Quantile regression for massive data with network-induced dependence, and application to the New York statewide planning and research cooperative system
COMMUNICATIONS IN STATISTICS-THEORY AND METHODS(2022)
摘要
Medical costs are often skewed to the right, heteroscedastic, and having a sophisticated relation with covariates. Moreover, medical cost datasets are always massive, such as in the New York Statewide Planning and Research Cooperative System Expenditure Study. Different observations can depend on each other as the spatial distribution of diseases induces complex correlation among patients coming from nearby communities. Therefore, it is not enough if only focus on the mean function regression models with low-dimensional covariates, small sample size and identically independent observations. In this paper, we propose a new quantile regression model to analyze medical costs. A network term is introduced to account for the dependence among different observations. We also consider variable selection for massive datasets. An adaptive lasso penalized variable selection method is applied in a parallel manner, the resulting estimators are combined through minimizing an extra penalized loss function. Simulation studies are conducted to illustrate the performance of the estimation method. We apply our method to the analysis of the New York State's Statewide Planning and Research Cooperative System, 2013.
更多查看译文
关键词
High-dimensional covariates, massive data, network, partially non-linear, non-independent observation, quantile regression, single-index, variable selection
AI 理解论文
溯源树
样例
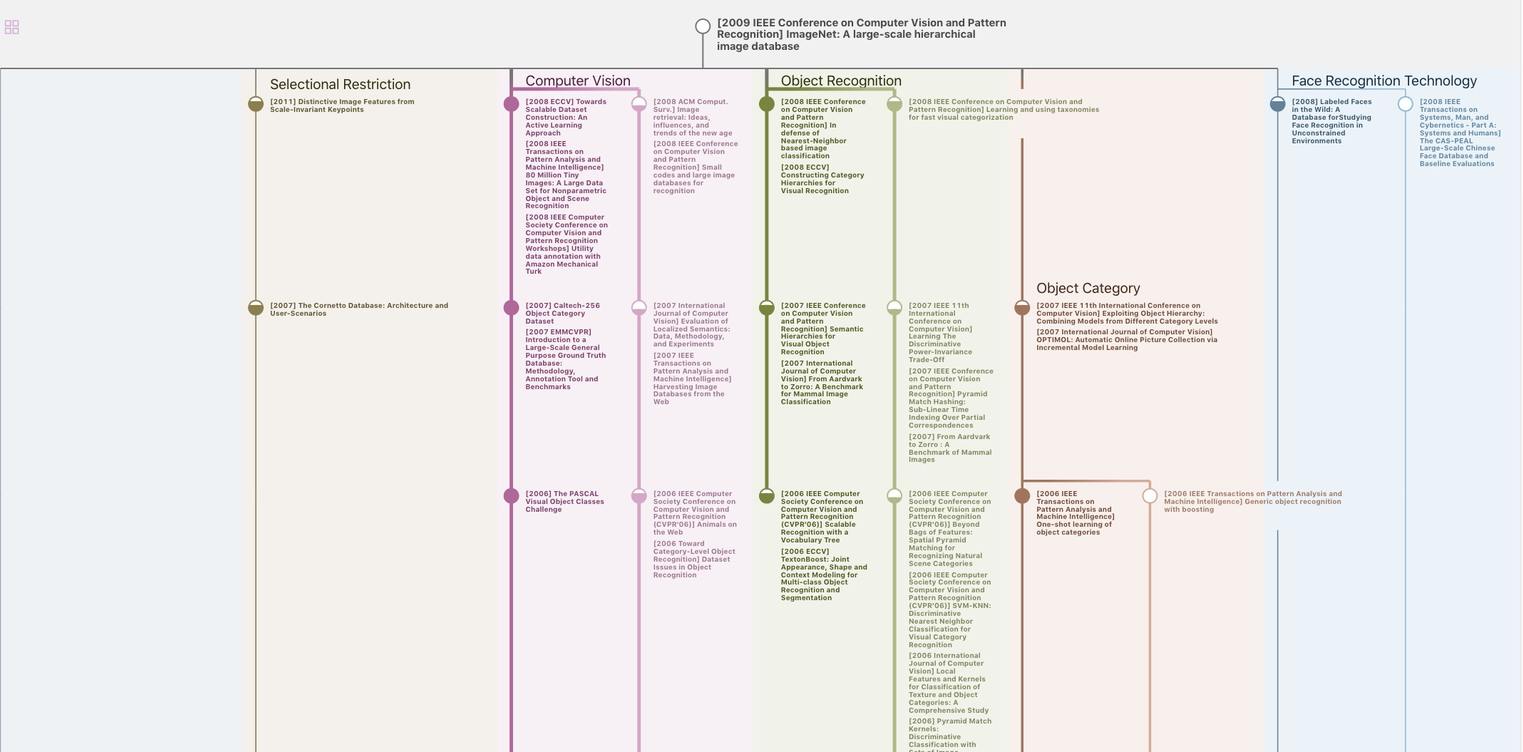
生成溯源树,研究论文发展脉络
Chat Paper
正在生成论文摘要