Multi-label Classification for Clinical Text with Feature-level Attention
2020 IEEE 6th Intl Conference on Big Data Security on Cloud (BigDataSecurity), IEEE Intl Conference on High Performance and Smart Computing, (HPSC) and IEEE Intl Conference on Intelligent Data and Security (IDS)(2020)
摘要
Multi-label text classification, which tags a given plain text with the most relevant labels from a label space, is an important task in the natural language process. To diagnose diseases, clinical researchers use a machine-learning algorithm to do multi-label clinical text classification. However, conventional machine learning methods can neither capture deep semantic information nor the context of words strictly. Diagnostic information from the EHRs (Electronic Health Records) is mainly constructed by unstructured clinical free text which is an obstacle for clinical feature extraction. Moreover, feature engineering is time-consuming and labor-intensive. With the rapid development of deep learning, we apply neural network models to resolve this problem mentioned above. To favor multi-label classification on EHRs, we propose FAMLC-BERT (Feature-level Attention for Multi-label classification on BERT) to capture semantic features from different layers. The model uses feature-level attention with BERT to recognize the labels of EHRs. We empirically compared our model with other state-of-the-art models on real-world documents collected from the hospital. Experiments show that our model achieved significant improvements compared to other selected benchmarks.
更多查看译文
关键词
Clinical Text,Multi-label Text Classification,Predicting Diagnosis,Attention,Deep Learning
AI 理解论文
溯源树
样例
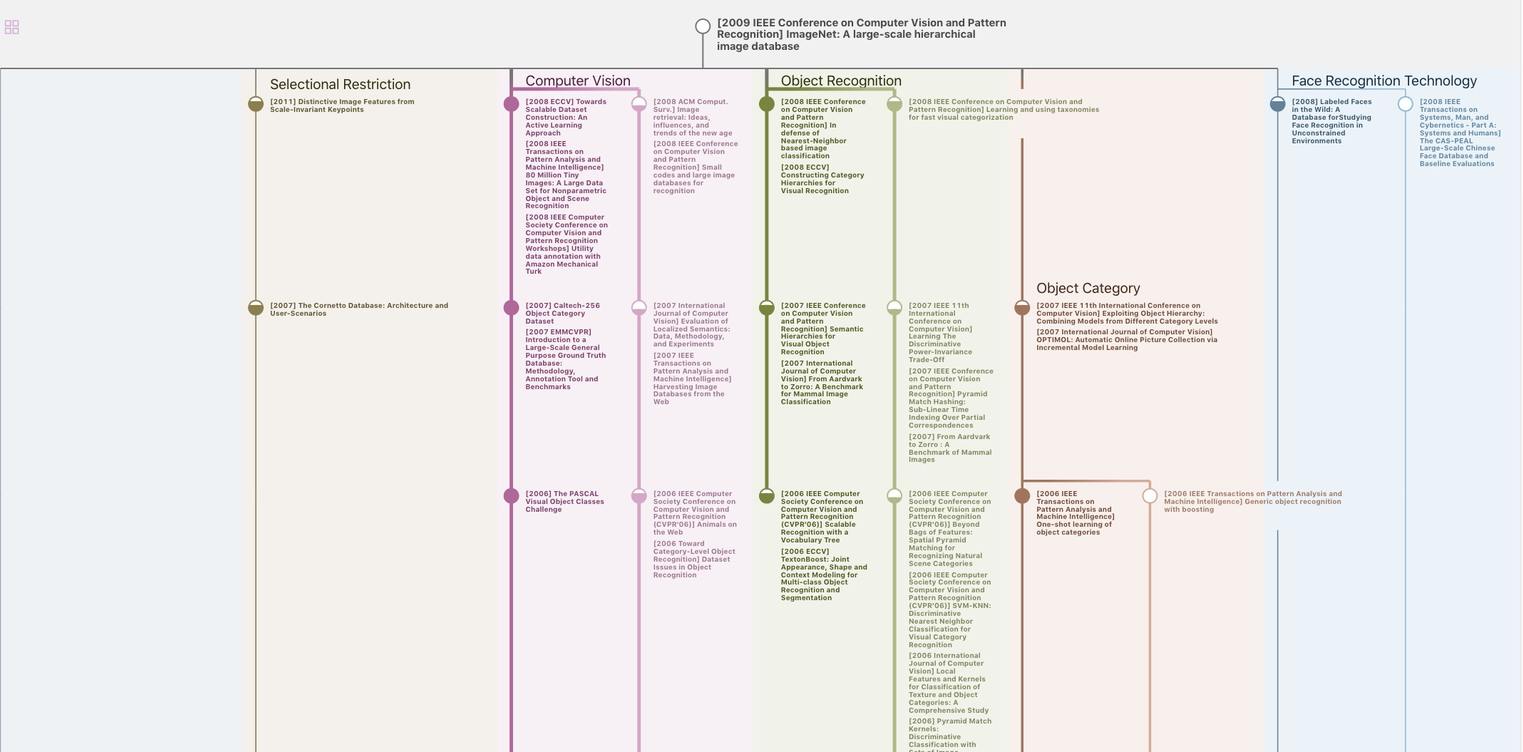
生成溯源树,研究论文发展脉络
Chat Paper
正在生成论文摘要