MGHCNET: A Deep Multi-Scale Granular and Holistic Channel Feature Generation Network for Image Super Resolution
2020 IEEE INTERNATIONAL CONFERENCE ON MULTIMEDIA AND EXPO (ICME)(2020)
摘要
Residual blocks use skip connections in order to facilitate the flow of information in the network and thus, provide a good network performance. As different objects in a generic image appear at different scales, employing a multi-scale feature generation module in a residual block for image super resolution can further improve the network performance. In this paper, a new residual block that generates features at multiple scales is proposed for the task of image super resolution. In order to enhance the representational capability of the network while keeping its complexity low, the proposed residual block uses two different feature generation techniques, namely, multi-scale granular channel feature generation and uni-scale holistic channel feature generation, and fuses their output feature maps. It is shown that the network using the proposed residual block outperforms the state-of-the-art lightweight super resolution networks on four benchmark datasets with various scaling factors.
更多查看译文
关键词
Deep Learning, Multi-scale Feature Generation, Image Super Resolution
AI 理解论文
溯源树
样例
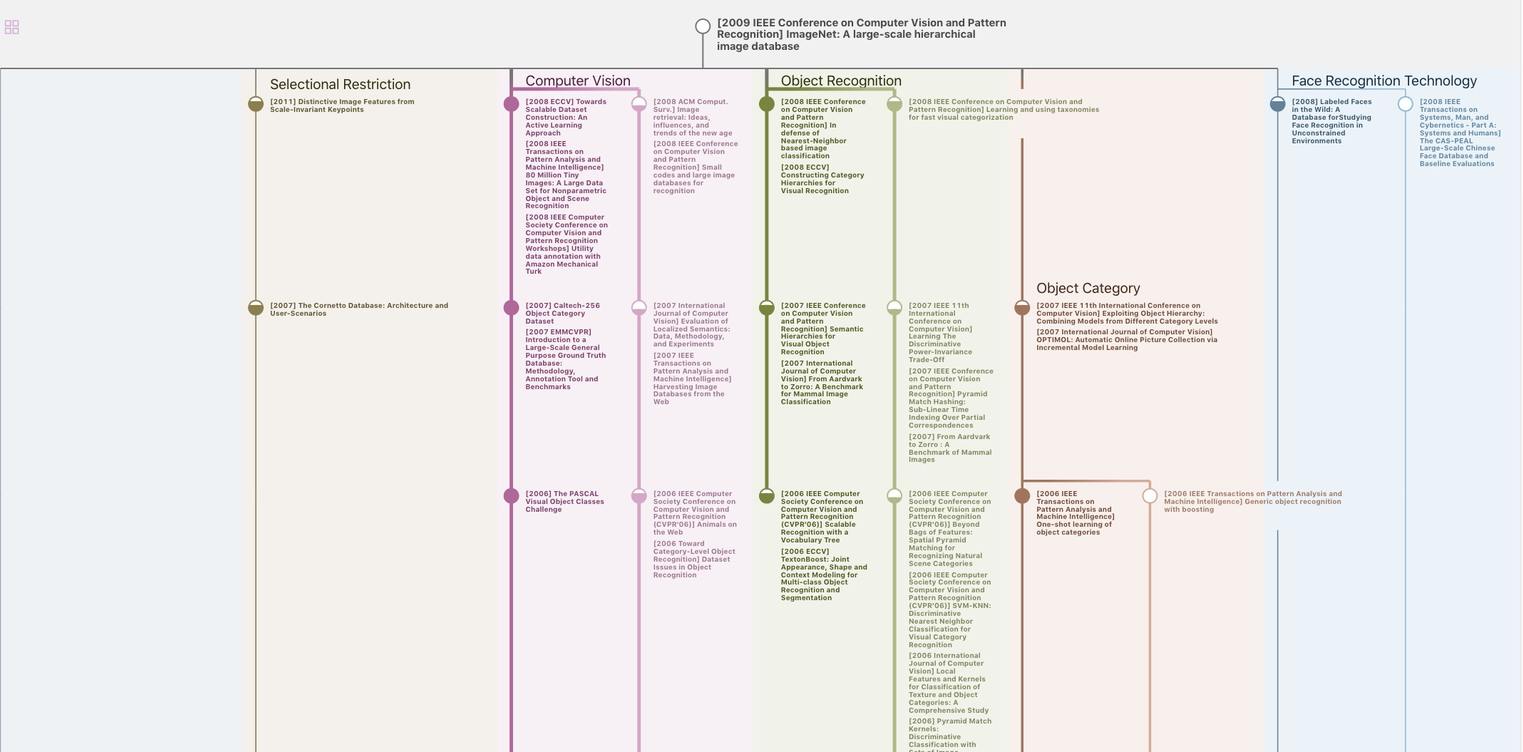
生成溯源树,研究论文发展脉络
Chat Paper
正在生成论文摘要