A novel Directional Framework for Source Counting and Source Separation in Instantaneous Underdetermined audio mixtures
IEEE-ACM TRANSACTIONS ON AUDIO SPEECH AND LANGUAGE PROCESSING(2020)
摘要
The audio source separation problem is a well-known problem that was addressed using a variety of techniques. A common setback in these techniques is that the total number of sound sources in the audio mixture must be known beforehand. However, this knowledge is not always available and thus needs to be estimated. Many approaches have attempted to estimate the number of sources in an audio mixture. There are several clustering techniques that can count the sources in an audio mixture, nonetheless, there are cases, where the directionality of the audio data in the mixture may lead these techniques to failure. In this article, we propose a generalised Directional Fuzzy C-Means (DFCM) framework that offers a complete multi-dimensional, directional solution to this problem. Our proposal shows remarkably high performance in estimating the correct number of sources in the majority of the cases and in addition, it can be used as an effective mechanism to separate the sources. The complete source counting-separation framework can act as a robust low-complexity simultaneous solution to both problems.
更多查看译文
关键词
Audio source counting,audio source separation,directional data,fuzzy c-means,multi-dimensional data
AI 理解论文
溯源树
样例
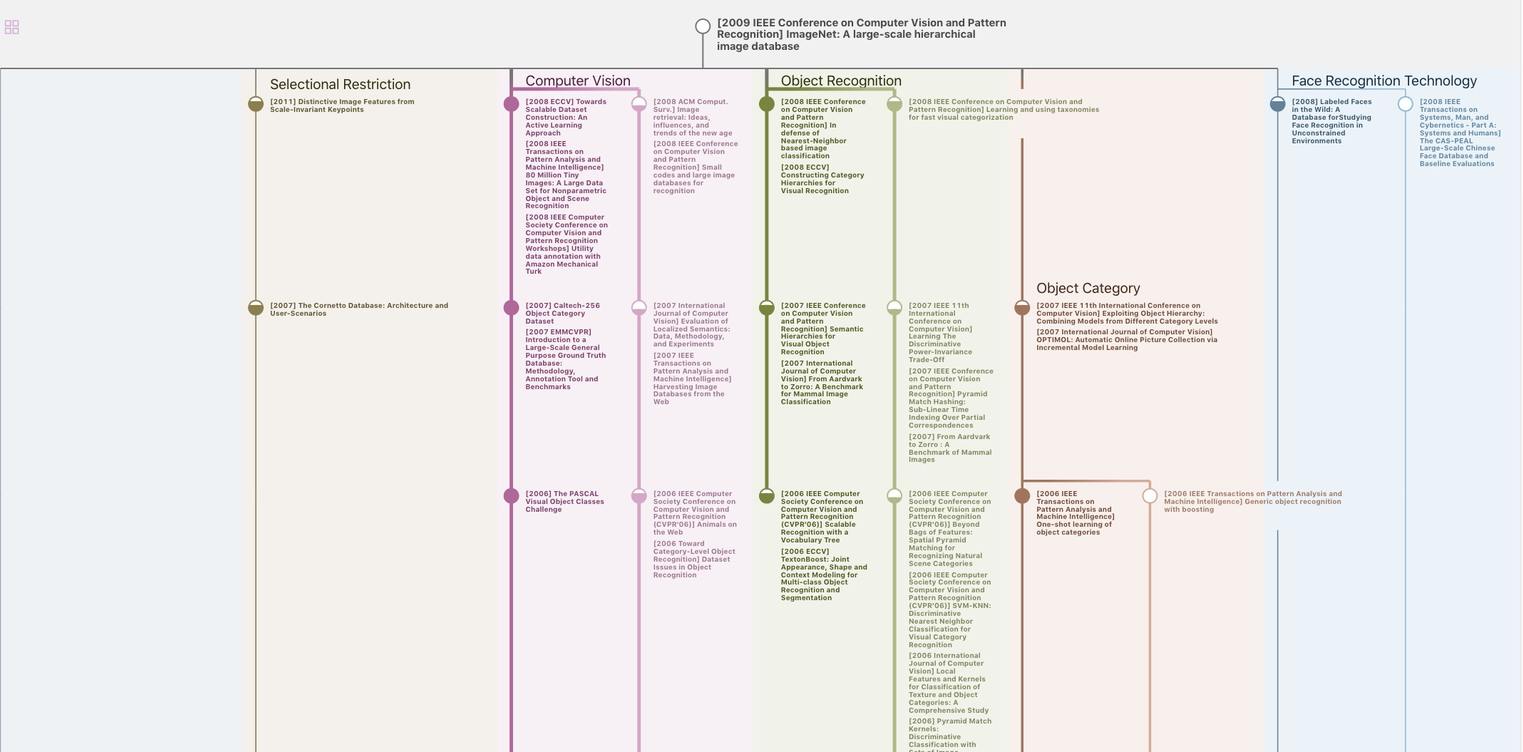
生成溯源树,研究论文发展脉络
Chat Paper
正在生成论文摘要