Streaming Algorithms For Robust Submodular Maximization
DISCRETE APPLIED MATHEMATICS(2021)
摘要
Submodular maximization is well studied in the fields of data mining and machine learning. We study the submodular maximization subject to a cardinality constraint k for large scale scenarios applications under two combined settings. One is that all elements arrive in a streaming fashion, and the other is that some elements may be invalid at last. For this problem, which is called streaming robust submodular maximization (SRSM) problem, we explore an approximation algorithm, returning a subset S from the ground set V with a limit size, such that it represents V and is robust to a broken set E well. Our algorithm only makes one pass over data, and achieves a constant-factor 0.1224 approximation guarantee, independent of the cardinality constraint parameter k. Based on the algorithm for SRSM problem, we continue to discuss this problem over sliding windows, in which we are asked to obtain a proper set that only considers the last W elements, and derive an algorithm with a constant (0.0612-epsilon)-approximation guarantee. At last we also propose numerical experiments on some applications to well demonstrate our algorithm for SRSM problem over sliding windows. (C) 2020 Elsevier B.V. All rights reserved.
更多查看译文
关键词
Streaming robust submodular maximization, Sliding windows, Performance guarantee
AI 理解论文
溯源树
样例
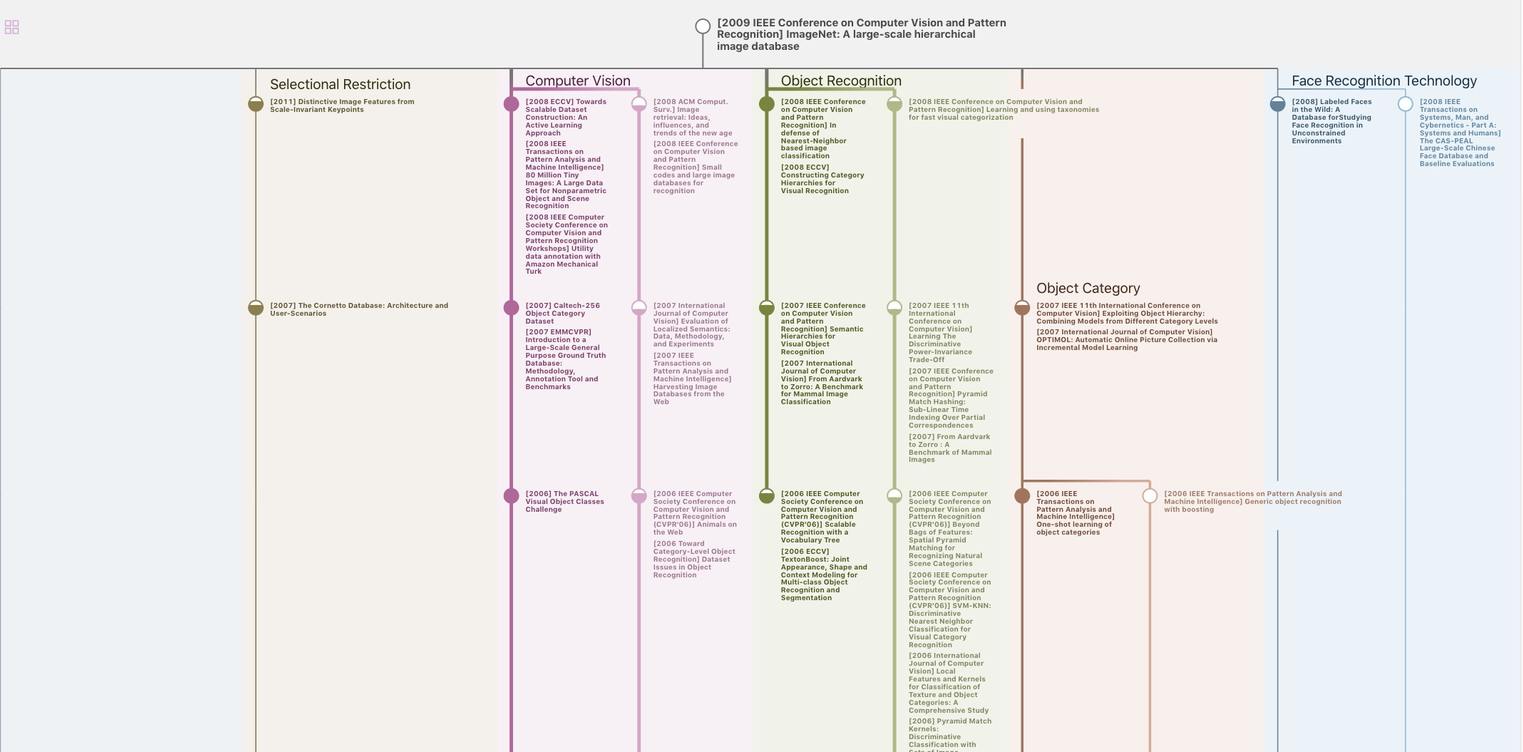
生成溯源树,研究论文发展脉络
Chat Paper
正在生成论文摘要