Neuroplex: learning to detect complex events in sensor networks through knowledge injection
SenSys '20: The 18th ACM Conference on Embedded Networked Sensor Systems Virtual Event Japan November, 2020(2020)
摘要
Despite the remarkable success in a broad set of sensing applications, state-of-the-art deep learning techniques struggle with complex reasoning tasks across a distributed set of sensors. Unlike recognizing transient complex activities (e.g., human activities such as walking or running) from a single sensor, detecting more complex events with larger spatial and temporal dependencies across multiple sensors is extremely difficult, e.g., utilizing a hospital's sensor network to detect whether a nurse is following a sanitary protocol as they traverse from patient to patient. Training a more complicated model requires a larger amount of data-which is unrealistic considering complex events rarely happen in nature. Moreover, neural networks struggle with reasoning about serial, aperiodic events separated by large quantities in the spatial-temporal dimensions.
We propose Neuroplex, a neural-symbolic framework that learns to perform complex reasoning on raw sensory data with the help of high-level, injected human knowledge. Neuroplex decomposes the entire complex learning space into explicit perception and reasoning layers, i.e., by maintaining neural networks to perform low-level perception tasks and neurally reconstructed reasoning models to perform high-level, explainable reasoning. After training the neurally reconstructed reasoning model using human knowledge, Neuroplex allows effective end-to-end training of perception models with an additional semantic loss using only sparse, high-level annotations. Our experiments and evaluation show that Neuroplex is capable of learning to efficiently and effectively detect complex events-which cannot be handled by state-of-the-art neural network models. During the training, Neuroplex not only reduces data annotation requirements by 100x, but also significantly speeds up the learning process for complex event detection by 4x.
更多查看译文
AI 理解论文
溯源树
样例
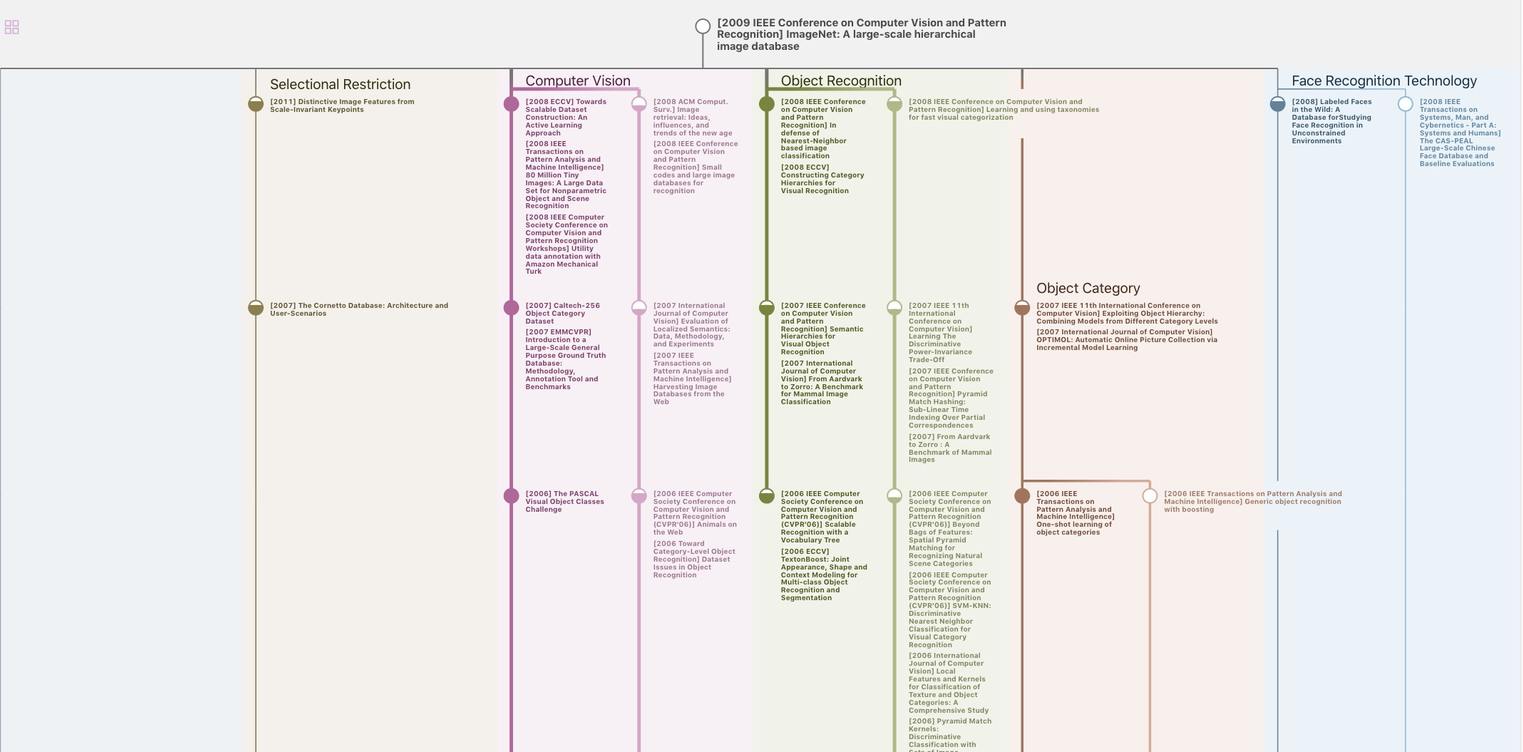
生成溯源树,研究论文发展脉络
Chat Paper
正在生成论文摘要