Crop growth stage estimation prior to canopy closure using deep learning algorithms
NEURAL COMPUTING & APPLICATIONS(2020)
摘要
Growth stage determination plays an important role in yield prediction and cereal husbandry decision-making. Conventionally, crop growth stage determination is performed manually by means of visual inspection. This paper investigates wheat and barley growth stage estimation by classification of proximal images using convolutional neural networks (ConvNets). A dataset consisting of 138,000 images captured prior to the crop canopy closure stage was acquired from 4 sites (7 different fields) in Ireland. The dataset includes images of 12 growth stages of wheat and 11 growth stages of barley captured for a number of crop varieties, seed rates and brightness levels. A camera was held at 2 m from the ground and two camera poses were used—downward-looking and declined to 45^∘ below the horizon. Classification was carried out using three different machine learning approaches: (1) a 5-layer ConvNet model, including three convolutional layers, which was trained from scratch on our crop dataset; (2) transfer learning based on a VGG19 network pre-trained on ImageNet with an additional four fully connected layers, and (3) a support vector machine with conventional feature extraction. The classification accuracies of the aforementioned models were found to be (1) 91.1–94.2
更多查看译文
关键词
Convolutional neural networks, Transfer learning, Cereal growth stage, SVM classifier
AI 理解论文
溯源树
样例
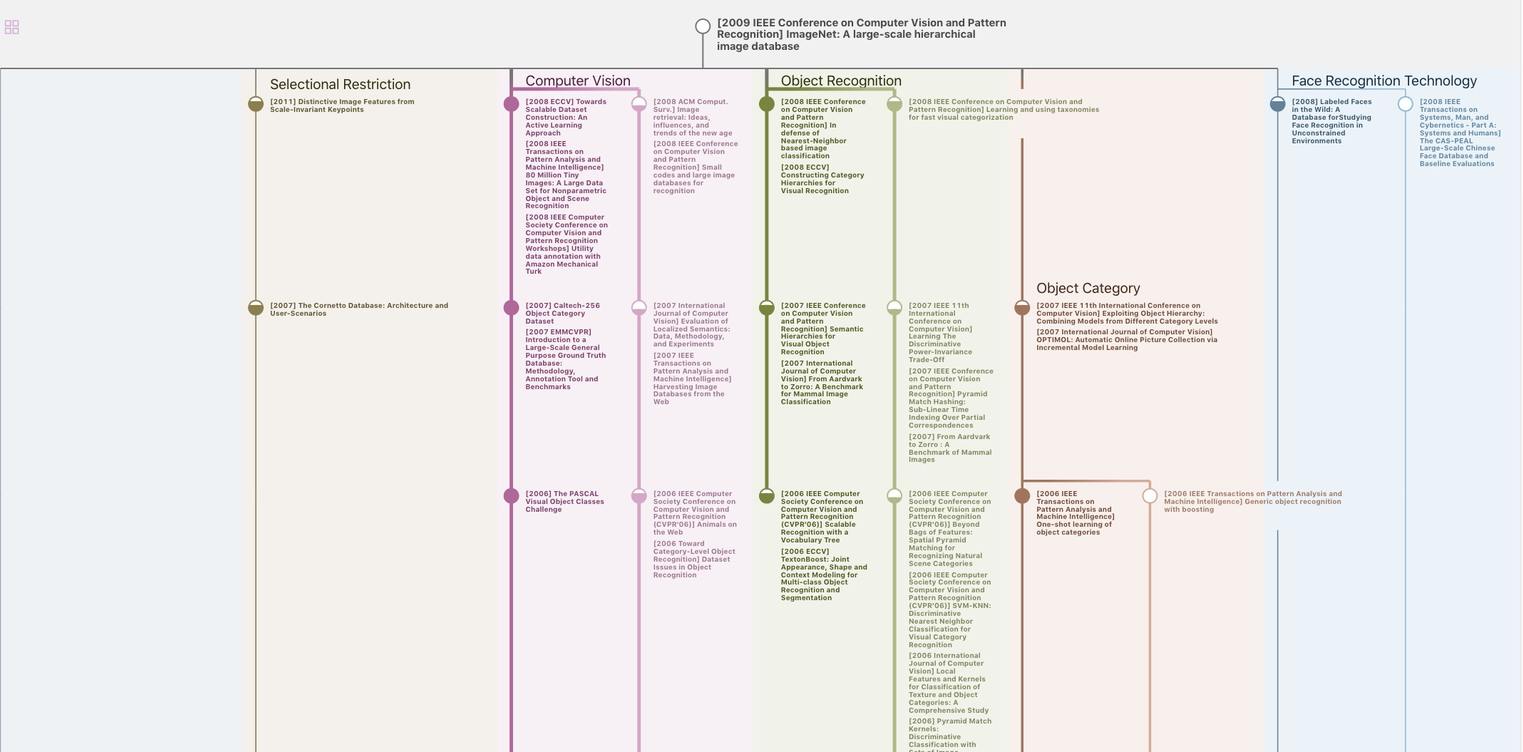
生成溯源树,研究论文发展脉络
Chat Paper
正在生成论文摘要