A Probabilistic Infection Model for Efficient Trace-Prediction of Disease Outbreaks in Contact Networks
international conference on computational science(2020)
摘要
We propose a novel method which we call the Probabilistic Infection Model (PIM). Instead of stochastically assigning exactly one state to each agent at a time, PIM tracks the likelihood of each agent being in a particular state. Thus, a particular agent can exist in multiple disease states concurrently. Our model gives an improved resolution of transitions between states, and allows for a more comprehensive view of outbreak dynamics at the individual level. Moreover, by using a probabilistic approach, our model gives a representative understanding of the overall trajectories of simulated outbreaks without the need for numerous (order of hundreds) of repeated Monte Carlo simulations. We simulate our model over a contact network constructed using registration data of university students. We model three diseases; measles and two strains of influenza. We compare the results obtained by PIM with those obtained by simulating stochastic SEIR models over the same the contact network. The results demonstrate that the PIM can successfully replicate the averaged results from numerous simulations of a stochastic model in a single deterministic simulation.
更多查看译文
关键词
Computational epidemics, Outbreak simulation, SEIR model
AI 理解论文
溯源树
样例
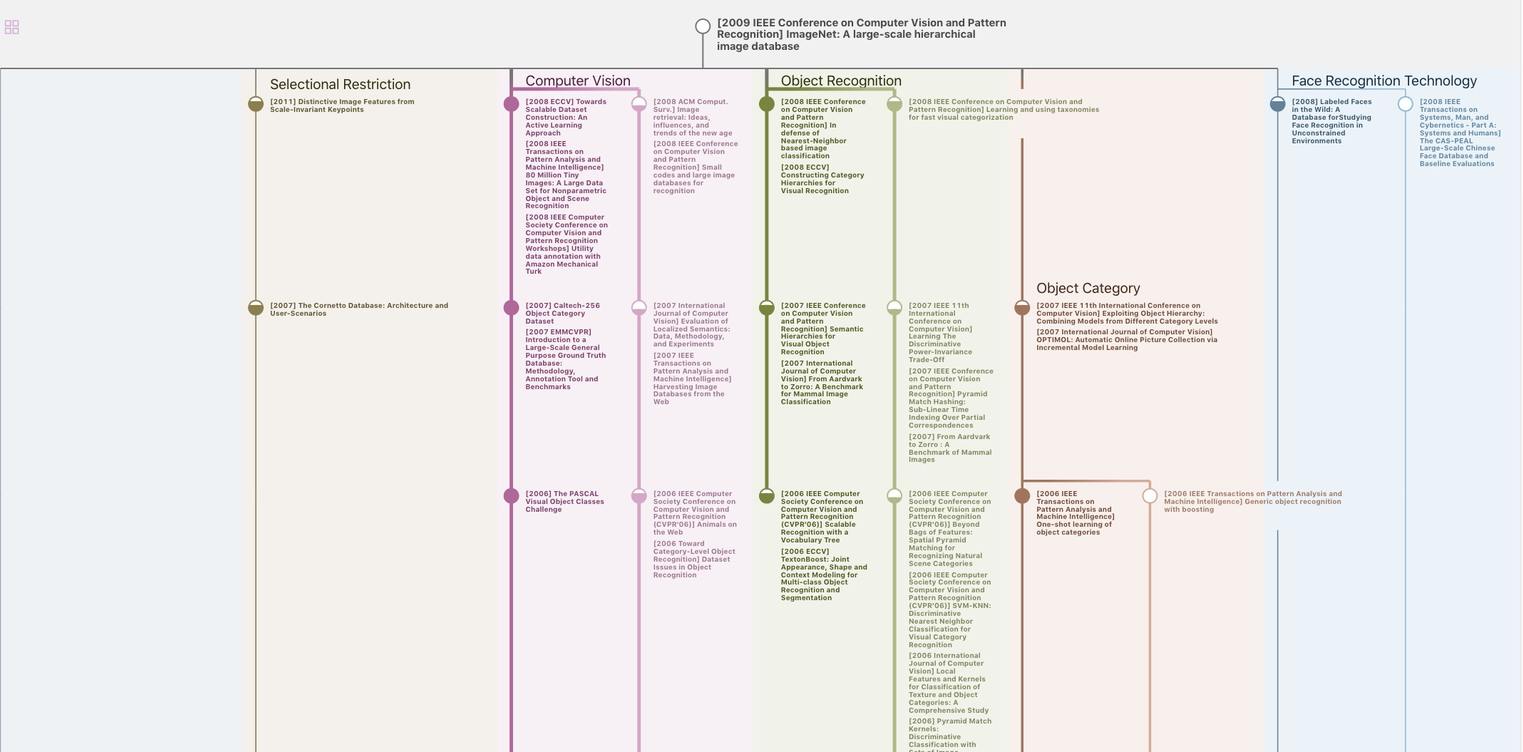
生成溯源树,研究论文发展脉络
Chat Paper
正在生成论文摘要