Digital methods to enhance the usefulness of patient experience data in services for long-term conditions: the DEPEND mixed-methods study
Health Services and Delivery Research(2020)
摘要
Background Collecting NHS patient experience data is critical to ensure the delivery of high-quality services. Data are obtained from multiple sources, including service-specific surveys and widely used generic surveys. There are concerns about the timeliness of feedback, that some groups of patients and carers do not give feedback and that free-text feedback may be useful but is difficult to analyse. Objective To understand how to improve the collection and usefulness of patient experience data in services for people with long-term conditions using digital data capture and improved analysis of comments. Design The DEPEND study is a mixed-methods study with four parts: qualitative research to explore the perspectives of patients, carers and staff; use of computer science text-analytics methods to analyse comments; co-design of new tools to improve data collection and usefulness; and implementation and process evaluation to assess use of the tools and any impacts. Setting Services for people with severe mental illness and musculoskeletal conditions at four sites as exemplars to reflect both mental health and physical long-terms conditions: an acute trust (site A), a mental health trust (site B) and two general practices (sites C1 and C2). Participants A total of 100 staff members with diverse roles in patient experience management, clinical practice and information technology; 59 patients and 21 carers participated in the qualitative research components. Interventions The tools comprised a digital survey completed using a tablet device (kiosk) or a pen and paper/online version; guidance and information for patients, carers and staff; text-mining programs; reporting templates; and a process for eliciting and recording verbal feedback in community mental health services. Results We found a lack of understanding and experience of the process of giving feedback. People wanted more meaningful and informal feedback to suit local contexts. Text mining enabled systematic analysis, although challenges remained, and qualitative analysis provided additional insights. All sites managed to collect feedback digitally; however, there was a perceived need for additional resources, and engagement varied. Observation indicated that patients were apprehensive about using kiosks but often would participate with support. The process for collecting and recording verbal feedback in mental health services made sense to participants, but was not successfully adopted, with staff workload and technical problems often highlighted as barriers. Staff thought that new methods were insightful, but observation did not reveal changes in services during the testing period. Conclusions The use of digital methods can produce some improvements in the collection and usefulness of feedback. Context and flexibility are important, and digital methods need to be complemented with alternative methods. Text mining can provide useful analysis for reporting on large data sets within large organisations, but qualitative analysis may be more useful for small data sets and in small organisations. Limitations New practices need time and support to be adopted and this study had limited resources and a limited testing time. Future work Further research is needed to improve text-analysis methods for routine use in services and to evaluate the impact of methods (digital and non-digital) on service improvement in varied contexts and among diverse patients and carers. Funding This project was funded by the NIHR Health Services and Delivery Research programme and will be published in full in Health Services and Delivery Research; Vol. 8, No. 28. See the NIHR Journals Library website for further project information.
更多查看译文
关键词
patient experience,patient experience data,patient feedback,digital health,digital participation,text mining,co-design,quality improvement,mental health services,rheumatology,mixed methods
AI 理解论文
溯源树
样例
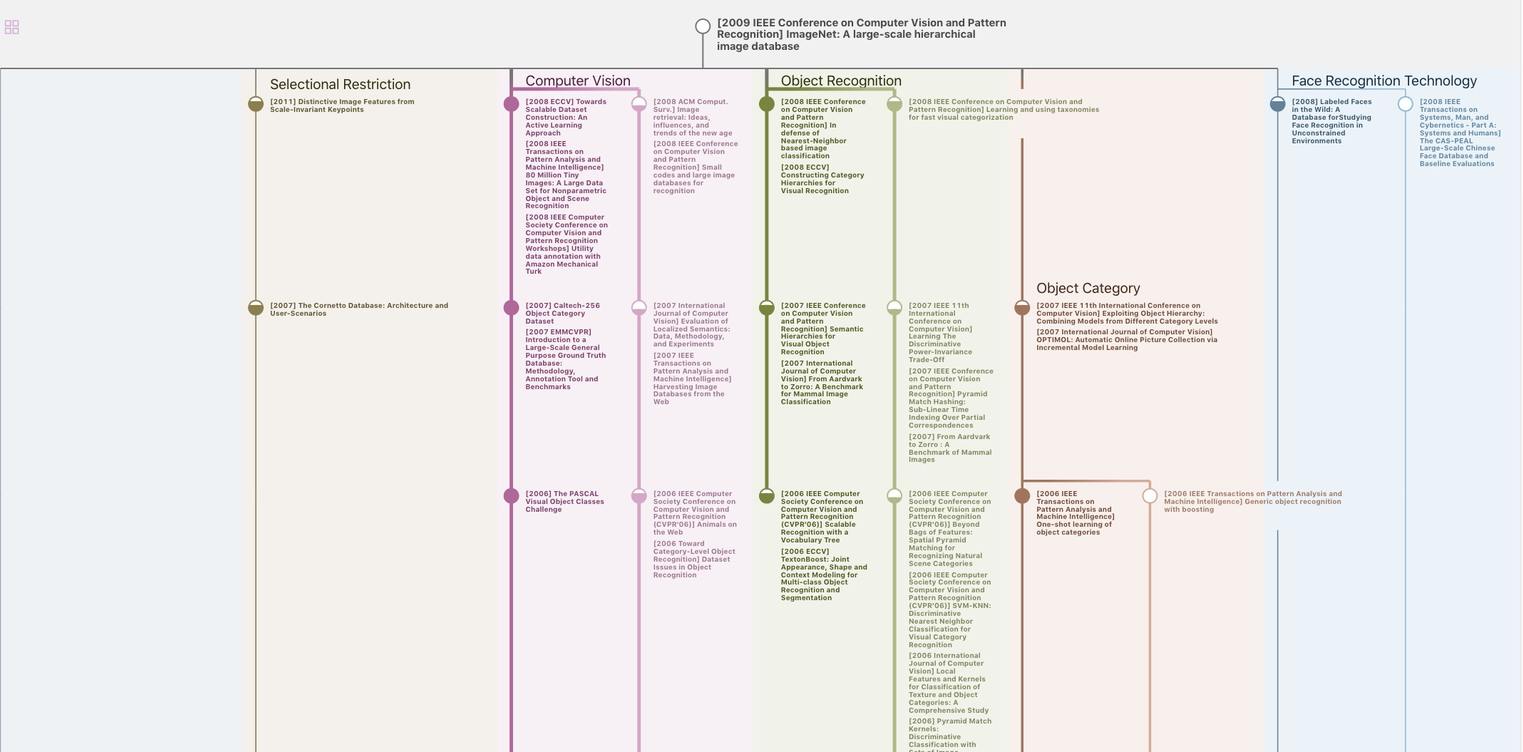
生成溯源树,研究论文发展脉络
Chat Paper
正在生成论文摘要