B-Scan Attentive Cnn For The Classification Of Retinal Optical Coherence Tomography Volumes
IEEE SIGNAL PROCESSING LETTERS(2020)
摘要
Optical coherence tomography (OCT) enables 3D cross-sectional imaging of the retinal tissues and has become an essential tool for the diagnosis of eye diseases. Clinically, the ophthalmologists examine each cross-sectional image (B- scan) of the 3D OCT volume to diagnose the retinal pathologies. However, this process is time-consuming and tedious. Automated methods in literature classify the individual B-scans and aggregate the diagnosis decision for the OCT volume using manual threshold-based rules. However, these methods lack generalizability and fail to incorporate the ophthalmologists' aspects of clinical diagnosis. Therefore, in this letter, we propose a B-scan attentive convolutional neural network (BACNN) that mimics the ophthalmologists' way of diagnosis by focusing on the clinically informative B- scans during the classification process. Specifically, a CNN based feature extraction module is used to extract spatial feature representations from the B-scans. Then, a self-attention module aggregates these features based on their clinical relevance to obtain a discriminative high-level feature vector for a reliable diagnosis. The experimental results on two clinical OCT datasets indicate that the proposed method provides improved classification performance. The method also demonstrates the correlation of the pathological symptoms in the B- scans with the attention weights.
更多查看译文
关键词
Retina, Pathology, Feature extraction, Three-dimensional displays, Diseases, Imaging, Reliability, Attention, classification, convolutional neural network (CNN), optical coherence tomography (OCT) volume
AI 理解论文
溯源树
样例
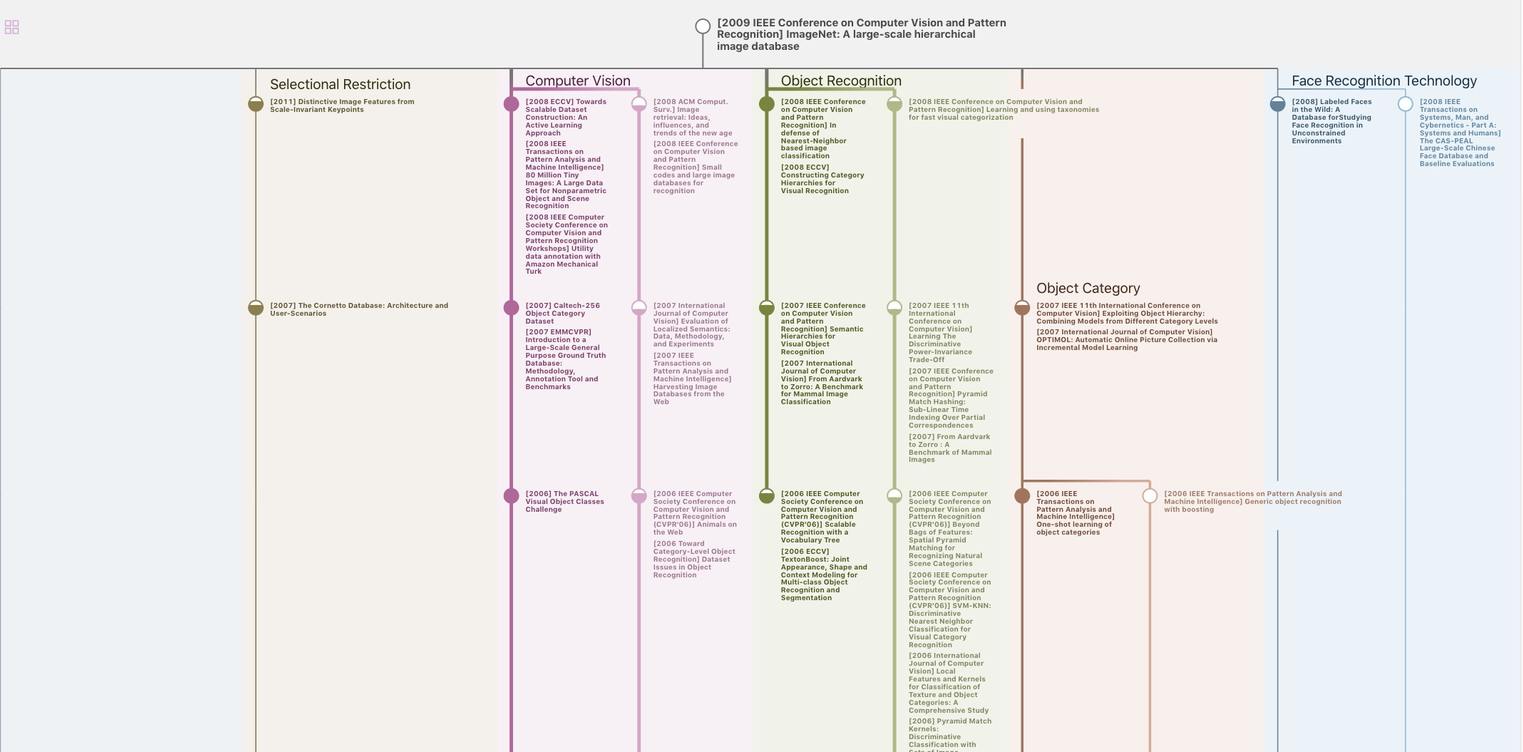
生成溯源树,研究论文发展脉络
Chat Paper
正在生成论文摘要