MobiPose: real-time multi-person pose estimation on mobile devices
SenSys '20: The 18th ACM Conference on Embedded Networked Sensor Systems Virtual Event Japan November, 2020(2020)
摘要
Human pose estimation is a key technique for many vision-based mobile applications. Yet existing multi-person pose-estimation methods fail to achieve a satisfactory user experience on commodity mobile devices such as smartphones, due to their long model-inference latency. In this paper, we propose MobiPose, a system designed to enable real-time multi-person pose estimation on mobile devices through three novel techniques. First, MobiPose takes a motion-vector-based approach to fast locate the human proposals across consecutive frames by fine-grained tracking of joints of human body, rather than running the expensive human-detection model for every frame. Second, MobiPose designs a mobile-friendly model that uses lightweight multi-stage feature extractions to significantly reduce the latency of pose estimation without compromising the model accuracy. Third, MobiPose leverages the heterogeneous computing resources of both CPU and GPU to execute the pose estimation model for multiple persons in parallel, which further reduces the total latency. We have implemented the MobiPose system on off-the-shelf commercial smartphones and conducted comprehensive experiments to evaluate the effectiveness of the proposed techniques. Evaluation results show that MobiPose achieves over 20 frames per second pose estimation with 3 persons per frame, and significantly outperforms the state-of-the-art baseline, with a speedup of up to 4.5X and 2.8X in latency on CPU and GPU, respectively, and an improvement of 5.1% in pose-estimation model accuracy. Furthermore, MobiPose achieves up to 62.5% and 37.9% energy-per-frame saving on average in comparison with the baseline on mobile CPU and GPU, respectively.
更多查看译文
AI 理解论文
溯源树
样例
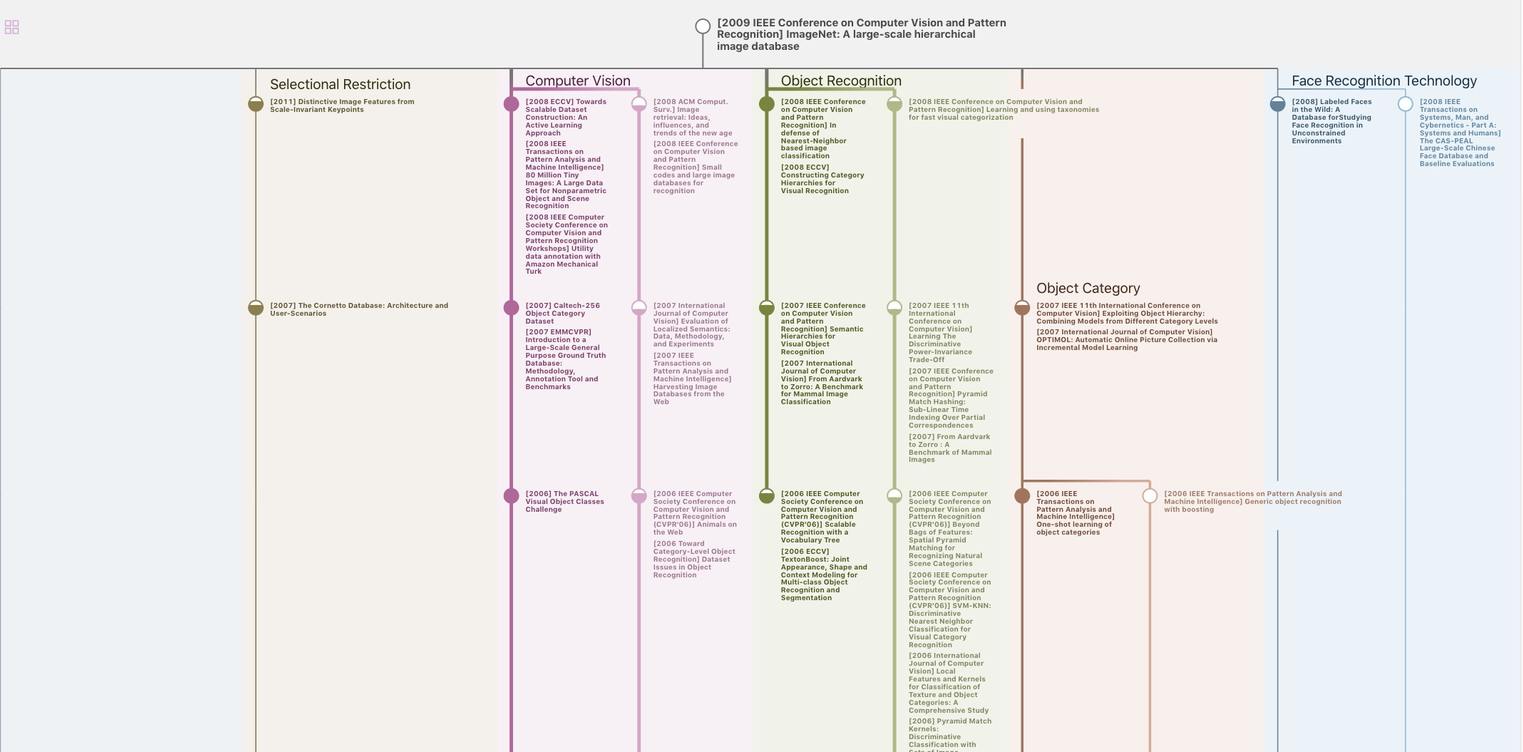
生成溯源树,研究论文发展脉络
Chat Paper
正在生成论文摘要