Dual Pyramid Attention Network for High-resolution Remotely Sensed Image Change Detection
ICMLC(2020)
摘要
Binary change detection in bi-temporal high-resolution images is of significance in many fields, such as assessment of forest resources, territorial resources surveys and urban planning. The existing change detection methods can be divided into unsupervised methods and supervised methods, and supervised strategies, such as deep learning (DL) algorithms tend to yield better effectiveness than unsupervised methods. Nevertheless, present change detection methods cannot tackle the complexity of remote sensing images, including the diversity of change condition and sophisticated external disturbance. In this paper, we propose a feature pyramid module (FPM) and global attention mechanism module (GAMM) for change detection in highresolution images. The proposed FPM is able to enrich semantic information in feature extraction procedure, and the proposed GAMM is capable of emphasizing difference information learning. Based on the two proposed models, a novel dual pyramid attention network (DPANet) is developed for supervised change detection in bi-temporal high resolution images. For our change detection method, the end-to-end Siamese fully convolution network DPANet is trained in fixed size of bi-temporal image pair, and output a binary pixel-level detection result. The experimental results with diversified data sets have verified that our method has achieved better change detection accuracy than other alternative DL-based methods and several unsupervised algorithms.
更多查看译文
关键词
Dual Pyramid Attention Network (DPANet), change detection, bi-temporal images, deep learning
AI 理解论文
溯源树
样例
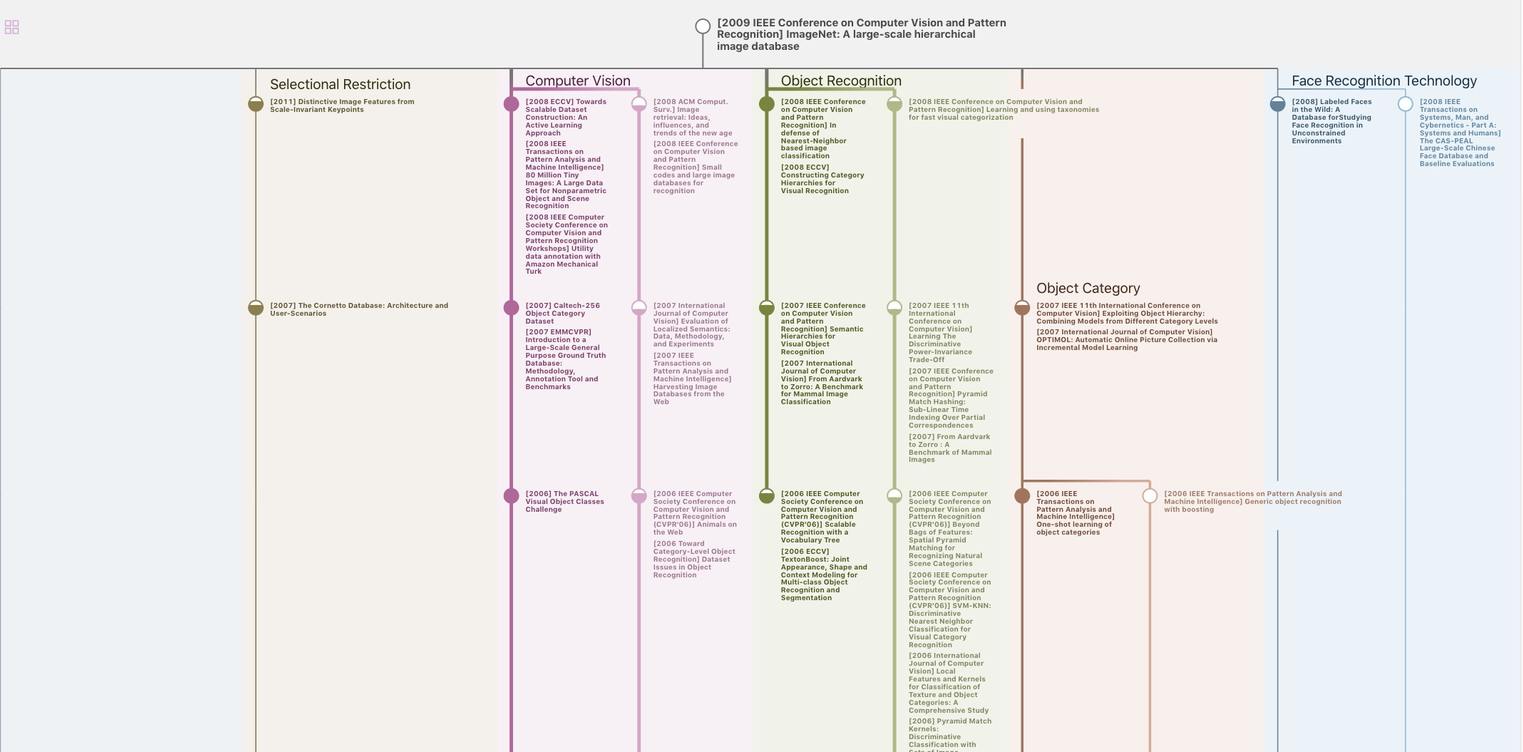
生成溯源树,研究论文发展脉络
Chat Paper
正在生成论文摘要