Indexing and progressive top- k similarity retrieval of trajectories
WORLD WIDE WEB-INTERNET AND WEB INFORMATION SYSTEMS(2020)
摘要
In this work, we study the performance of state-of-the-art access methods to efficiently store and retrieve trajectories in spatial networks. First, we study how efficiently such methods can manage trajectory data to support indexing for data demanding applications where trajectory retrieval must be fast. At the same time, trajectory insertions, deletions and modifications should also be executed efficiently. Secondly, we compare the performance of progressive processing of trajectory similarity top- k queries, which is a common query in spatial applications. Specifically, we examine FNR-trees (Frentzos 2003 ) and MON-trees (de Almeida and Gueting, 2005 ), which have been proposed for trajectory management, against a novel variation of our proposed Cluster-extended Adjacency Lists (CeAL) (Tiakas and Rafailidis 2015 ). In particular: (a) we extend the above access methods to efficiently handle trajectories of objects that move in large spatial networks, and (b) to enhance their performance, we create an entirely new implementation framework to generate trajectories and to test the trajectory management and retrieval for each approach. With respect to the generation of trajectories, we extend the generator by Brinkhoff ( 2000 ) to efficiently support very large spatial networks. Finally, we conduct extensive experimentation which demonstrates that the proposed method CeAL prevails in space and time complexity.
更多查看译文
关键词
Location-based services, Trajectory, indexing, Spatio-temporal queries, Progressive processing, Top-k queries, Similarity retrieval
AI 理解论文
溯源树
样例
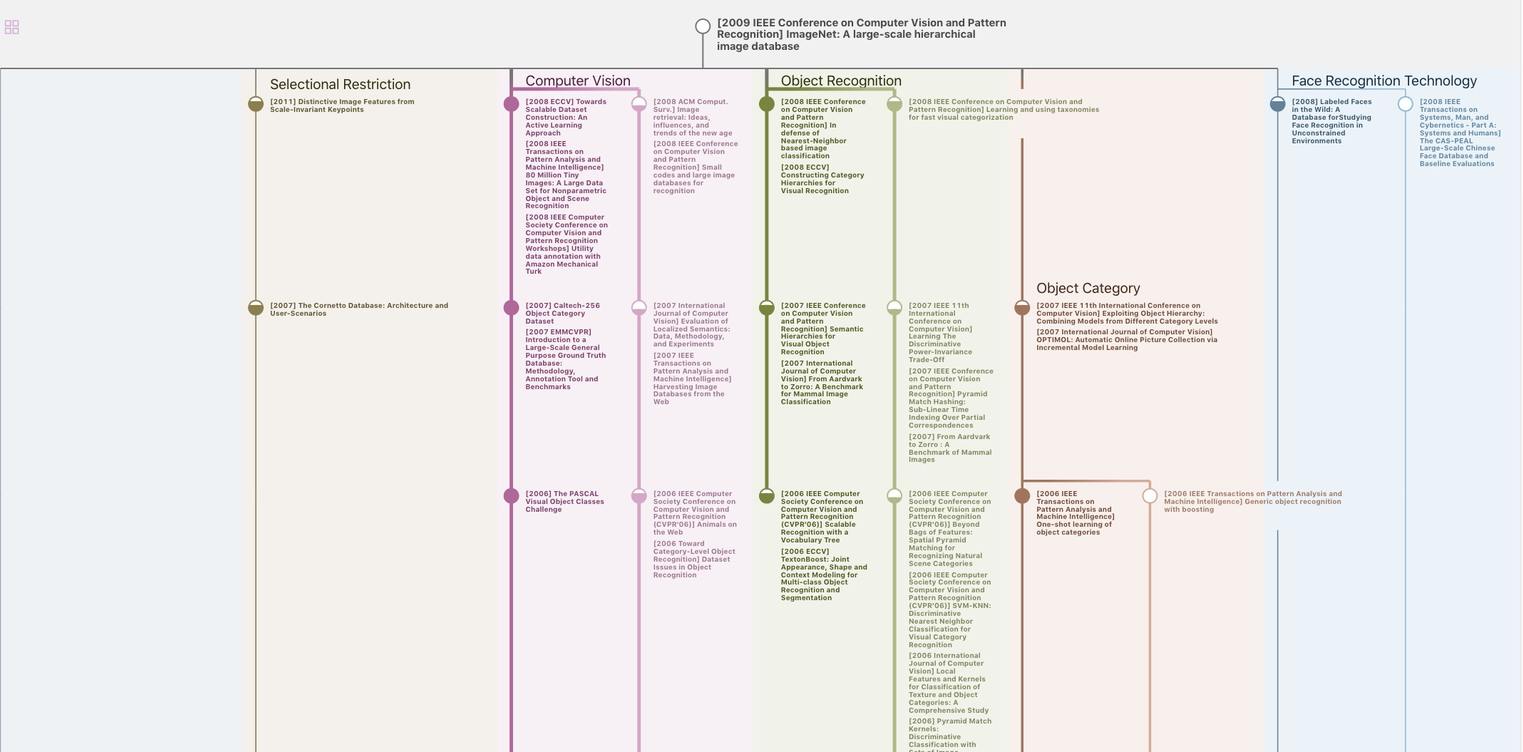
生成溯源树,研究论文发展脉络
Chat Paper
正在生成论文摘要