Refinement And Universal Approximation Via Sparsely Connected Relu Convolution Nets
IEEE SIGNAL PROCESSING LETTERS(2020)
摘要
We construct a highly regular and simple structured class of sparsely connected convolutional neural networks with rectifier activations that provide universal function approximation in a coarse-to-fine manner with increasing number of layers. The networks are localized in the sense that local changes in the function to be approximated only require local changes in the final layer of weights. At the core of the construction lies the fact that the characteristic function can be derived from a convolution of characteristic functions at the next coarser resolution via a rectifier passing. The latter refinement result holds for all higher order univariate B-splines.
更多查看译文
关键词
Function approximation, neural networks, splines
AI 理解论文
溯源树
样例
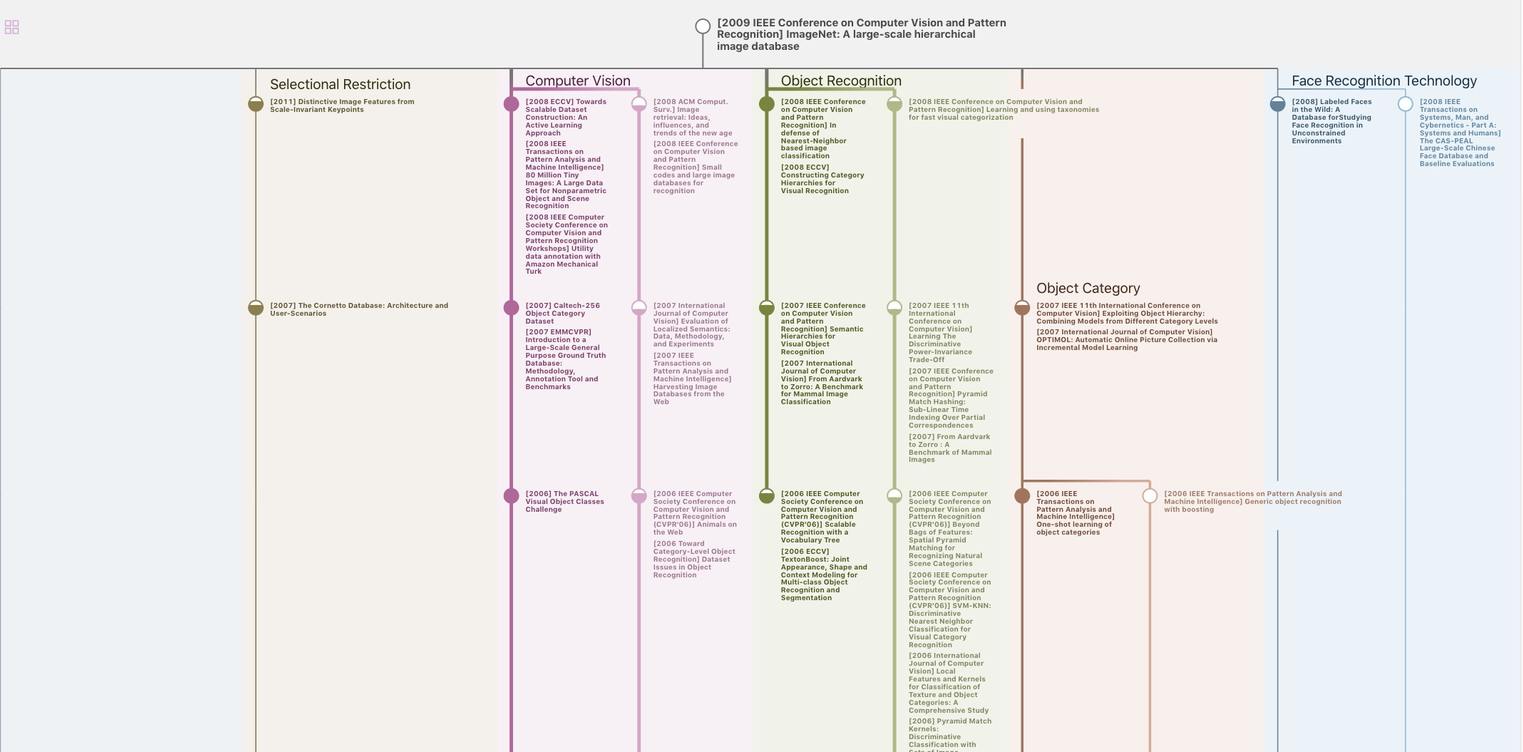
生成溯源树,研究论文发展脉络
Chat Paper
正在生成论文摘要