Developing New Colored Dissolved Organic Matter Retrieval Algorithms Based on Sparse Learning
IEEE JOURNAL OF SELECTED TOPICS IN APPLIED EARTH OBSERVATIONS AND REMOTE SENSING(2020)
摘要
Colored dissolved organic matter (CDOM) is an important biochemical state indicator of aquatic ecosystems. However, its retrieval from remote sensing datasets remains a challenging task due to its high spatiotemporal variability and interference from other water constituents. In this article, we aim to develop new CDOM inversion algorithms by taking advantage of a representative sparse learning algorithm known as least absolute shrinkage and selection operator (LASSO), which is applied to identify the optimal band arithmetic terms for the CDOM inversion and estimate the model parameters in a more global and robust manner than can statistics-based methods. Moreover, a two-stage inversion framework is presented to further enhance the stability of LASSO in addressing inadequate in situ sample circumstances. Within the framework, two different schemes are proposed to handle the band arithmetic terms information propagation between the two stages, for which two new algorithms are proposed. Experimental results obtained from the in situ bio-optical dataset and the synthesized dataset under various training sample sizes indicate that both of the proposed algorithms can deliver performance superior to six state-of-the-art CDOM inversion algorithms, and with less sensitivity to the training sample states. In addition, the results offered by the new algorithms also enjoy biochemical interpretability, revealing that the red/blue ratio terms are well suited for inverting the CDOM content on these datasets.
更多查看译文
关键词
Colored dissolved organic matter (CDOM),remote sensing,sparse learning,water quality inversion
AI 理解论文
溯源树
样例
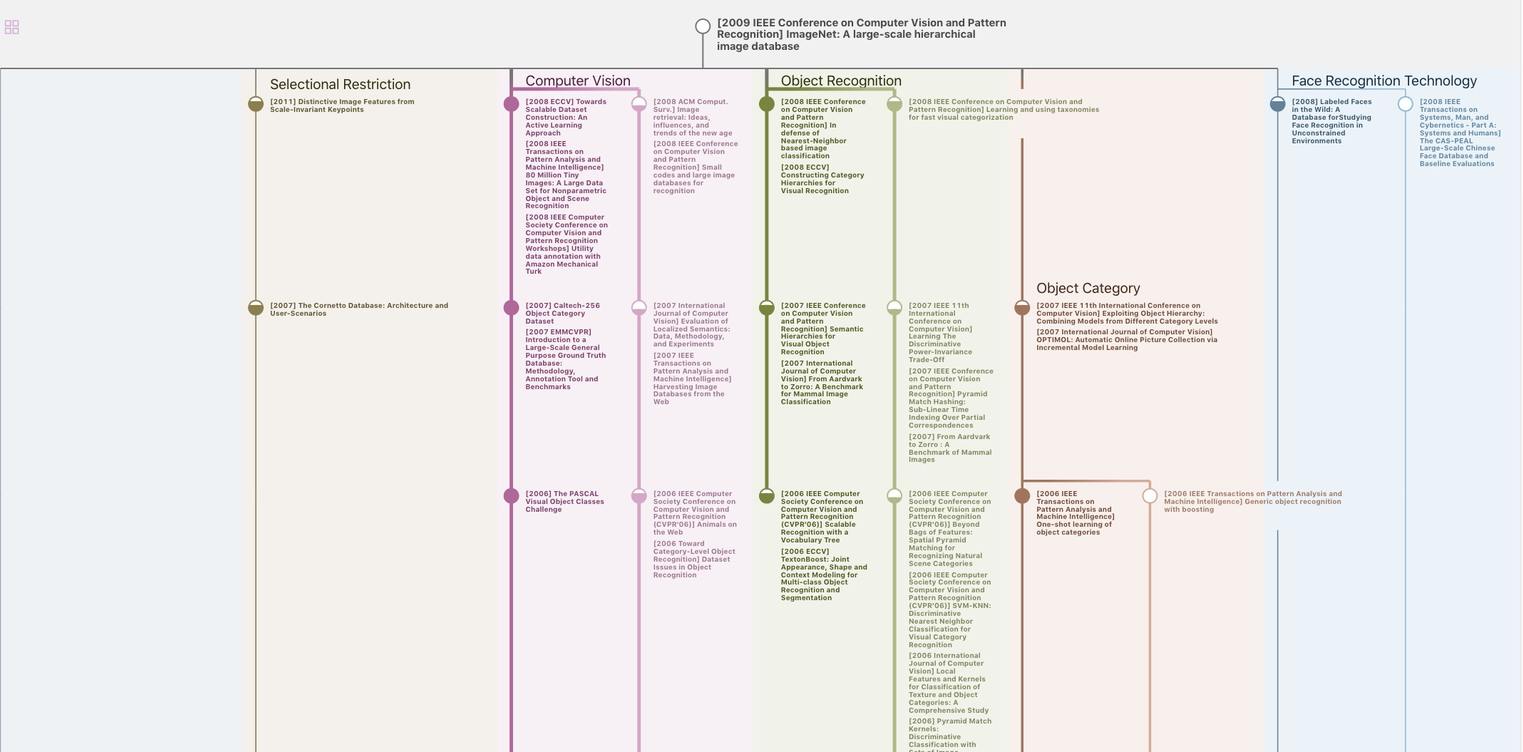
生成溯源树,研究论文发展脉络
Chat Paper
正在生成论文摘要