Learning To Align Via Wasserstein For Person Re-Identification
IEEE TRANSACTIONS ON IMAGE PROCESSING(2020)
摘要
Existing successful person re-identification (Re-ID) models often employ the part-level representation to extract the fine-grained information, but commonly use the loss that is particularly designed for global features, ignoring the relationship between semantic parts. In this paper, we present a novel triplet loss that emphasizes the salient parts and also takes the consideration of alignment. This loss is based on the crossing-bing matching metric that also known as Wasserstein Distance. It measures how much effort it would take to move the embeddings of local features to align two distributions, such that it is able to find an optimal transport matrix to re-weight the distance of different local parts. The distributions in support of local parts is produced via a new attention mechanism, which is calculated by the inner product between high-level global feature and local features, representing the importance of different semantic parts w.r.t. identification. We show that the obtained optimal transport matrix can not only distinguish the relevant and misleading parts, and hence assign different weights to them, but also rectify the original distance according to the learned distributions, resulting in an elegant solution for the mis-alignment issue. Besides, the proposed method is easily implemented in most Re-ID learning system with end-to-end training style, and can obviously improve their performance. Extensive experiments and comparisons with recent Re-ID methods manifest the competitive performance of our method.
更多查看译文
关键词
Semantics, Heating systems, Measurement, Learning systems, Training, Estimation, Feature extraction, Person re-identification, deep metric learning, convolutional neural network, Wasserstein distance
AI 理解论文
溯源树
样例
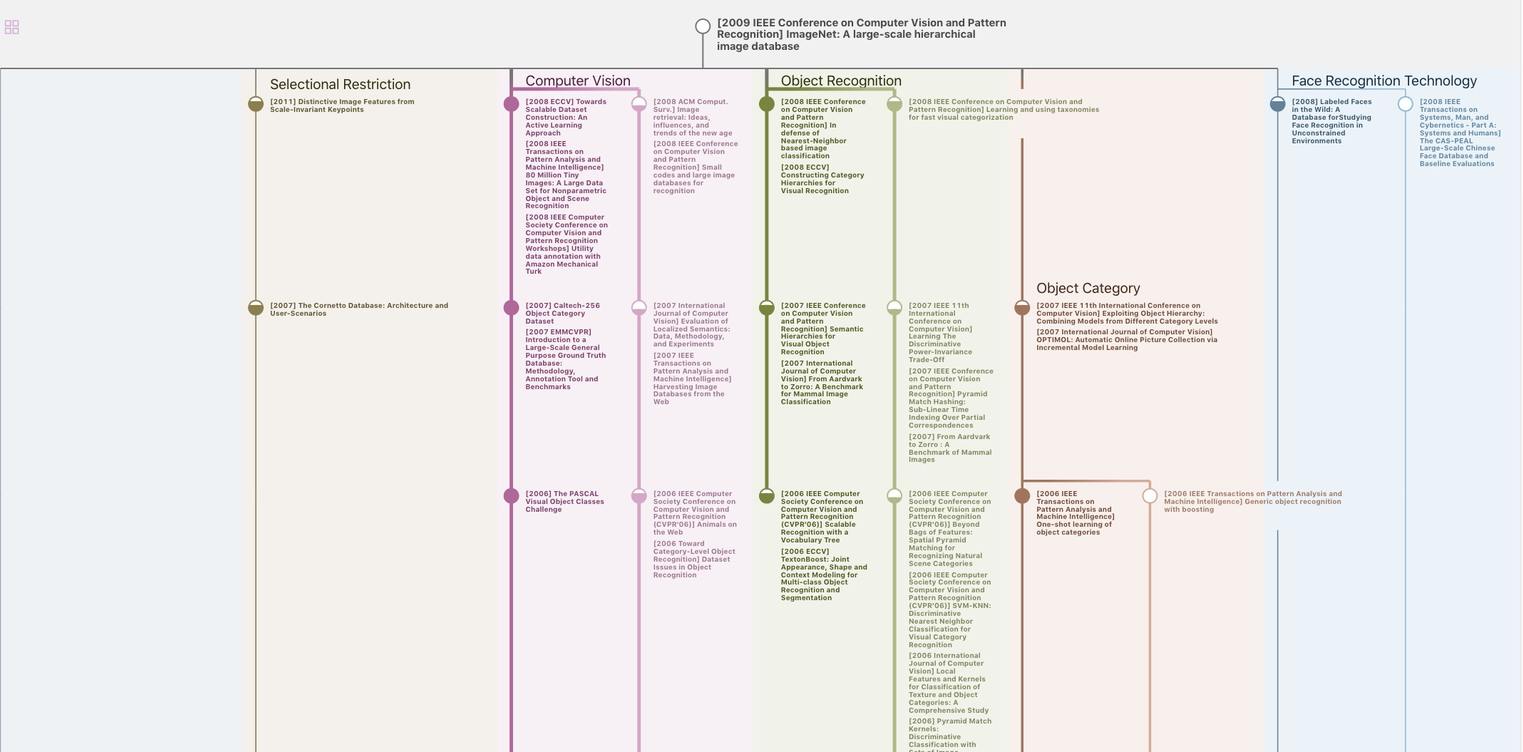
生成溯源树,研究论文发展脉络
Chat Paper
正在生成论文摘要