Groupwise Non-Rigid Registration with Deep Learning: An Affordable Solution Applied to 2D Cardiac Cine MRI Reconstruction
ENTROPY(2020)
摘要
Groupwise image (GW) registration is customarily used for subsequent processing in medical imaging. However, it is computationally expensive due to repeated calculation of transformations and gradients. In this paper, we propose a deep learning (DL) architecture that achieves GW elastic registration of a 2D dynamic sequence on an affordable average GPU. Our solution, referred to as dGW, is a simplified version of the well-known U-net. In our GW solution, the image that the other images are registered to, referred to in the paper astemplate image, is iteratively obtained together with the registered images. Design and evaluation have been carried out using 2D cine cardiac MR slices from 2 databases respectively consisting of 89 and 41 subjects. The first database was used for training and validation with 66.6-33.3% split. The second one was used for validation (50%) and testing (50%). Additional network hyperparameters, which are-in essence-those that control the transformation smoothness degree, are obtained by means of a forward selection procedure. Our results show a 9-fold runtime reduction with respect to an optimization-based implementation; in addition, making use of the well-known structural similarity (SSIM) index we have obtained significative differences with dGW with respect to an alternative DL solution based on Voxelmorph.
更多查看译文
关键词
non-rigid registration,elastic motion,CNN,deep learning
AI 理解论文
溯源树
样例
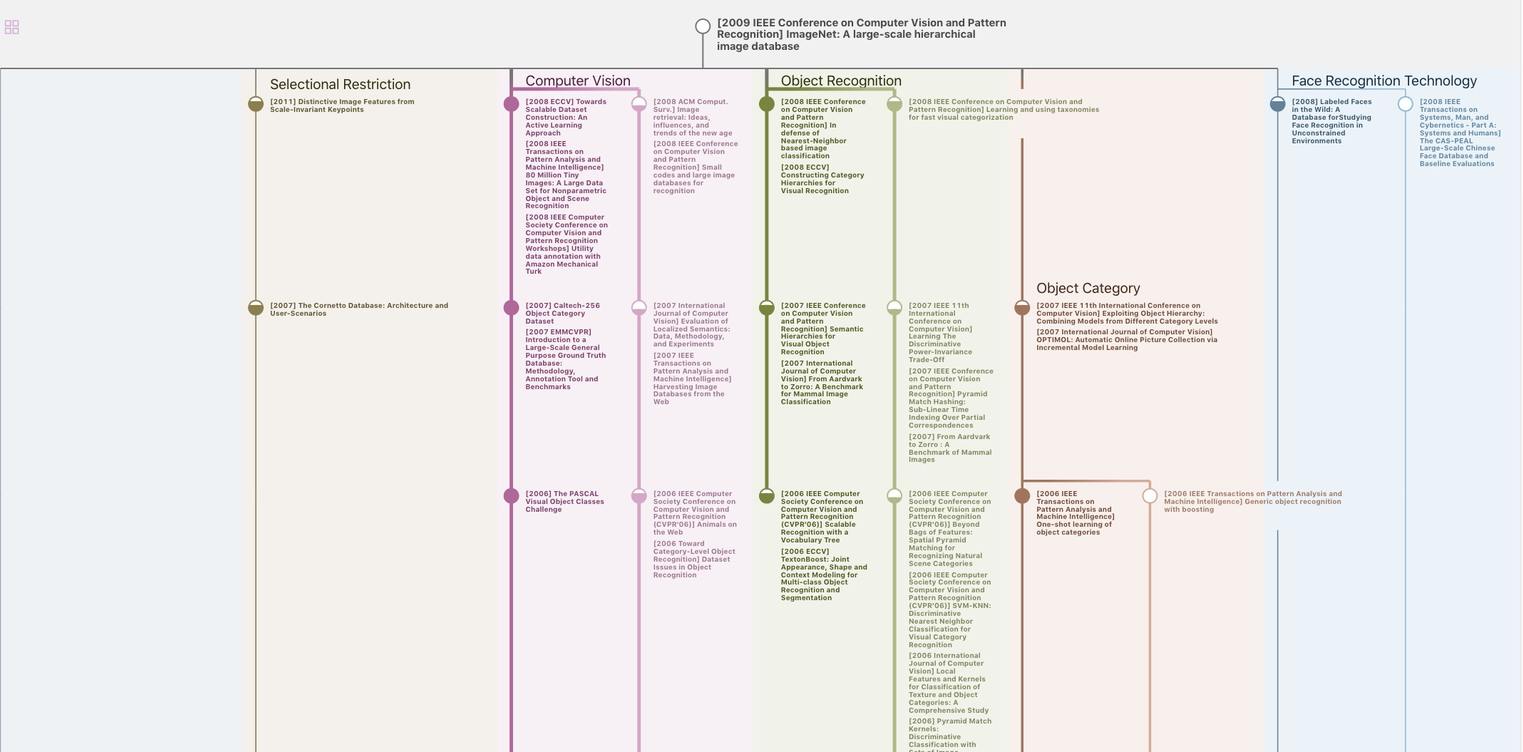
生成溯源树,研究论文发展脉络
Chat Paper
正在生成论文摘要