Prediction of Chlorophyll-a Concentrations in the Nakdong River Using Machine Learning Methods
Water(2020)
摘要
Many studies have attempted to predict chlorophyll-a concentrations using multiple regression models and validating them with a hold-out technique. In this study commonly used machine learning models, such as Support Vector Regression, Bagging, Random Forest, Extreme Gradient Boosting (XGBoost), Recurrent Neural Network (RNN), and Long–Short-Term Memory (LSTM), are used to build a new model to predict chlorophyll-a concentrations in the Nakdong River, Korea. We employed 1–step ahead recursive prediction to reflect the characteristics of the time series data. In order to increase the prediction accuracy, the model construction was based on forward variable selection. The fitted models were validated by means of cumulative learning and rolling window learning, as opposed to the hold–out technique. The best results were obtained when the chlorophyll-a concentration was predicted by combining the RNN model with the rolling window learning method. The results suggest that the selection of explanatory variables and 1–step ahead recursive prediction in the machine learning model are important processes for improving its prediction performance.
更多查看译文
关键词
machine learning,recurrent neural network,long-short-term memory,1-step ahead recursive prediction,variable selection,water quality,chlorophyll-a
AI 理解论文
溯源树
样例
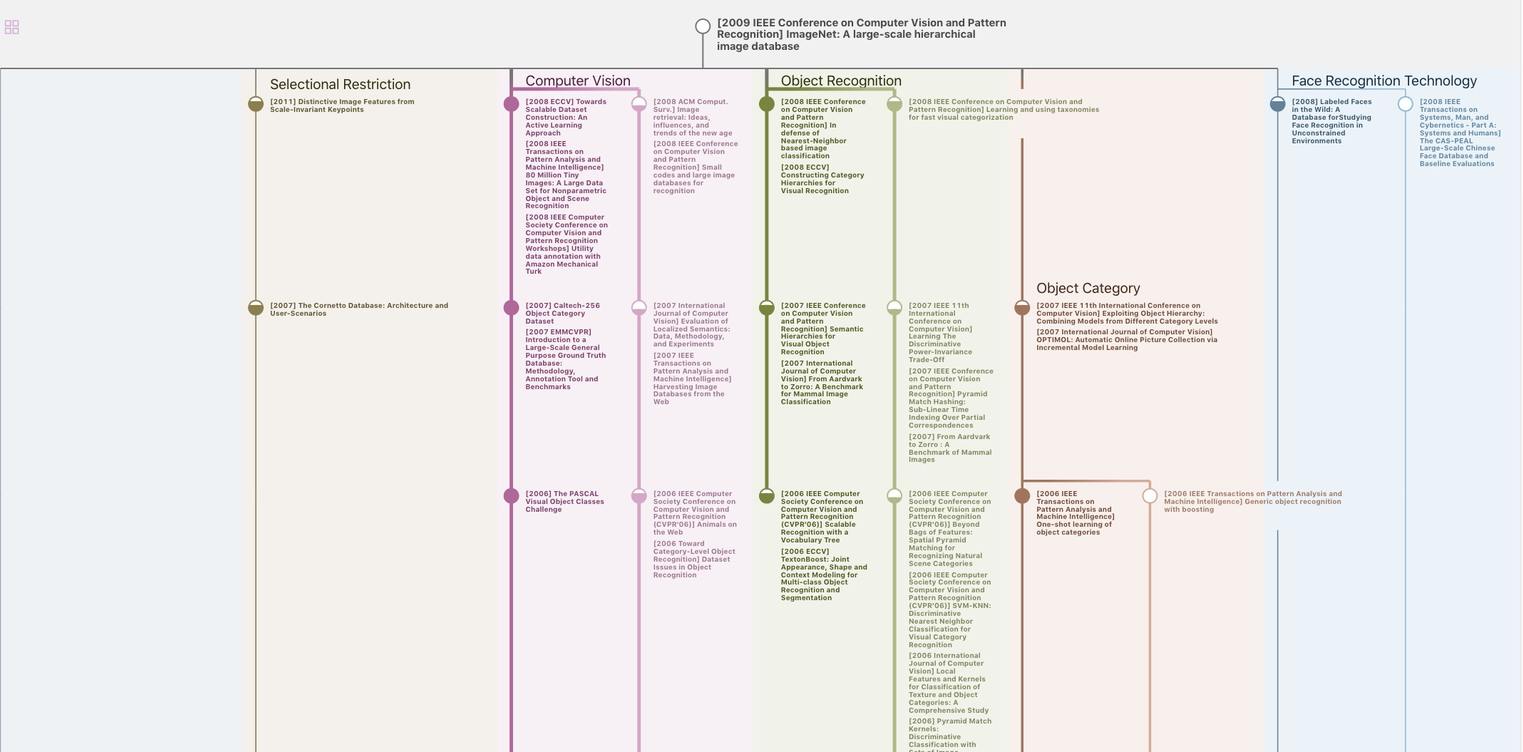
生成溯源树,研究论文发展脉络
Chat Paper
正在生成论文摘要