A New Similarity Space Tailored for Supervised Deep Metric Learning
ACM Transactions on Intelligent Systems and Technology(2023)
摘要
AbstractWe propose a novel deep metric learning method. Differently from many works in this area, we define a novel latent space obtained through an autoencoder. The new space, namely S-space, is divided into different regions describing positions where pairs of objects are similar/dissimilar. We locate makers to identify these regions and estimate the similarities between objects through a kernel-based Cauchy distribution to measure the markers’ distance and the new data representation. In our approach, we simultaneously estimate the markers’ position in the S-space and represent the objects in the same space. Moreover, we propose a new regularization function to prevent similar markers from collapsing altogether. Our method emphasizes the group property (separability) while preserving instance representativity. We present evidence that our proposal can represent complex spaces, for instance, when groups of similar objects are located in disjoint regions. We compare our proposal to nine different distance metric learning approaches (four of them are based on deep learning) on 28 real-world heterogeneous datasets. According to the four quantitative metrics used, our method overcomes all of the nine strategies from the literature.
更多查看译文
关键词
Similarity space,deep metric learning,latent feature space,regularization function
AI 理解论文
溯源树
样例
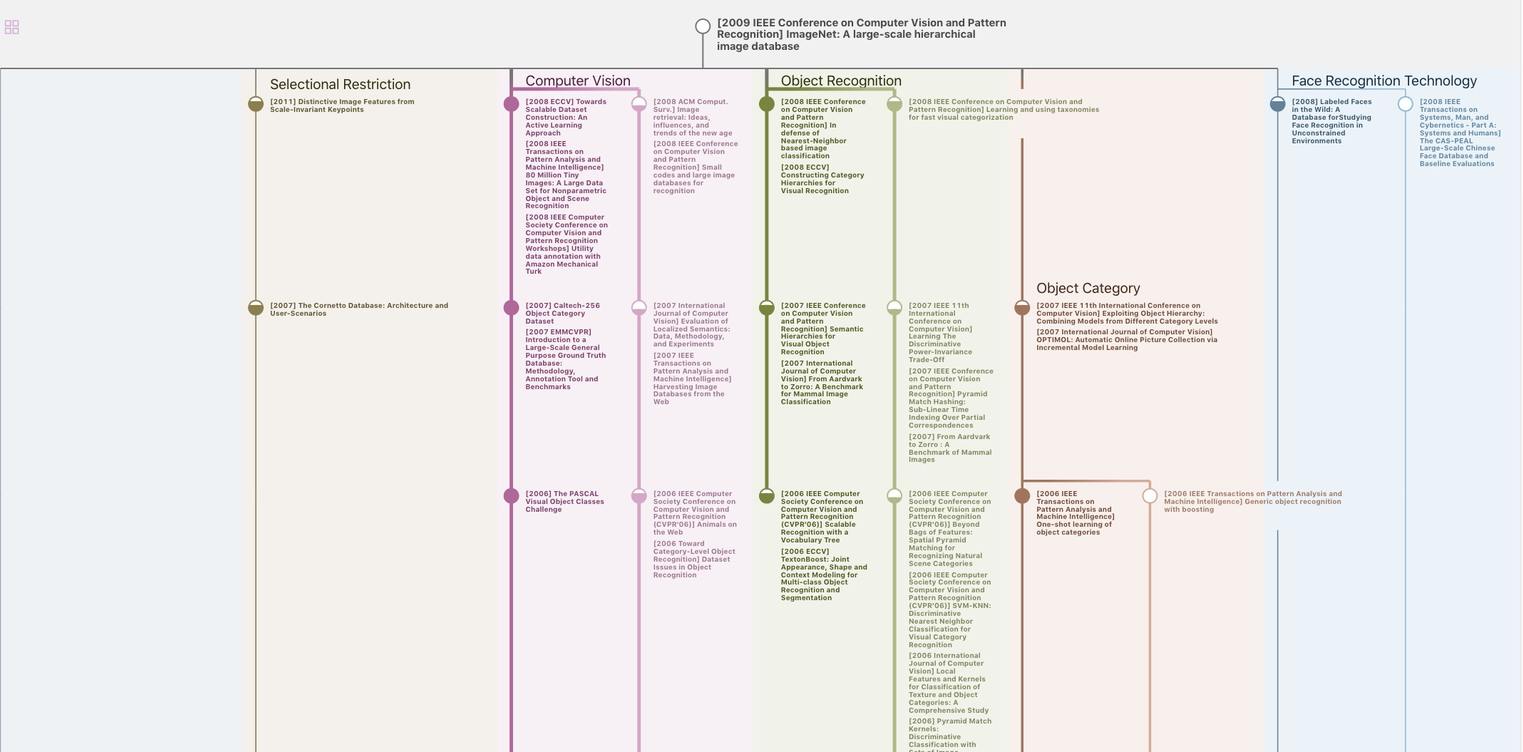
生成溯源树,研究论文发展脉络
Chat Paper
正在生成论文摘要