Deep Neural Network For The Detection Of Early Neoplasia In Barrett'S Oesophagus
Gastrointestinal Endoscopy(2021)
摘要
Introduction Missed oesophageal adenocarcinoma occurs within one year of index endoscopy in a quarter of patients undergoing surveillance for Barrett’s oesophagus (BE). Increased efforts are necessary to improve early neoplasia detection in BE so that early curative endotherapy can be offered. The aim of this study was to develop a deep Neural network that can aid in the diagnosis of early neoplasia in BE. Methods Videos were prospectively collected in high definition white light and i-scan Pentax (Hoya, Japan) imaging modes in patients with dysplastic lesions in BE (high grade dysplasia/intramucosal adenocarcinoma) and patients with non-dysplastic Barrett’s oesophagus. The combination of endoscopic resection margins/targeted biopsy sites and histology served as the ground truth for areas of dysplasia in the videos. All videos with segments of dysplasia were annotated for definite presence of dysplasia. We trained a convolutional neural network with a Resnet101 architecture to classify images into dysplastic or non-dysplastic using randomly selected frames from annotated videos. Results A total of 65 different patients each with a video of BE assessment (28 with HGD/Intramucosal cancer and 37 controls) and a total of 266,930 frames were included in the study. The cases were randomly divided into three independent groups: training (N = 39, 17 dysplasia, 22 controls), testing (N = 7, 3 dysplasia, 4 controls) and independent validation set (N =19, 8 dysplasia, 11 controls). For training, a balanced dataset was created with equal numbers of dysplastic and non-dysplastic frames. All available dysplastic frames were used whereas non-dysplastic frames were chosen randomly. The neural network was trained using a total of 73,266 frames. This was then tested on a balanced testing set of 4228 frames. For the independent validation dataset, all frames in the video sequences were included. This came to a total of 189,436 frames (9194 positive). A dysplastic lesion was only diagnosed if it was deemed present in a sequence of consecutive frames. The neural network classified dysplasia in BE with a sensitivity of 88.26% and specificity of 80.13% in the independent validation set. The area under the ROC was 0.91. Conclusion Preliminary data suggest that a convolutional neural network can be trained to detect dysplastic BE mucosa with accuracies similar to expert endoscopists. The algorithm was created from annotations using whole videos which minimised selection bias. ROC curve analysis for detection of Barrett’s dysplasia (figure 1).
更多查看译文
AI 理解论文
溯源树
样例
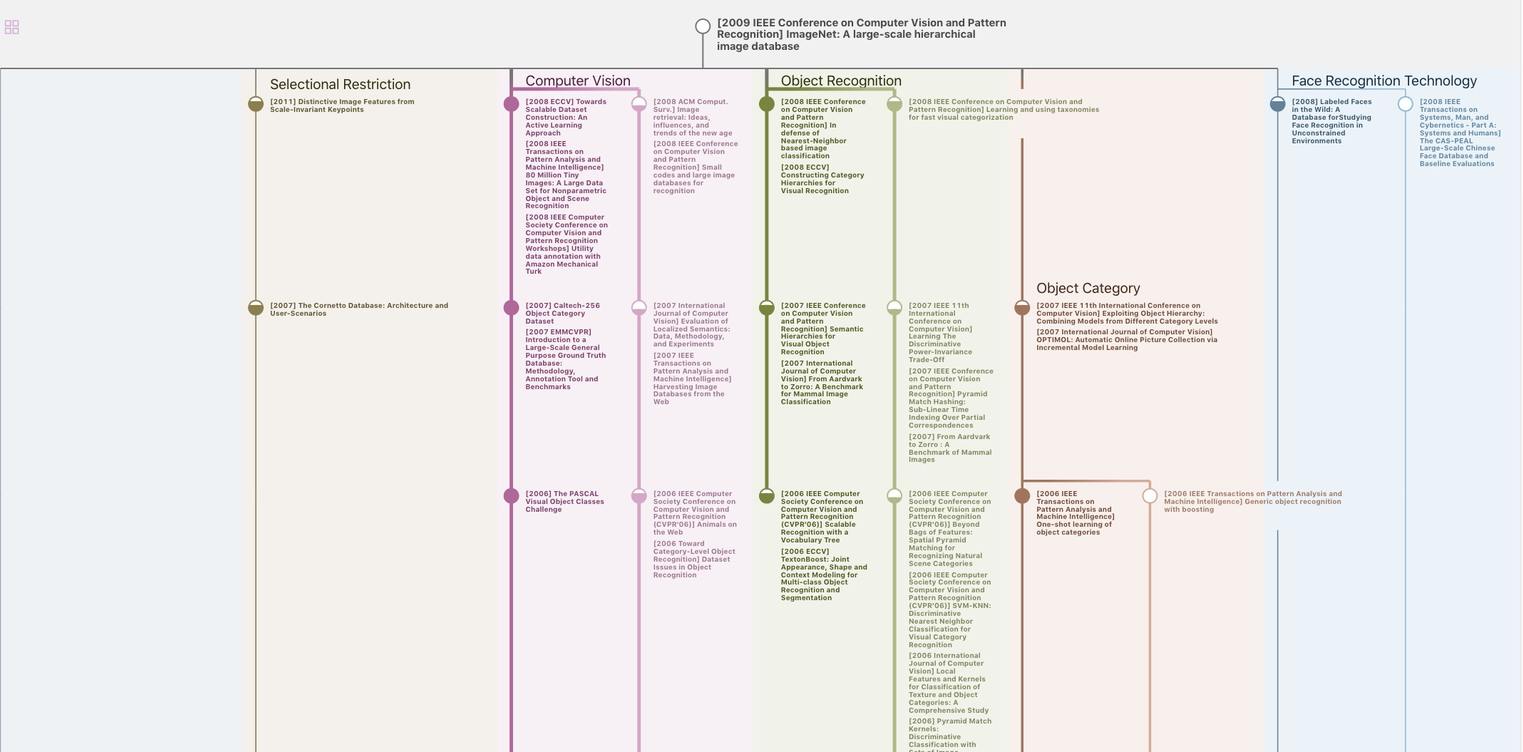
生成溯源树,研究论文发展脉络
Chat Paper
正在生成论文摘要