Determinantal Point Processes Implicitly Regularize Semiparametric Regression Problems
arxiv(2022)
摘要
Semiparametric regression models are used in several applications which require comprehensibility without sacrificing accuracy. Typical examples are spline interpolation in geophysics and nonlinear time series problems, where the system includes a linear and nonlinear component. We discuss here the use of a finite determinantal point process (DPP) for approximating semiparametric models. Recently, Barthelme ', Tremblay, Usevich, and Amblard introduced a novel representation of finite DPPs. These authors formulated extended L-ensembles that can conveniently represent partial -projection DPPs and suggest their use for optimal interpolation. With the help of this formalism, we derive a key identity illustrating the implicit regularization effect of determinantal sampling for semiparametric regression and interpolation. Also, a novel projected Nystro center dot m approximation is defined and used to derive a bound on the expected in-sample prediction error for the corresponding approximation of semiparametric regression. This work naturally extends similar results obtained for kernel ridge regression.
更多查看译文
关键词
determinantal point processes,regression
AI 理解论文
溯源树
样例
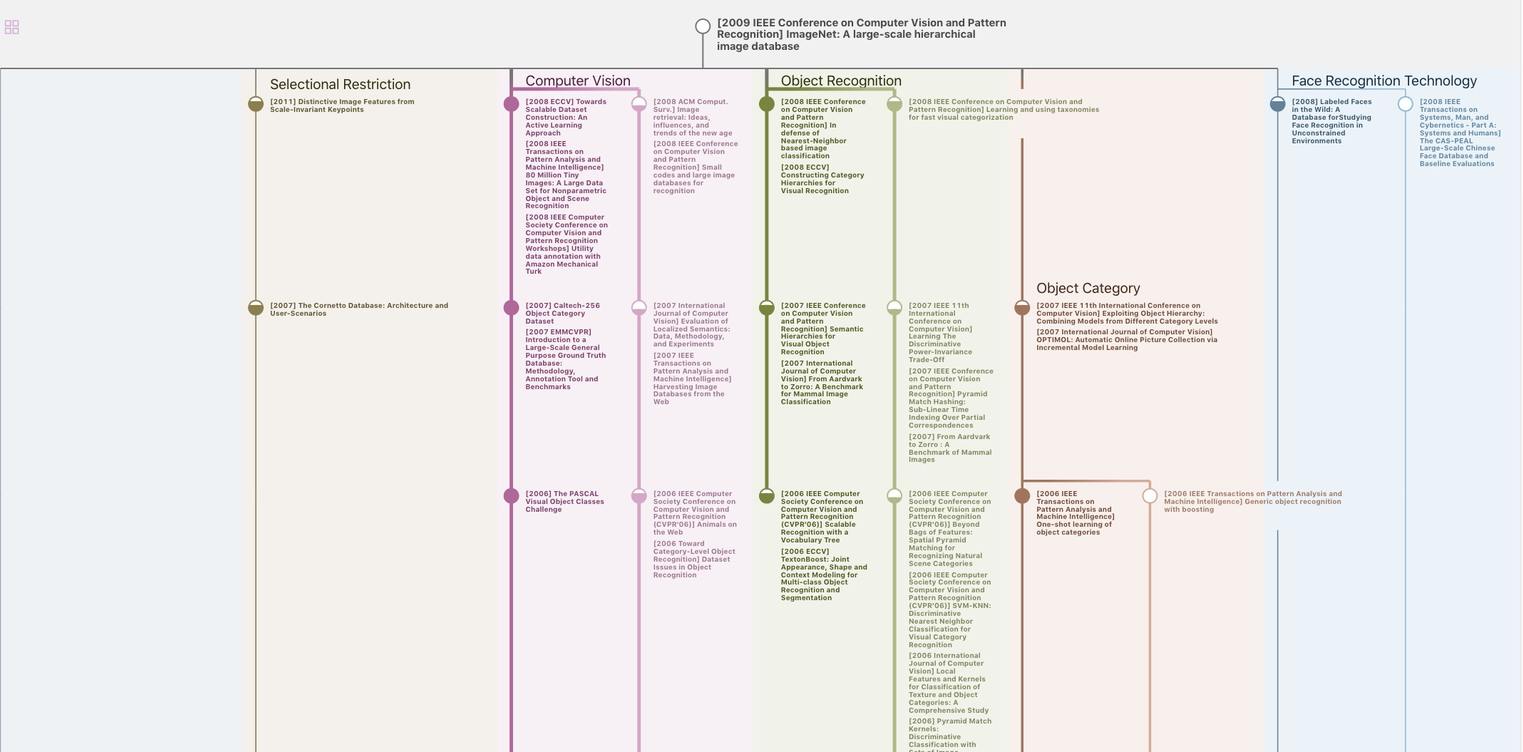
生成溯源树,研究论文发展脉络
Chat Paper
正在生成论文摘要