StatNet: Statistical Image Restoration for Low-Dose CT using Deep Learning
IEEE Journal of Selected Topics in Signal Processing(2020)
摘要
Deep learning has recently attracted widespread interest as a means of reducing noise in low-dose CT (LDCT) images. Deep convolutional neural networks (CNNs) are typically trained to transfer high-quality image features of normal-dose CT (NDCT) images to LDCT images. However, existing deep learning approaches for denoising LDCT images often overlook the statistical property of CT images. In this paper, we propose an approach to statistical image restoration for LDCT using deep learning. We introduce a loss function to incorporate the noise property in image domain derived from the noise statistics in sinogram domain. In order to capture the spatially-varying statistics of CT images, we increase the receptive fields of the neural network to cover full-size CT slices. In addition, the proposed network utilizes $z$-directional correlation by taking multiple consecutive CT slices as input. For performance evaluation, the proposed networks are trained and validated with a public dataset consisting of LDCT-NDCT image pairs. We also perform a retrospective study by testing the networks with clinical LDCT images. The experimental results show that the denoising networks successfully reduce the noise level and restore the image details without adding artifacts. This study demonstrates that the statistical deep learning approach can restore the image quality of LDCT without loss of anatomical information.
更多查看译文
关键词
Low-dose CT,statistical image restoration,convolutional neural network,generative adversarial network,leave-one-out cross-validation,retrospective clinical study
AI 理解论文
溯源树
样例
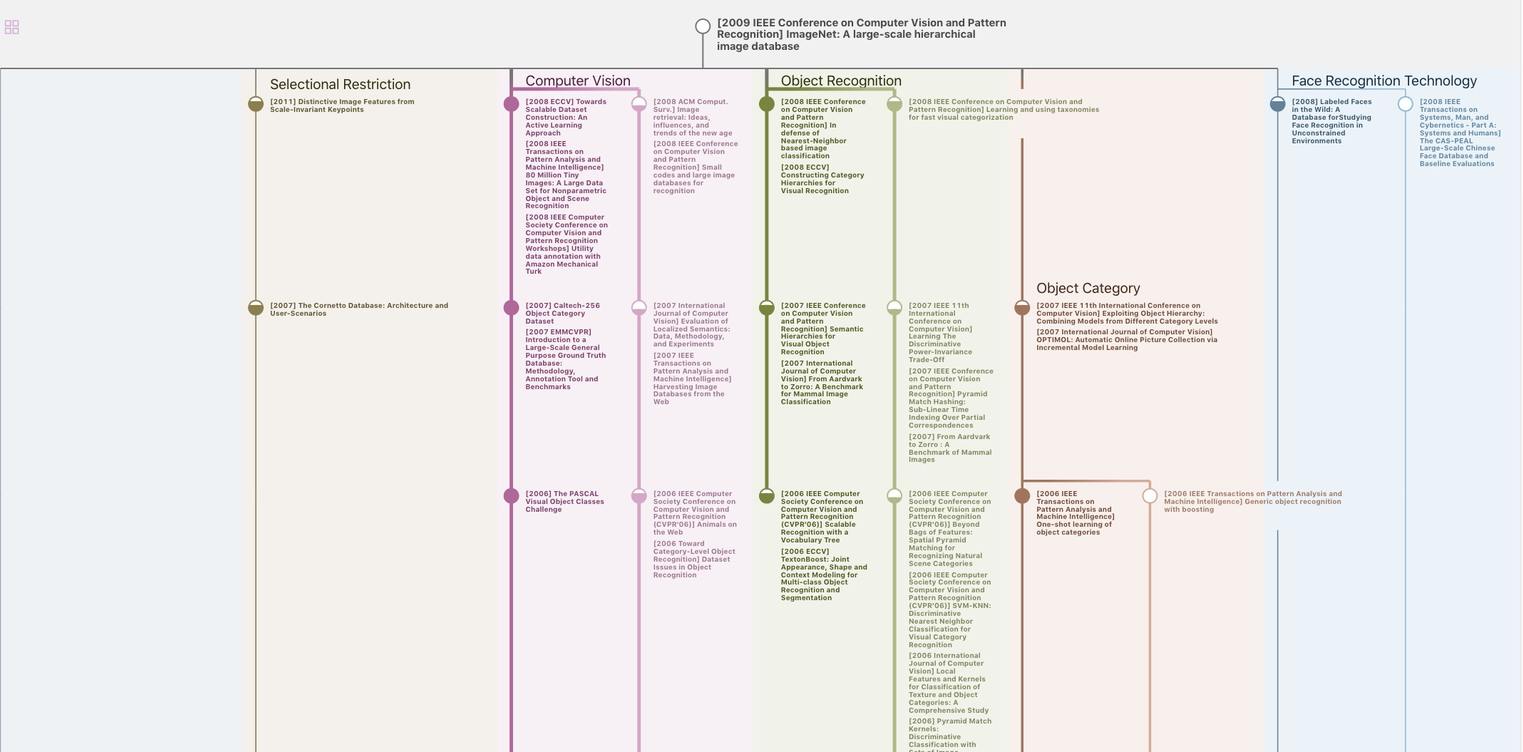
生成溯源树,研究论文发展脉络
Chat Paper
正在生成论文摘要