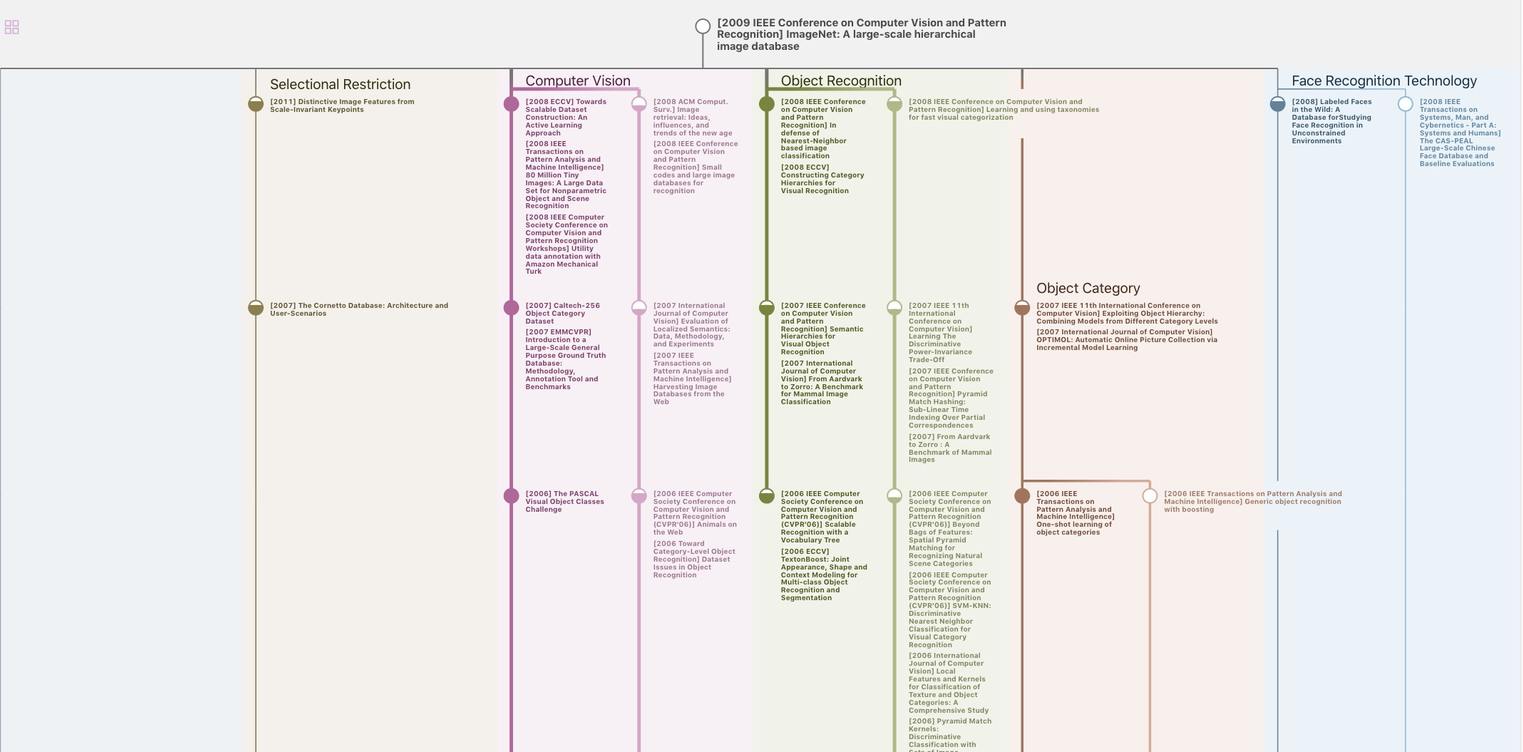
生成溯源树,研究论文发展脉络
Chat Paper
正在生成论文摘要