Soft Regression Of Monocular Depth Using Scale-Semantic Exchange Network
IEEE ACCESS(2020)
摘要
This paper focuses on depth estimation from single monocular image. Most of existing methods regress depth values or classify depth labels, based on single scale feature representations. However, neither regression nor classification can avoid their inherent defects. Single scale context and low-level semantic cannot support accurate depth estimations. We innovatively address single monocular depth estimation by performing soft regression on probability distribution of classification generated by our proposed scale-semantic exchange network (SSE-Net). Our network maintains rich high-resolution representations. With adding high-to-low resolution to form more stages, the repeated context fusions guarantee each representation receives scale information from other parallel representations over and over. Mutual channel attention mechanism is proposed for emphasizing specific semantics of feature representation. We allow each depth class to be shifted and scaled adaptively. Depth estimation is calculated as expected value of probability distribution. The experimental results verify the efficiency of every proposed components and show competitive results compared with the recent state-of-the-art methods.
更多查看译文
关键词
Estimation, Semantics, Probability distribution, Image segmentation, Learning systems, Deep learning, Image resolution, Depth estimation, scale-semantic exchange network, mutual attention, soft regression
AI 理解论文
溯源树
样例
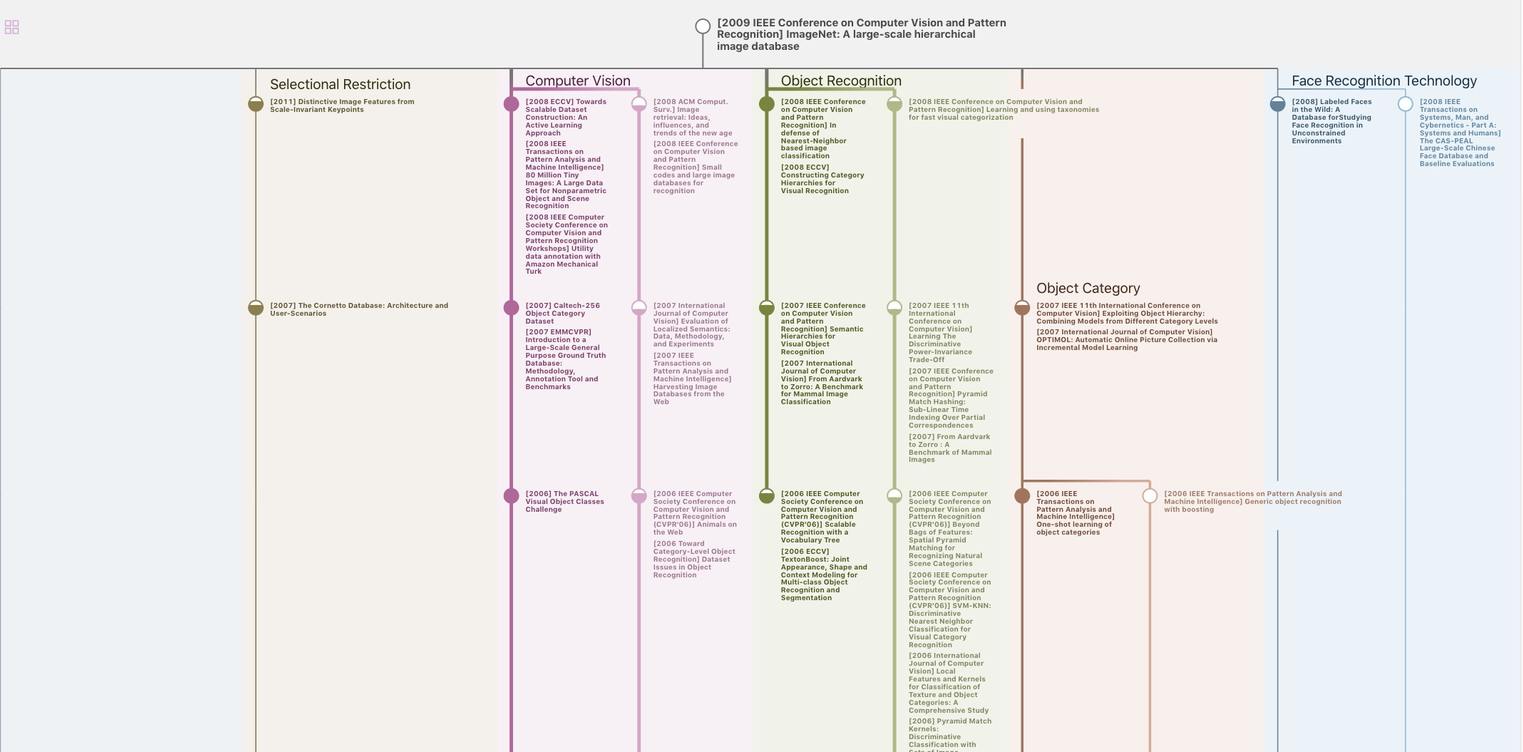
生成溯源树,研究论文发展脉络
Chat Paper
正在生成论文摘要