Remote Power Side-Channel Attacks on CNN Accelerators in FPGAs
arxiv(2020)
摘要
To lower cost and increase the utilization of Cloud FPGAs, researchers have recently been exploring the concept of multi-tenant FPGAs, where multiple independent users simultaneously share the same FPGA. Despite its benefits, multitenancy opens up the possibility of malicious users co-locating on the same FPGA as a victim user, and extracting sensitive information. This issue becomes especially serious when the user is running a machine learning algorithm that is processing sensitive or private information. To demonstrate the dangers, this paper presents the first remote, power-based side-channel attack on a deep neural network accelerator running in a variety of Xilinx FPGAs and also on Cloud FPGAs using Amazon Web Services (AWS) F1 instances. This work in particular shows how to remotely obtain voltage estimates as a deep neural network inference circuit executes, and how the information can be used to recover the inputs to the neural network. The attack is demonstrated with a binarized convolutional neural network used to recognize handwriting images from the MNIST handwritten digit database. With the use of precise time-to-digital converters for remote voltage estimation, the MNIST inputs can be successfully recovered with a maximum normalized cross-correlation of 84% between the input image and the recovered image on local FPGA boards and 77% on AWS F1 instances. The attack requires no physical access nor modifications to the FPGA hardware.
更多查看译文
关键词
cnn accelerators,side-channel
AI 理解论文
溯源树
样例
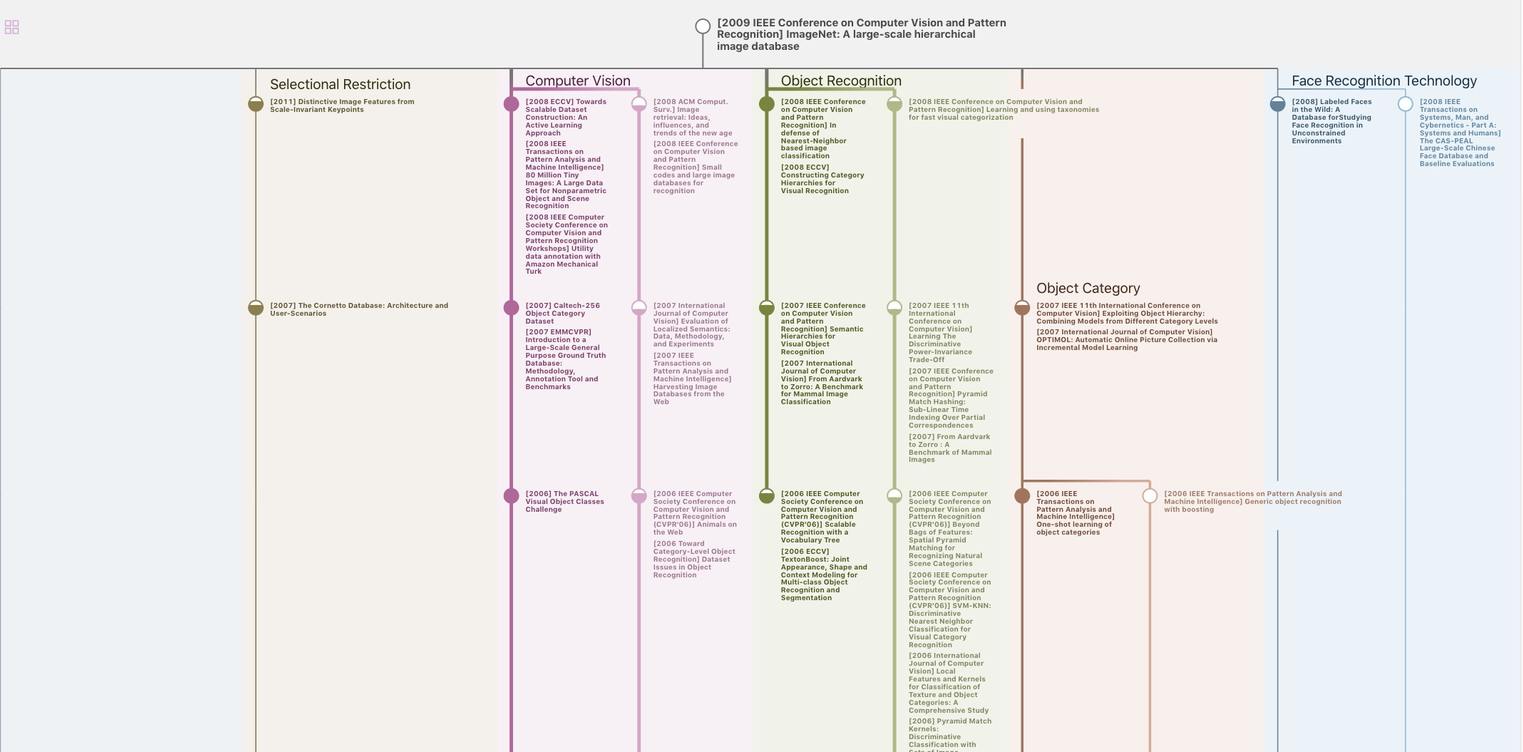
生成溯源树,研究论文发展脉络
Chat Paper
正在生成论文摘要