IoT-based monitoring and data-driven modelling of drip irrigation system for mustard leaf cultivation experiment
Information Processing in Agriculture(2021)
摘要
The changing dynamics, non-linearity of soil moisture content, as well as other weather and plant variables requires real-time monitoring and accurate predictive model for effective irrigation and crop management. In this paper, an improved monitoring and data-driven modelling of the dynamics of parameters affecting the irrigation of mustard leaf plant is presented. An IoT-based monitoring framework is implemented using ESPresso Lite V2.0 module interfaced with different soil moisture sensors (VH-400), flowmeter (YF-S201) as well as Davis vantage pro 2 weather station to measure soil moisture content, irrigation volume, and computation of the reference evapotranspiration (ETo). The data collected including plant images were transmitted to the Raspberry Pi 3 controller for onward online storage and the data are displayed on the IoT dashboard. The combination of both soil moisture and ETo values was used for scheduling a drip irrigated plant grown in a greenhouse for 35 days. A total number of 20, 703 experimental data samples are collected from the IoT-based platform was further used for data driven modelling through system identification in MATLAB. The result shows the development of different predictive models for soil moisture content prediction. The ARX prediction model is found to perform better than the ARMX, BJ and State space model in terms of estimated fit of 91.31%, 91.09%, 91.08%, and 90.75% respectively. Therefore, a robust monitoring framework for irrigation system has been developed, while the performance of the identified ARX model is promising to predict the volumetric soil water content.
更多查看译文
关键词
Internet of Things,Precision Irrigation,System Identification,Predictive Model,Monitoring,Control,MATLAB
AI 理解论文
溯源树
样例
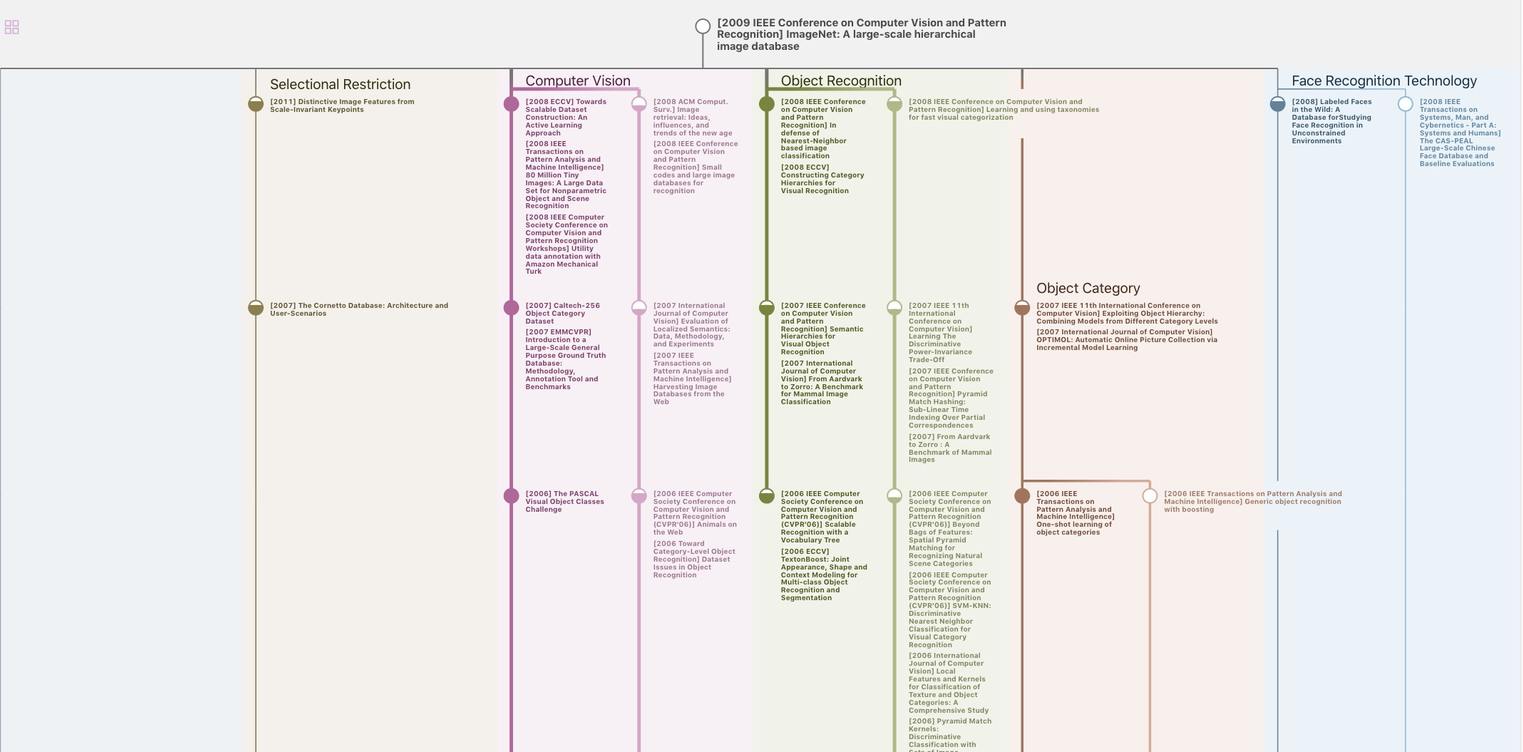
生成溯源树,研究论文发展脉络
Chat Paper
正在生成论文摘要