Online multi-object tracking with pedestrian re-identification and occlusion processing
The Visual Computer(2020)
摘要
Tracking-by-detection is a common approach for online multi-object tracking problem. At present, the following challenges still exist in the multi-object tracking scenarios: (1) The result of object re-tracking after full occlusion is not ideal; (2) The predicted position of object is not accurate enough in the complicated video scenarios. Aiming at these two problems, this paper proposes a multi-object tracking framework called DROP (Deep Re-identification Occlusion Processing). The framework consists of object detection, fast pedestrian re-identification, and a confidence-based data association algorithm. A lightweight convolutional neural network that can solve the re-tracking problem is constructed by increasing and learning the affinity of appearance features of the same object in different frames. And this paper proposes to judge the occlusion of the object that can solve inaccurate position predicted by Kalman filter by using the data association result of the appearance features of the object, and to reduce the matching error by improving the data association formula. The experimental results on the multi-object tracking datasets MOT15 and MOT16 show that the proposed method can improve the precision while ensure the real-time tracking performance.
更多查看译文
关键词
Multi-object tracking,Tracking-by-detection,Pedestrian re-identification,Data association
AI 理解论文
溯源树
样例
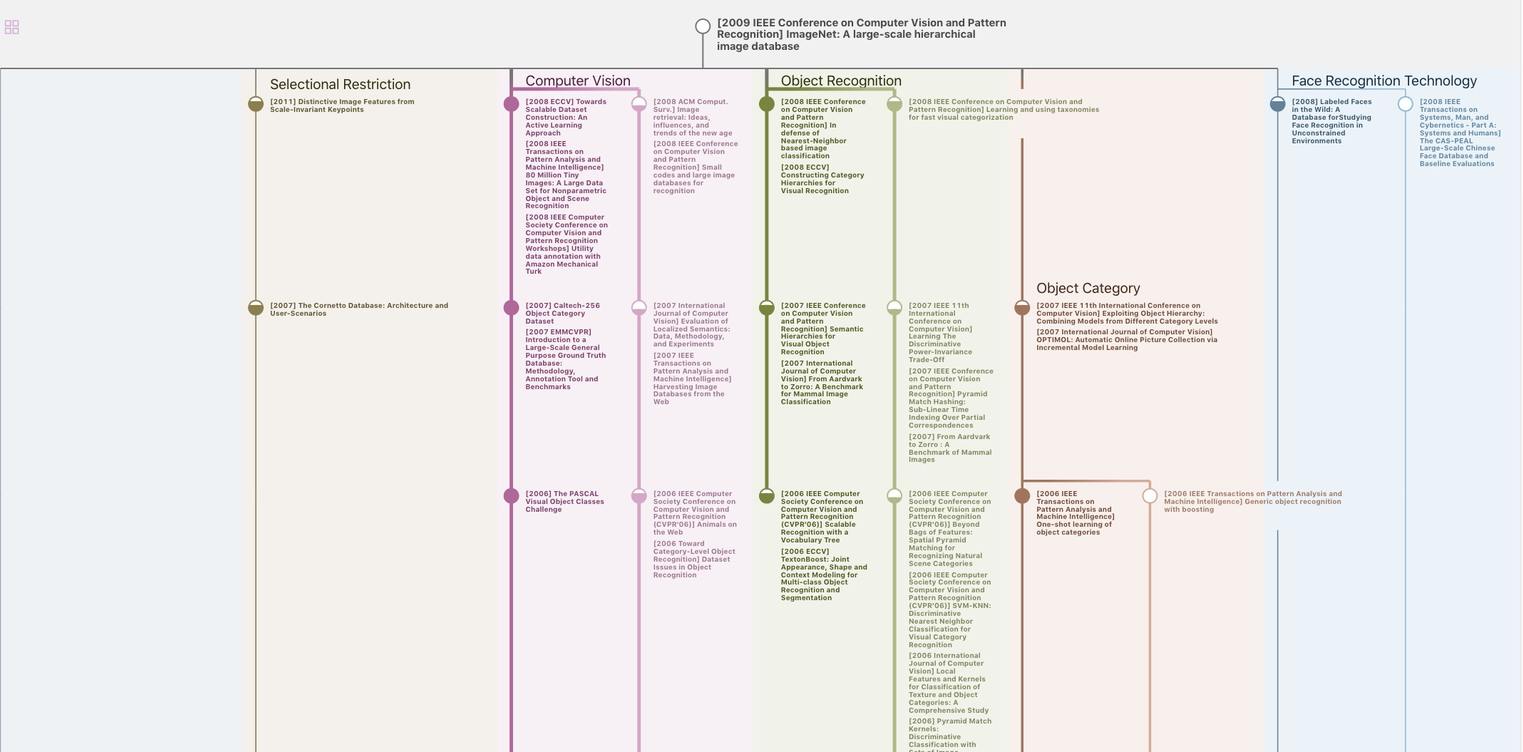
生成溯源树,研究论文发展脉络
Chat Paper
正在生成论文摘要