Data Efficient Segmentation of Various 3D Medical Images Using Guided Generative Adversarial Networks
IEEE ACCESS(2020)
摘要
The recent significant increase in accuracy of medical image processing is attributed to the use of deep neural networks as manual segmentation generates errors in interpretation besides, is very arduous and inefficient. Generative adversarial networks (GANs) is a particular interest to medical researchers, as it implements adversarial loss without explicit modeling of the probability density function. Medical image segmentation methods face challenges of generalization and over-fitting, as medical data suffers from various shapes and diversity of organs. Furthermore, generating a sufficiently large annotated dataset at a clinical site is costly. To generalize learning with a small amount of training data, we propose guided GANs (GGANs) that can decimate samples from an input image and guide networks to generate images and corresponding segmentation mask. The decimated sampling is the key element of the proposed method employed to reduce network size using only a few parameters. Moreover, this method yields promising results by generating several outputs, such as bagging approach. Furthermore, errors of loss function increase, during the generation of original images and corresponding segmentation mask, in comparison to generating only the segmentation mask. Minimization of increased error leads (GGANs) to enhance the performance of segmentation using smaller datasets and less testing time. This method can be applied to a wide range of segmentation problems for different modalities and various organs (such as aortic root, left atrium, knee cartilage, and brain tumors) during a real-time crisis in hospitals. The proposed network also yields high accuracy compared to state-of-the-art networks.
更多查看译文
关键词
Image segmentation,Biomedical imaging,Gallium nitride,Tumors,Generative adversarial networks,Shape,Valves,Medical image segmentation,generative adversarial network,aortic valve,left atrium,knee cartilage,brain tumor,decimated sample
AI 理解论文
溯源树
样例
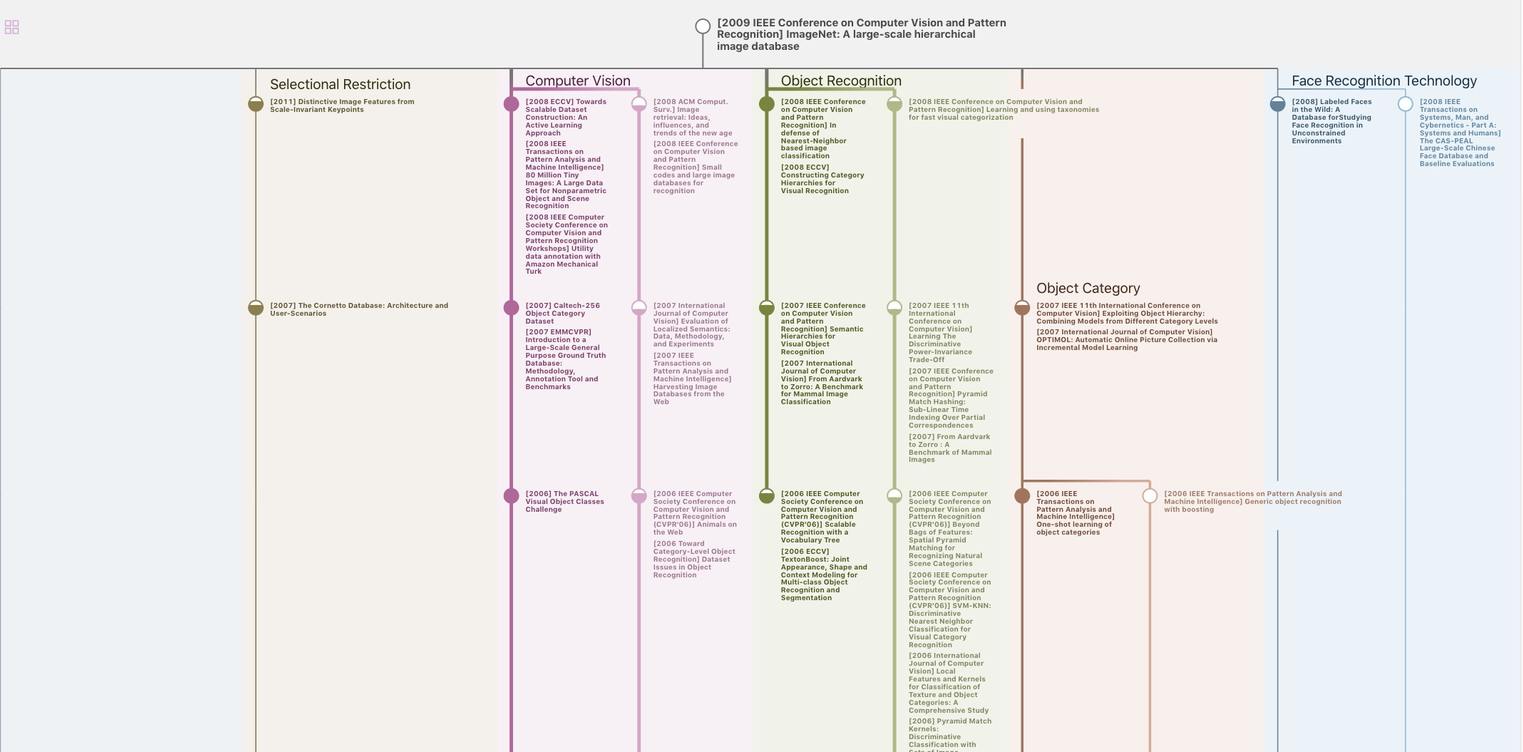
生成溯源树,研究论文发展脉络
Chat Paper
正在生成论文摘要