Dual unsupervised features fusion for hyperspectral image classification
INTERNATIONAL JOURNAL OF REMOTE SENSING(2020)
摘要
An unsupervised feature extraction method could effectively address the phenomenon where using a limited number of labelled samples leads to poor hyperspectral image (HSI) classification. Inspired by this method, we have developed a new method based on fusing dual unsupervised features for HSI classification in this paper. First, we used principal component analysis (PCA) to reduce the dimensions of HSI data and obtain the first set of unsupervised features. Then, we got the second set of unsupervised features by adopting the self-taught learning method, which could learn more discriminatory features by making full use of the information of unlabelled samples in the same HSI. Next, we used the correlation canonical analysis (CCA) algorithm to fuse linearly dual unsupervised learning features. Finally, we proved the effectiveness of the features extracted by the proposed algorithm by applying the extreme learning machine (ELM) classifier to evaluate the purposes. Experiments with three widely used real HSI datasets showed good classification performance when the number of training samples was quite limited. This demonstrates that the proposed feature learning method was indeed an effective method for HSI classification.
更多查看译文
AI 理解论文
溯源树
样例
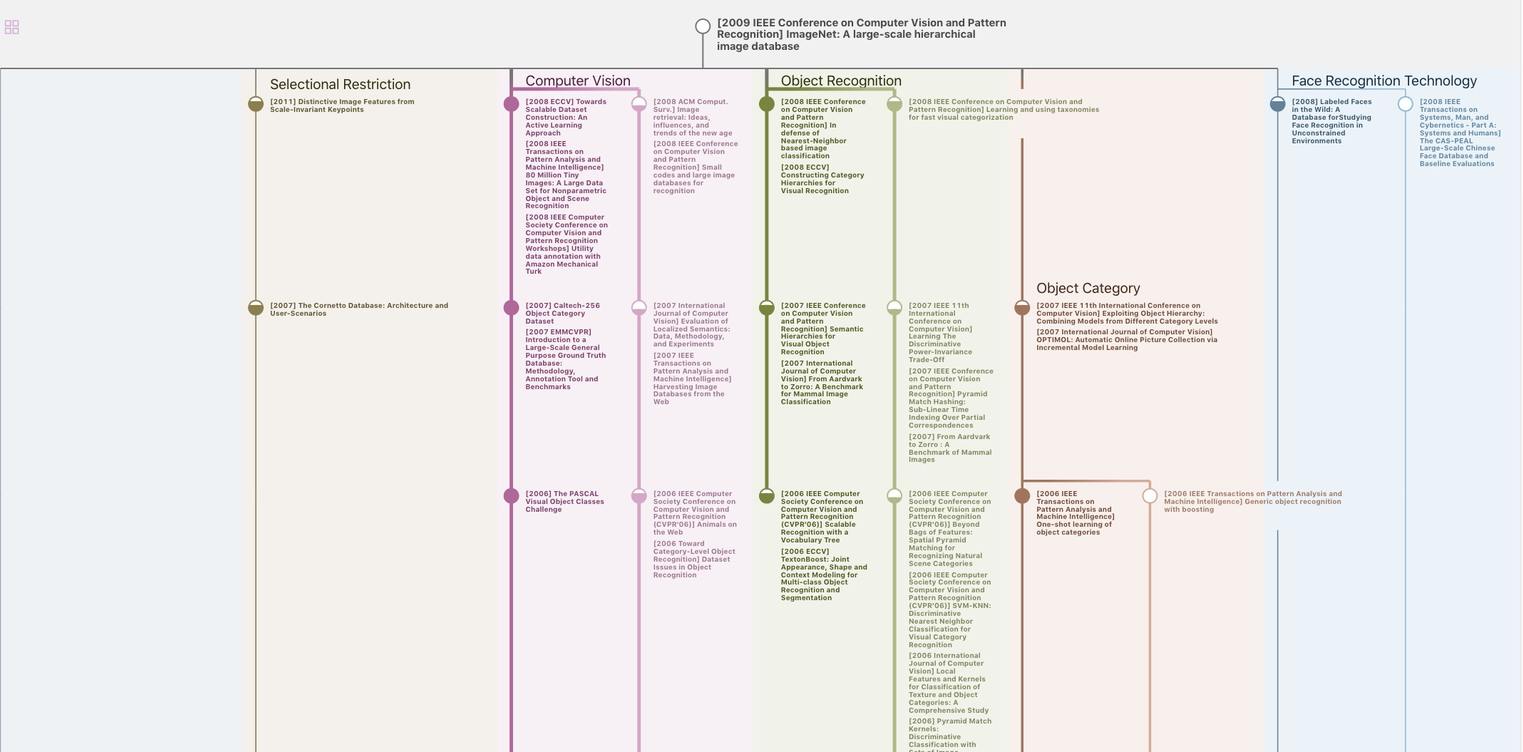
生成溯源树,研究论文发展脉络
Chat Paper
正在生成论文摘要