Ember: energy management of batteryless event detection sensors with deep reinforcement learning
SenSys '20: The 18th ACM Conference on Embedded Networked Sensor Systems Virtual Event Japan November, 2020(2020)
摘要
Energy management can extend the lifetime of batteryless, energy-harvesting systems by judiciously utilizing the energy available. Duty cycling of such systems is especially challenging for event detection, as events arrive sporadically and energy availability is uncertain. If the node sleeps too much, it may miss important events; if it depletes energy too quickly, it will stop operating in low energy conditions and miss events. Thus, accurate event prediction is important in making this tradeoff. We propose Ember, an energy management system based on deep reinforcement learning to duty cycle event-driven sensors in low energy conditions. We train a policy using historical real-world data traces of motion, temperature, humidity, pressure, and light events. The resulting policy can learn to capture up to 95% of the events without depleting the node. Without historical data for training when deploying a node at a new location, we propose a self-supervised mechanism to collect ground-truth data while learning from the data at the same time. Ember learns to capture the majority of events within a week without any historical data and matches the performance of the policies trained with historical data in a few weeks. We deployed 40 nodes running Ember for indoor sensing and demonstrate that the learned policies generalize to real-world settings as well as outperform state-of-the-art techniques.
更多查看译文
AI 理解论文
溯源树
样例
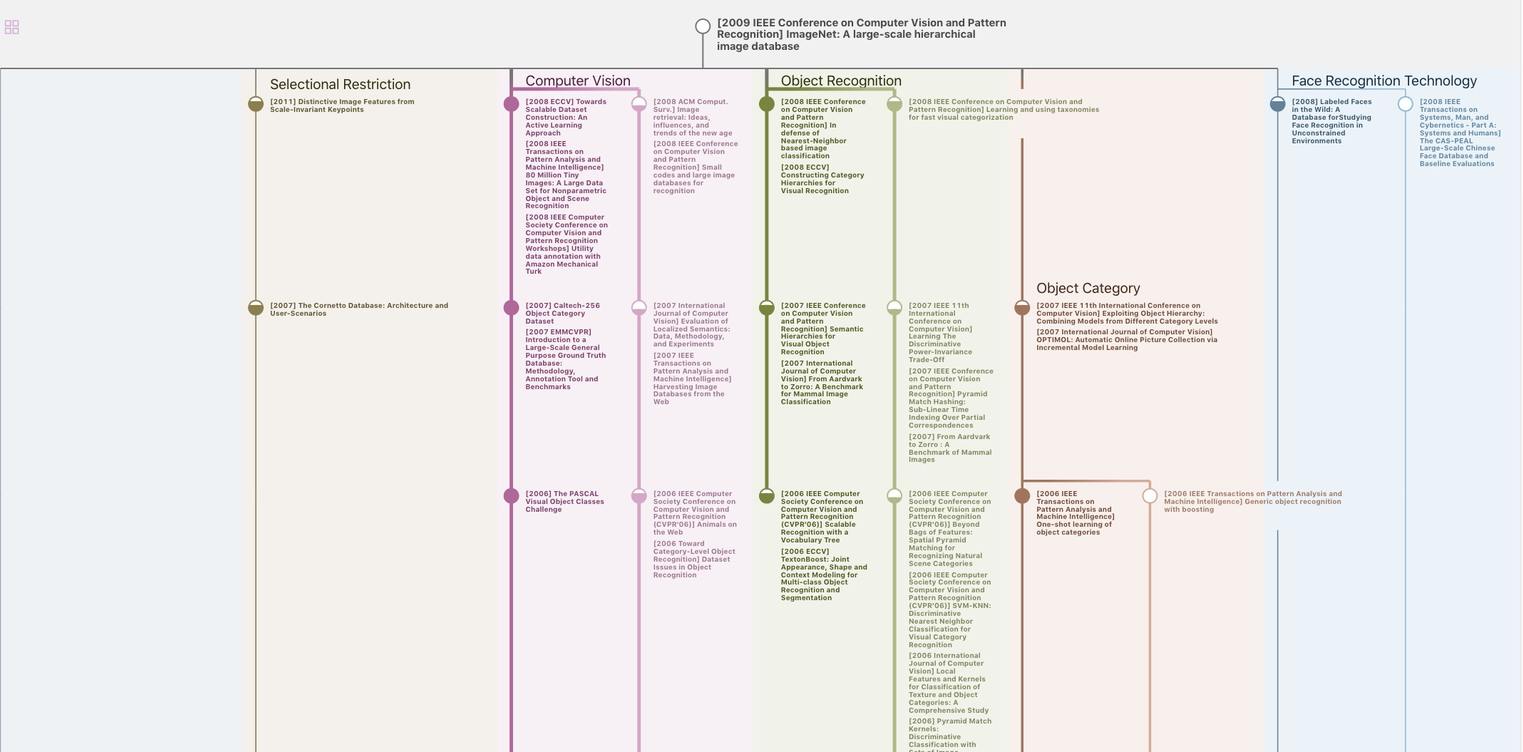
生成溯源树,研究论文发展脉络
Chat Paper
正在生成论文摘要