An Efficient Model-Free Approach for Controlling Large-Scale Canals via Hierarchical Reinforcement Learning
IEEE Transactions on Industrial Informatics(2021)
摘要
Large-scale canals with cascaded pools are constructed wordwide to divert water from rich to arid areas to mitigate water shortages. Efficient control of canals is essential to improve water-diversion performance. Numerous model-based approaches have been proposed and made great progress for canal control. However, when the predictive model is unavailable or unpromising for long time step predictions, model-free approaches could be considered as a possible way to achieve efficient control. Since most existing model-free approaches are focused on control of small canals or reservoirs, this article proposes a new control approach named policy and action reinforcement learning (PARL) for large-scale canals. We leverage the idea of “divide and conquer” to decompose the control task of large-scale canals into policy learning and action learning subtasks, and develop PARL by means of hierarchical reinforcement learning. Extensive experiments are conducted via numerical simulation on the case study of Chinese South to North Water Transfer Project, and experimental results show that PARL can achieve desirable performance improvements over other model-free learning approaches.
更多查看译文
关键词
Canal control,hierarchical reinforcement learning (HRL),model-free,multipool,south to north water transfer project
AI 理解论文
溯源树
样例
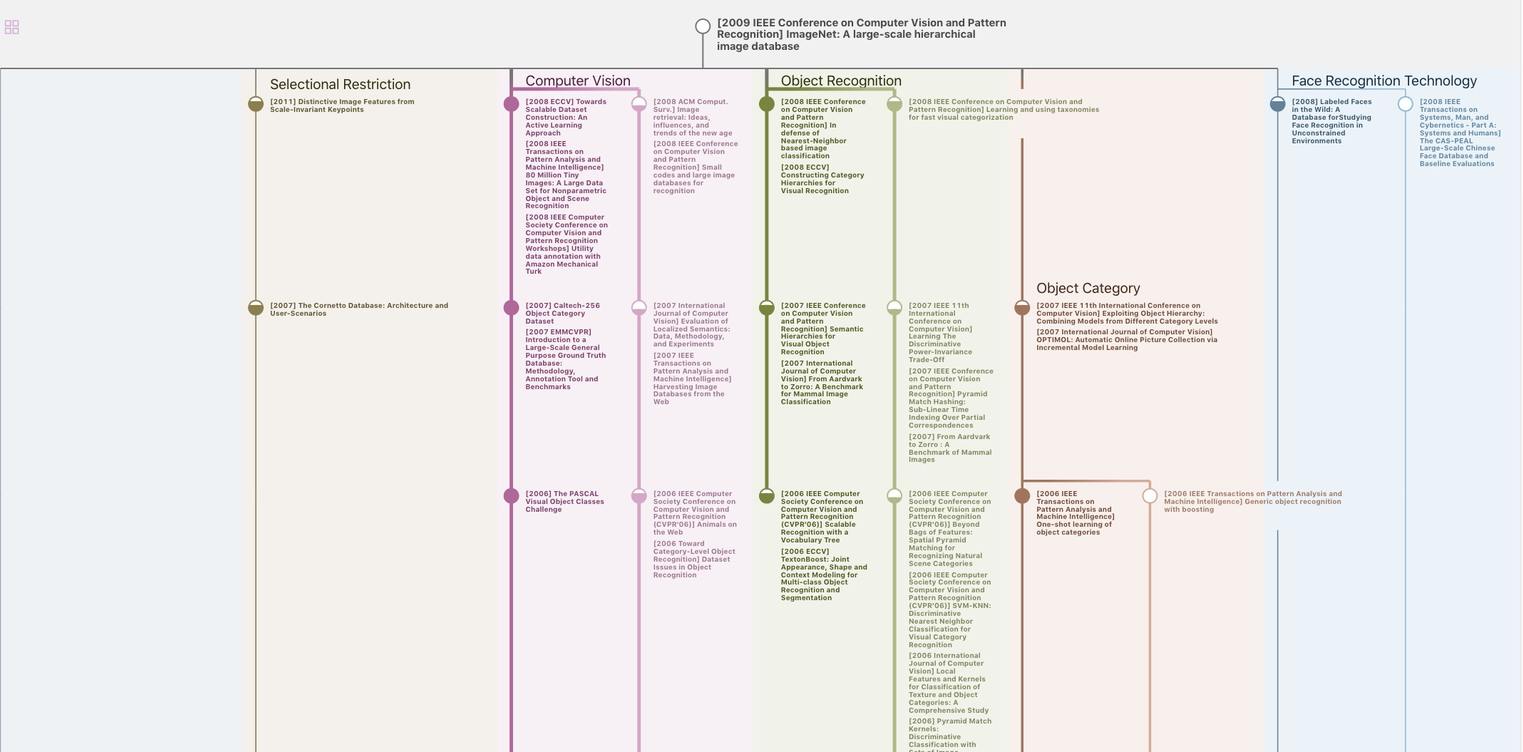
生成溯源树,研究论文发展脉络
Chat Paper
正在生成论文摘要