Simultaneous feature selection and clustering of micro-array and RNA-sequence gene expression data using multiobjective optimization
INTERNATIONAL JOURNAL OF MACHINE LEARNING AND CYBERNETICS(2020)
摘要
In this paper, we have devised a multiobjective optimization solution framework for solving the problem of gene expression data clustering in reduced feature space. Here clustering problem is viewed from two different aspects: clustering of genes in reduced sample space or clustering of samples in reduced gene space. Three objective functions: two internal cluster validity indices and the count on the number of features are optimized simultaneously by a popular multiobjective simulated annealing based approach, namely AMOSA. Here, point symmetry based distance is used for the assignment of gene data points to different clusters. Seven publicly available benchmark gene expression data sets are used for experimental purpose. Both aspects of clustering in reduced feature space is demonstrated. The proposed gene expression clustering technique outperforms the existing nine clustering techniques. Apart from this, also some statistical and biological significant tests have been carried out to show that the proposed FSC-MOO technique is more statistically and biologically enriched
更多查看译文
关键词
Gene expression data clustering,Feature selection,Point symmetry based distance,Multiobjective optimization,Cluster validity index
AI 理解论文
溯源树
样例
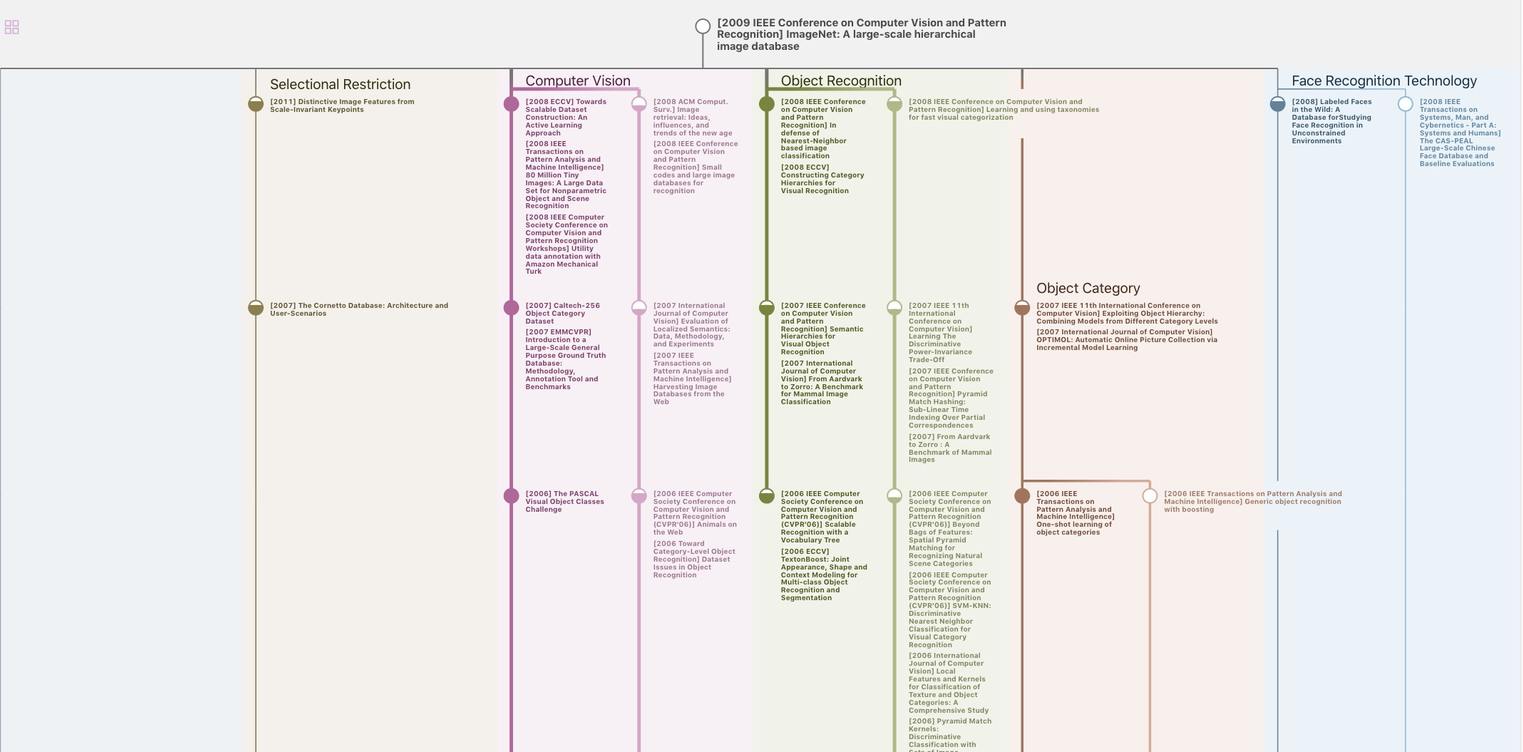
生成溯源树,研究论文发展脉络
Chat Paper
正在生成论文摘要