Explaining The Genetic Causality For Complex Phenotype Via Deep Association Kernel Learning
PATTERNS(2020)
摘要
The genetic effect explains the causality from genetic mutations to the development of complex diseases. Existing genome-wide association study (GWAS) approaches are always built under a linear assumption, restricting their generalization in dissecting complicated causality such as the recessive genetic effect. Therefore, a sophisticated and general GWAS model that can work with different types of genetic effects is highly desired. Here, we introduce a deep association kernel learning (DAK) model to enable automatic causal genotype encoding for GWAS at pathway level. DAK can detect both common and rare variants with complicated genetic effects where existing approaches fail. When applied to four real-world GWAS datasets including cancers and schizophrenia, our DAK discovered potential casual pathways, including the association between dilated cardiomyopathy pathway and schizophrenia.
更多查看译文
关键词
association analysis,deep learning,disease causality,genome-wide association studies,kernel learning
AI 理解论文
溯源树
样例
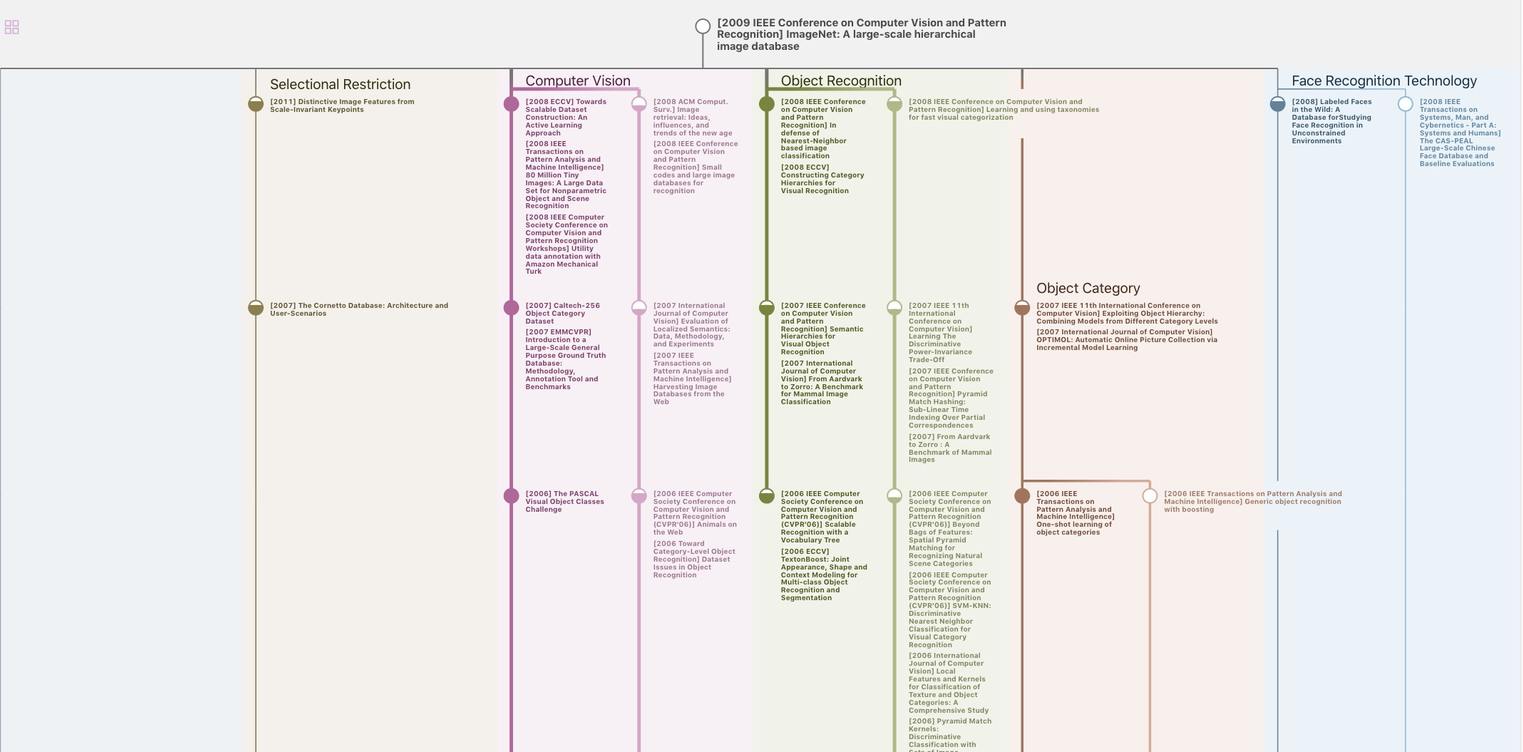
生成溯源树,研究论文发展脉络
Chat Paper
正在生成论文摘要