Deep learning-based hybrid dynamic biased track (DL-HDBT) routing for under water acoustic sensor networks
JOURNAL OF AMBIENT INTELLIGENCE AND HUMANIZED COMPUTING(2020)
摘要
In underwater acoustic sensor networks (UASN), the main challenging issues are bandwidth, higher propagation delay, and heavy packet loss during data transmission. The issues can be solved through efficient routing algorithms. Due to the complexity and variability of the underwater acoustic environment, the underwater acoustic sensor network has the characteristics of fluidity, sparse deployment, and energy limitation, which brings certain challenges to underwater positioning technology. Aiming at the scenario that the node redundancy in the underwater acoustic sensor network leads to low positioning efficiency, this paper has proposed the deep learning-high dynamic biased track (DL-HDBT) algorithm. The DL-HDBT combines the deep learning and hybrid dynamic biased tracking algorithm. Deep learning (DL) helps in the identification of the best relay nodes in the network and traffic-congested nodes are tracked using a high dynamic bias track. The routing protocol has been implemented in the ns2-AqaSim simulator and testbed for measurement of the performance metrics of the UASN. The simulation results showed that the novel routing method throughput has increased by 17%, 35%, and 57% when compared with SUN, VBF and DF method. It can effectively improve the throughput of nodes, balance positioning performance as well as energy use efficiency, and optimize the positioning result of UWASN.
更多查看译文
关键词
Routing, Deep learning, High dynamic biases, Throughput, Underwater acoustic sensor networks, Deep neural network, Tracking
AI 理解论文
溯源树
样例
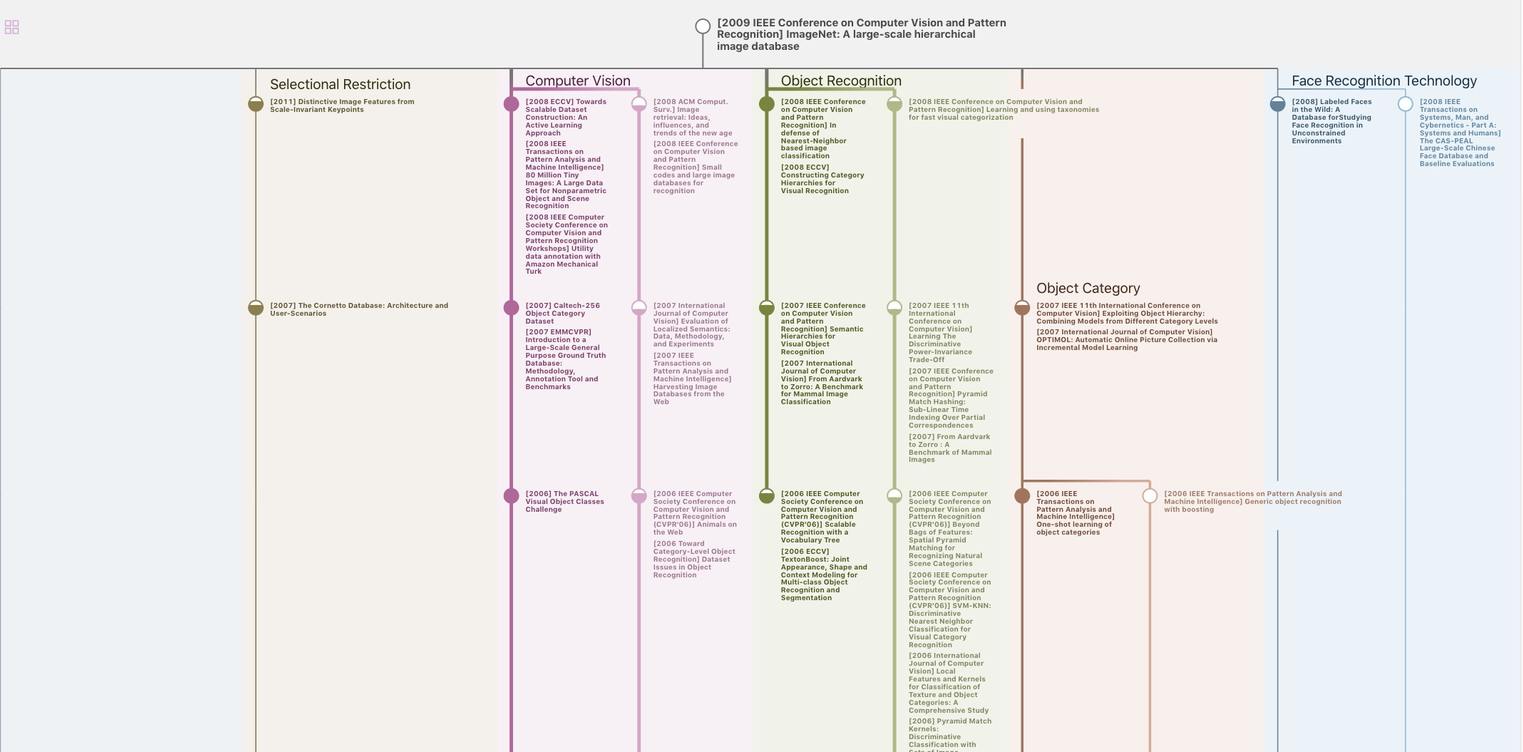
生成溯源树,研究论文发展脉络
Chat Paper
正在生成论文摘要