Modeling Spatial Trajectories with Attribute Representation Learning (Extended Abstract).
IEEE Transactions on Knowledge and Data Engineering(2023)
摘要
The widespread use of positioning devices has given rise to many trajectories, with each having three explicit attributes: user ID, location ID, and time-stamp and an implicit attribute: activity type (akin to "topic" in text mining). To model these trajectories, existing works learn different attribute representations by either introducing latent activity types based on topic models or transforming the location and time context into a low-dimensional space via embedding techniques. In this paper, we propose a holistic approach named Human Mobility Representation Model (HMRM) to simultaneously produce the vector representations of all four (explicit and implicit) attributes. We evaluate HMRM on two real check-in datasets collected from Foursquare. Experimental results show that HMRM could not only improve the performance of capturing latent activity types, but also learn better trajectory embeddings.
更多查看译文
关键词
Trajectory,Semantics,Tensors,Task analysis,Data models,Context modeling,Collaborative work
AI 理解论文
溯源树
样例
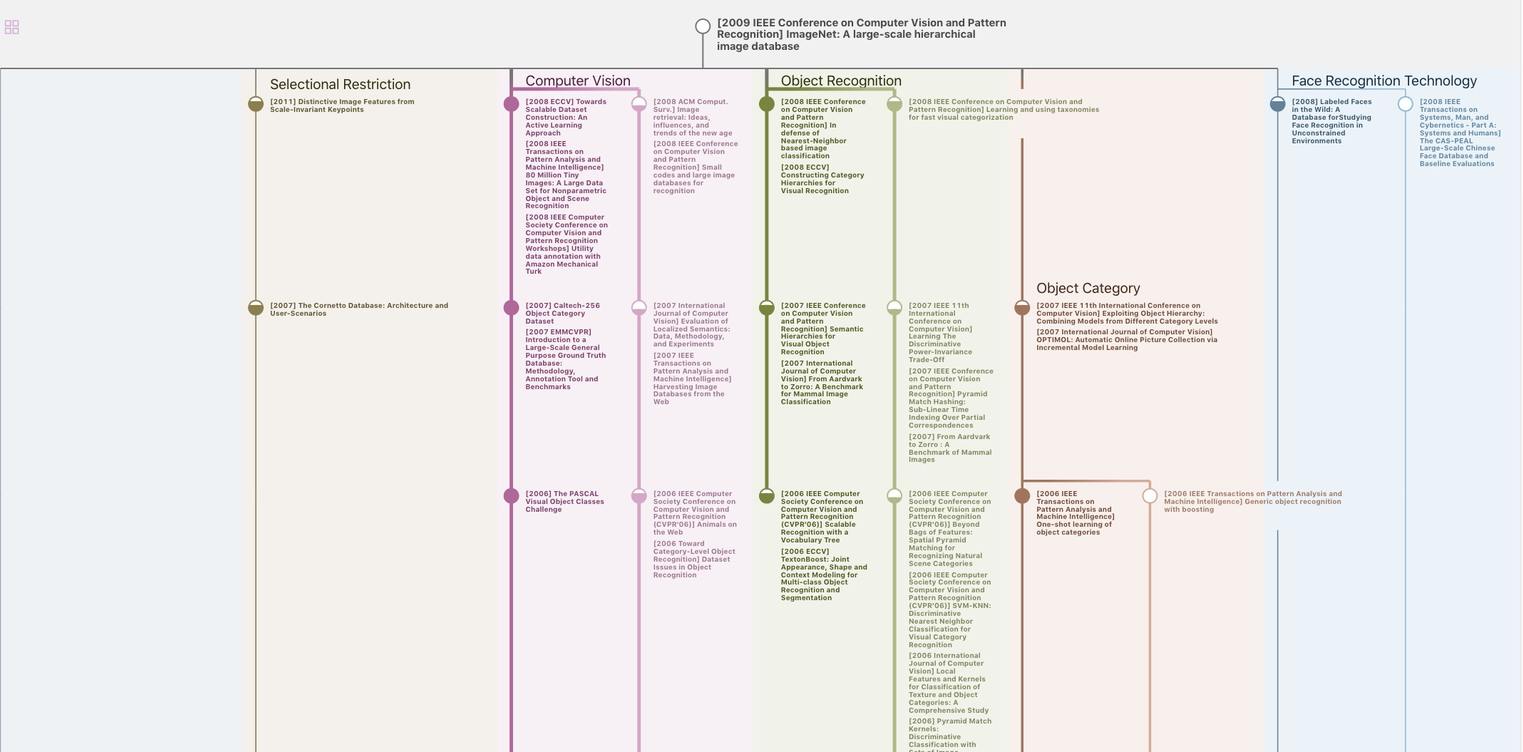
生成溯源树,研究论文发展脉络
Chat Paper
正在生成论文摘要