Tag-informed collaborative topic modeling for cross domain recommendations
Knowledge-Based Systems(2020)
摘要
Collaborative topic modeling is powerful to alleviate data sparsity in recommender systems owing to the incorporation of collaborative filtering and topic models. However sufficient textual data is not always available. On the other hand, tags serving as supplementary description of items can reflect users’ interests in item attributes. But previous works only mine the effect of tags on ratings in one domain and ignore that in related domains items can be related in attributes. Tags encode similar properties of items and can be transferred across domains to mutually benefit recommendations for both domains. In this study we propose a TagCDCTR (Tag-informed Cross Domain Collaborative Topic Regression) model, which exploits shared tags as bridges to link related domains through an extended collaborative topic modeling framework. The model exploits the inter-domain relations by encoding cross domain item–item similarity based on common tags and jointly learning a shared set of topics from all domains together. Collectively factorizing the rating matrices of multiple domains into common user latent factors and domain-specific item latent factors, so that the learned item latent factors are linked through the inter-domain relations, helping to capture the items more comprehensively. The rich information reused in multiple domains alleviates data sparsity and the semantic advantage of topics and tags provides a better interpretability of recommendations. The experiments conducted on three datasets demonstrate that TagCDCTR outperforms state-of-the-art collaborative-topic-based models and cross-domain-based models.
更多查看译文
关键词
Collaborative filtering,Recommender system,Cross domain,Topic model
AI 理解论文
溯源树
样例
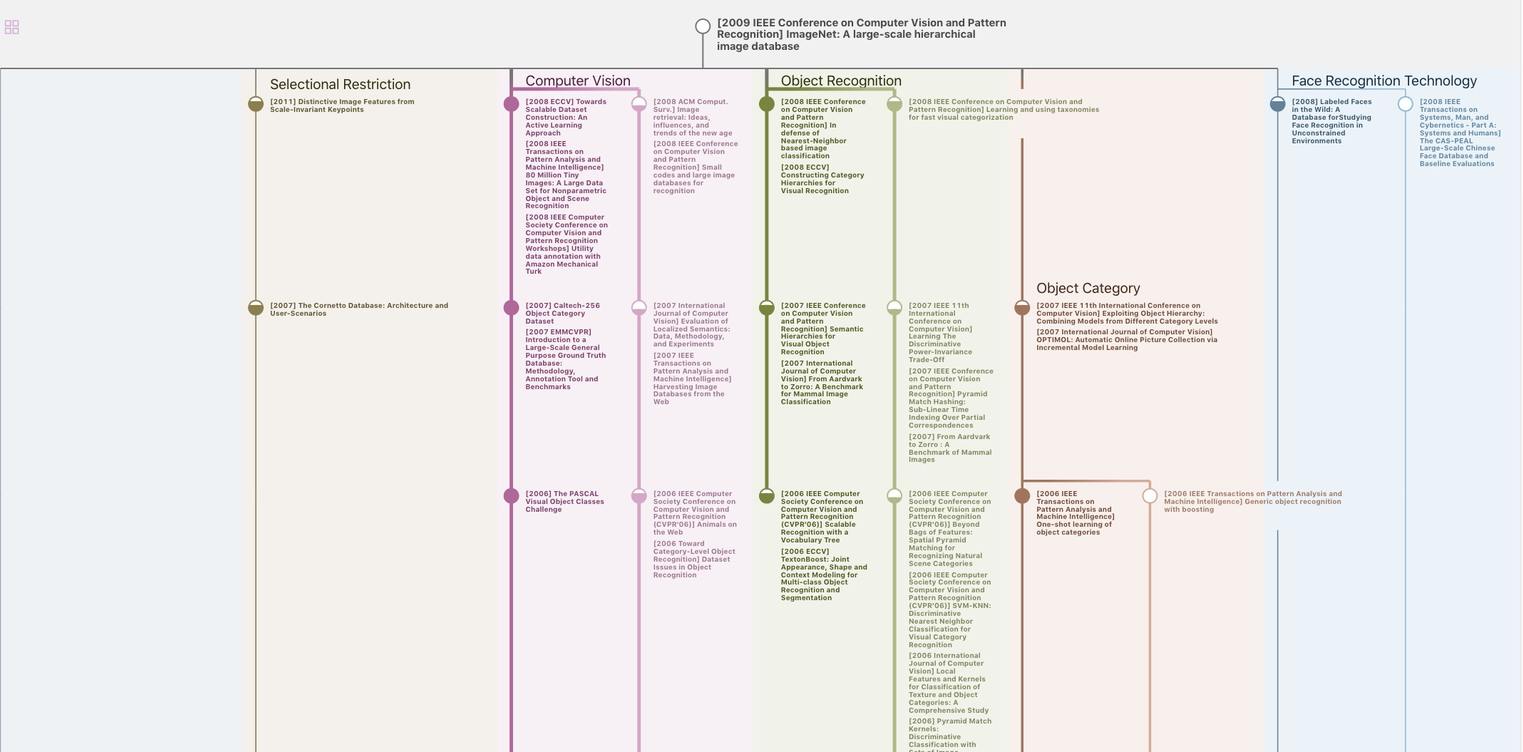
生成溯源树,研究论文发展脉络
Chat Paper
正在生成论文摘要