Variational Denoising Autoencoders And Least-Squares Policy Iteration For Statistical Dialogue Managers
IEEE SIGNAL PROCESSING LETTERS(2020)
摘要
The use of Reinforcement Learning (RL) approaches for dialogue policy optimization has been the new trend for dialogue management systems. Several methods have been proposed, which are trained on dialogue data to provide optimal system response. However, most of these approaches exhibit performance degradation in the presence of noise, poor scalability to other domains, as well as performance instabilities. To overcome these problems, we propose a novel approach based on the incremental, sample-efficient Least-Squares Policy Iteration (LSPI) algorithm, which is trained on compact, fixed-size dialogue state encodings, obtained from deep Variational Denoising Autoencoders (VDAE). The proposed scheme exhibits stable and noise-robust performance, which significantly outperforms the current state-of-the-art, even in mismatched noise environments.
更多查看译文
关键词
Noise reduction, Signal processing algorithms, Encoding, Training, Optimization, Approximation algorithms, Degradation, Variational autoencoders, denoising, dialogue systems, sample-efficient statistical dialogue managers, least-squares policy iteration
AI 理解论文
溯源树
样例
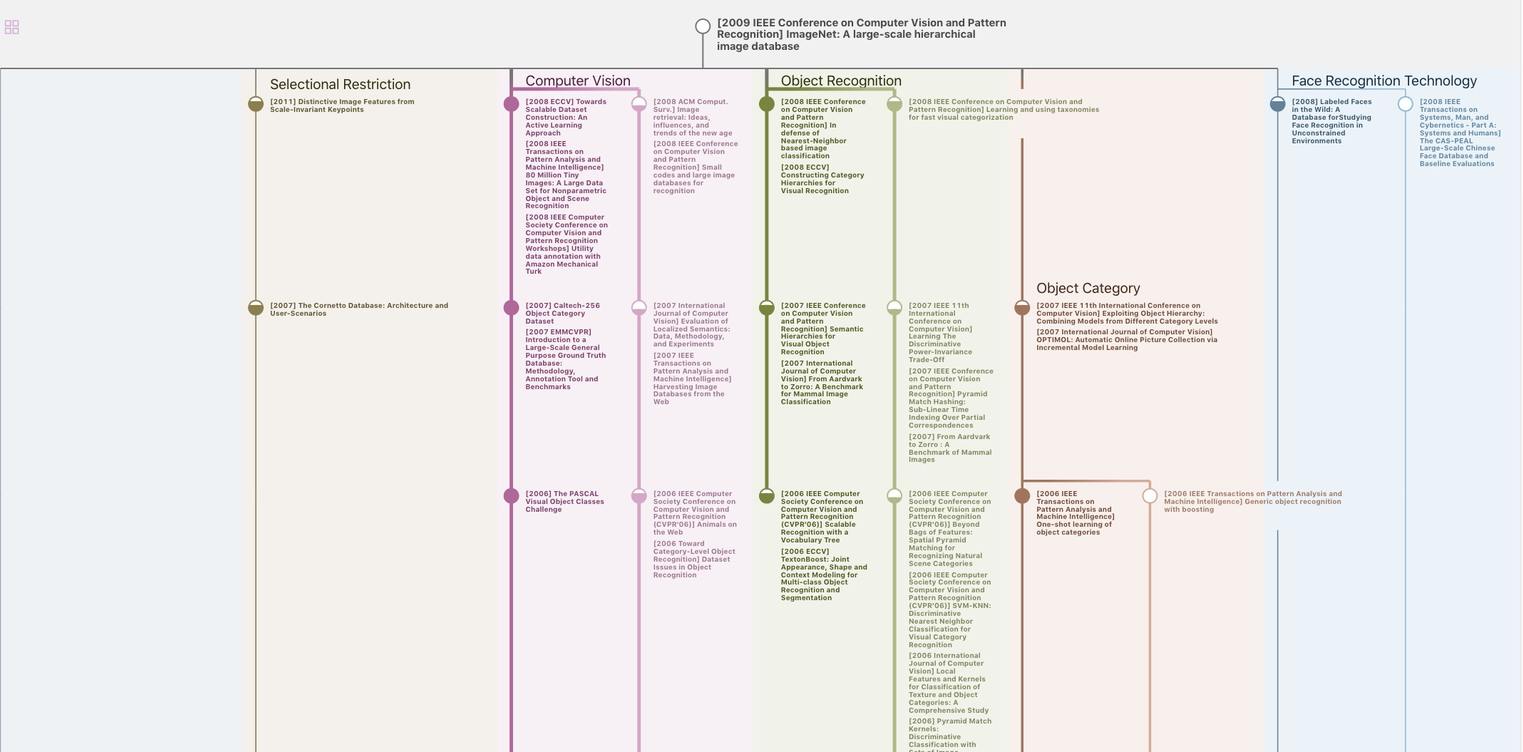
生成溯源树,研究论文发展脉络
Chat Paper
正在生成论文摘要