Adaptive Deep Metric Learning For Affective Image Retrieval And Classification
IEEE TRANSACTIONS ON MULTIMEDIA(2021)
摘要
An image is worth a thousand words. Many researchers have conducted extensive studies to understand visual emotions since an increasing number of users express emotions via images and videos online. However, most existing methods based on convolutional neural networks aim to retrieve and classify affective images in a discrete label space while ignoring both the hierarchical and complex nature of emotions. On the one hand, different from concrete and isolated object concepts (e.g., cat and dog), a hierarchical relationship exists among emotions. On the other hand, most widely used deep methods depend on the representation from fully connected layers, which lacks the essential texture information for recognizing emotions. In this work, we address the above problems via adaptive deep metric learning. Specifically, we design an adaptive sentiment similarity loss, which is able to embed affective images considering the emotion polarity and adaptively adjust the margin between different image pairs. To effectively distinguish affective images, we further propose the sentiment vector that captures the texture information extracted from multiple convolutional layers. Finally, we develop a unified multi-task deep framework to simultaneously optimize both retrieval and classification goals. Extensive and thorough evaluations on four benchmark datasets demonstrate that the proposed framework performs favorably against the state-of-the-art methods.
更多查看译文
关键词
Measurement, Visualization, Semantics, Feature extraction, Task analysis, Image analysis, Image retrieval, Affective image retrieval, convolutional neural network, deep metric learning, visual sentiment analysis
AI 理解论文
溯源树
样例
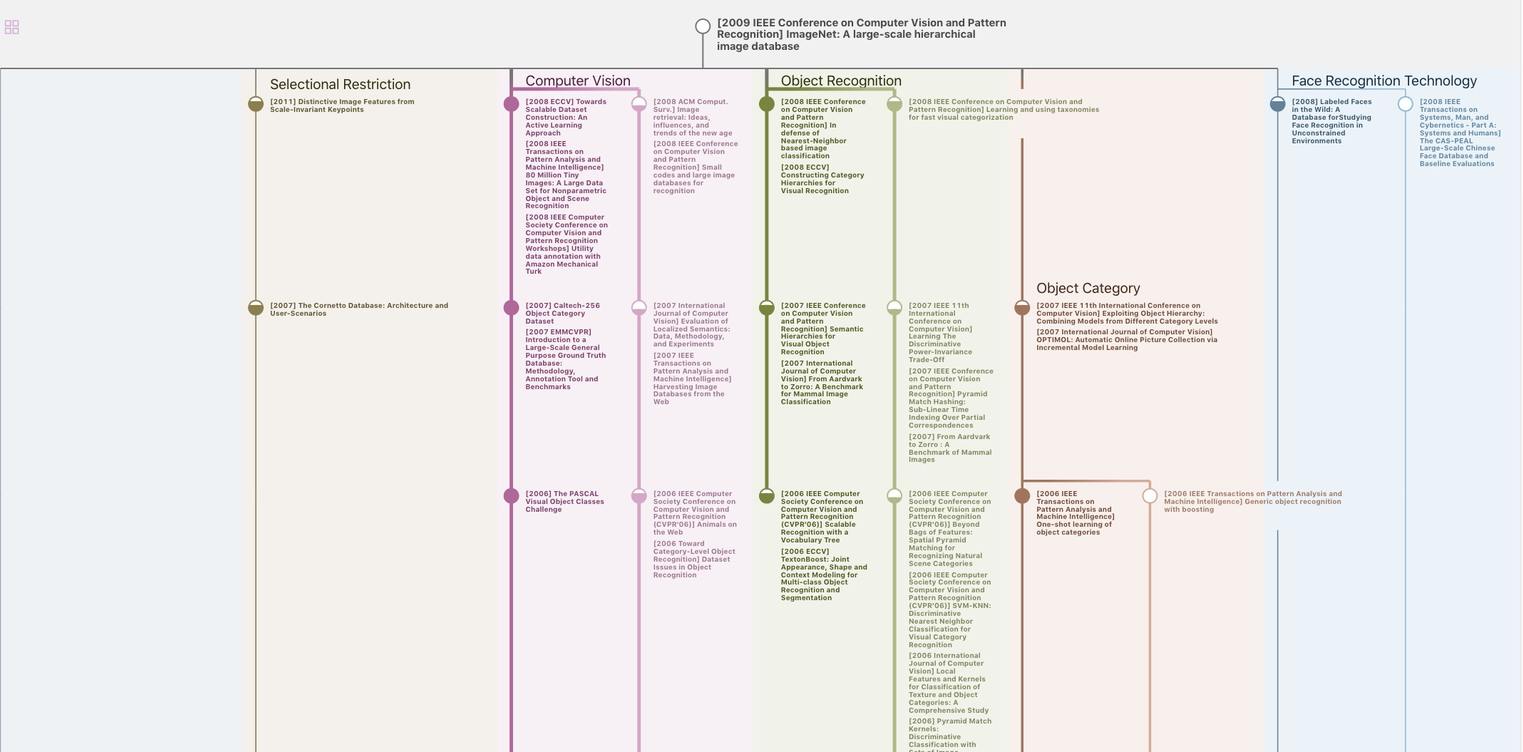
生成溯源树,研究论文发展脉络
Chat Paper
正在生成论文摘要