Classification with segmentation for credit scoring and bankruptcy prediction
EMPIRICAL ECONOMICS(2020)
摘要
Segmentation or clustering is a key concept in the economy. It can be defined as a grouping of interconnected firms or individuals in a particular domain. It is a powerful tool that may be used to form clusters in order to increase business productivity and efficiency. The aim of this paper is to investigate the concept of clusters in the development of credit scoring models. We propose a hybrid method based on clustering and random forest techniques. The purpose is to design an effective credit scoring model and enhance financial bankruptcy prediction. We use clustering to partition data into relatively homogeneous groups of credit applicants where the aim is to cluster creditworthy applicants against non-creditworthy ones. We consider k-means algorithm in the clustering step to segment similar applicants into groups. Then, we apply random forest learning technique on the clustered data. Empirical studies are conducted on six well-known financial datasets of different sizes. The numerical results are promising and show the effectiveness of the proposed technique for applicants segmentation. When clustering is used with classification, the resulting method succeeds in improving highly the classification performance.
更多查看译文
关键词
Credit scoring, Clustering, Segmentation, Classification, Banking, Finance
AI 理解论文
溯源树
样例
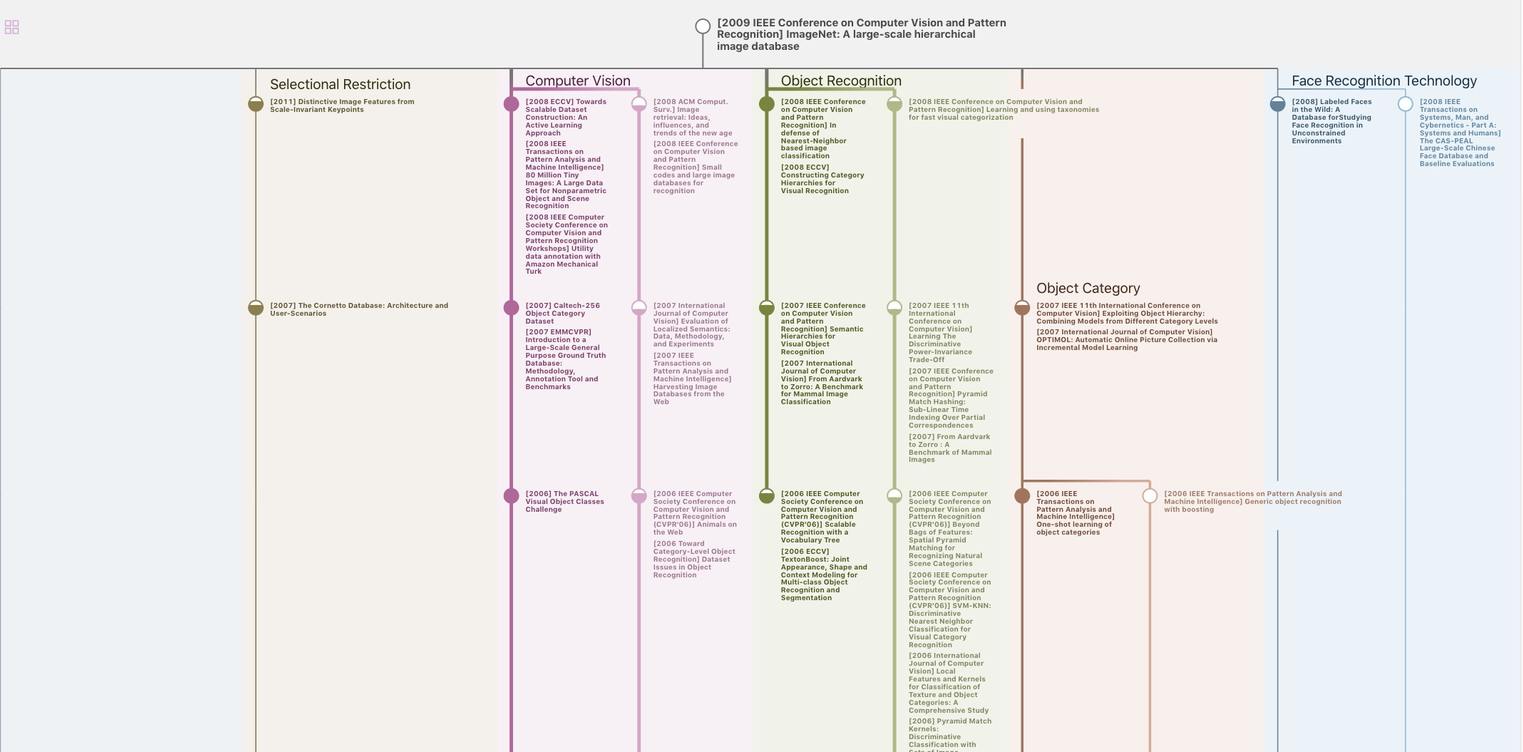
生成溯源树,研究论文发展脉络
Chat Paper
正在生成论文摘要