TRAT: Tracking by attention using spatio-temporal features
Neurocomputing(2022)
摘要
Robust object tracking requires knowledge of tracked objects’ appearance, motion and their evolution over time. Although motion provides distinctive and complementary information especially for fast moving objects, most of the recent tracking architectures primarily focus on the objects’ appearance information. In this paper, we propose a two-stream deep neural network tracker that uses both spatial and temporal features. Our architecture is developed over ATOM tracker and contains two backbones: (i) 2D-CNN network to capture appearance features and (ii) 3D-CNN network to capture motion features. The features returned by the two networks are then fused with attention based Feature Aggregation Module (FAM). Since the whole architecture is unified, it can be trained end-to-end. The experimental results show that the proposed tracker TRAT (TRacking by ATtention) achieves the state-of-the-art performance on most of the benchmarks and it significantly outperforms the baseline ATOM tracker. The source code and pretrained models can be found at https://github.com/Hasan4825/TRAT.
更多查看译文
关键词
Tracking,Two-stream network,3D CNN,Feature aggregation module,Channel attention,Temporal features
AI 理解论文
溯源树
样例
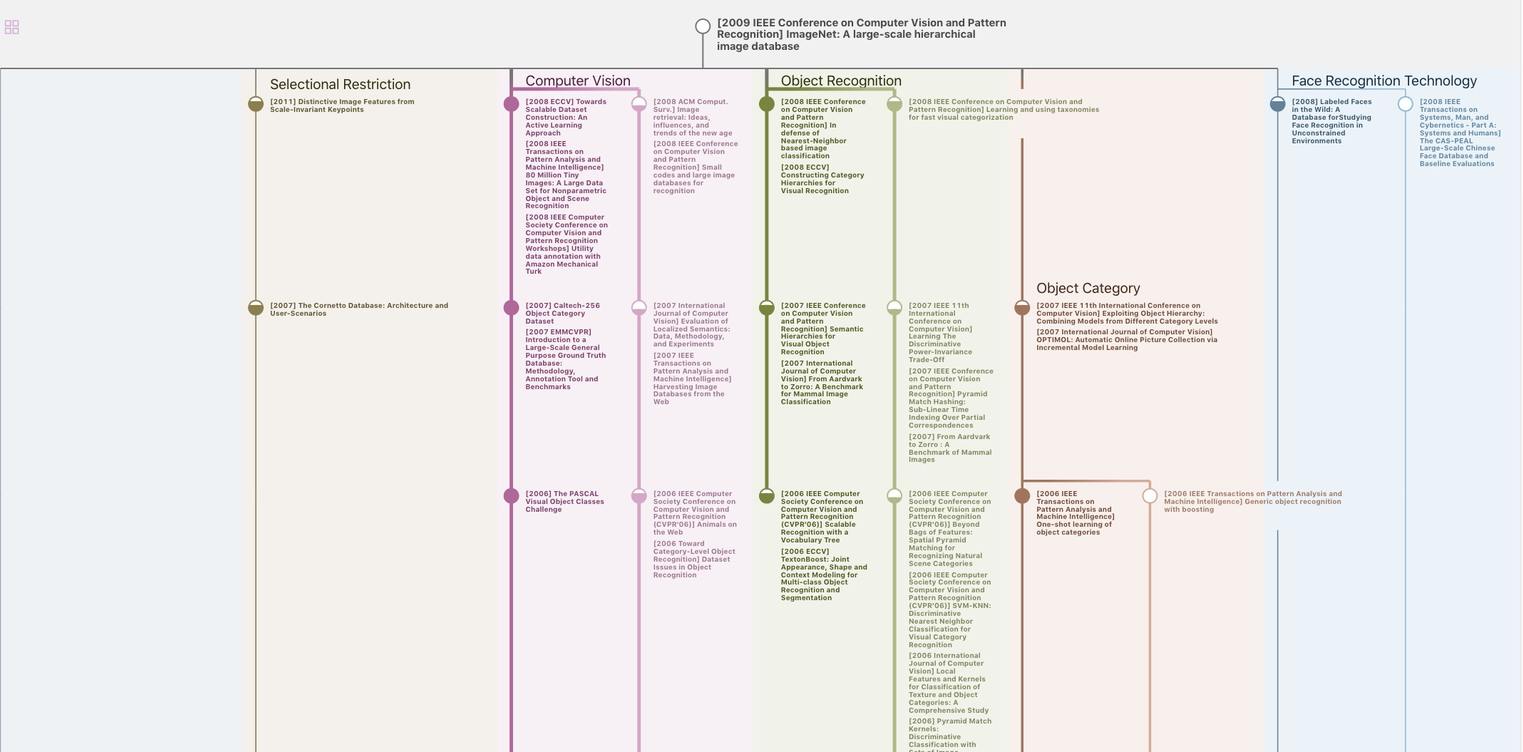
生成溯源树,研究论文发展脉络
Chat Paper
正在生成论文摘要