Cardiomegaly Detection On Chest Radiographs: Segmentation Versus Classification
IEEE ACCESS(2020)
摘要
In this study, we investigate the detection of cardiomegaly on frontal chest radiographs through two alternative deep-learning approaches - via anatomical segmentation and via image-level classification. We used the publicly available ChestX-ray14 dataset, and obtained heart and lung segmentation annotations for 778 chest radiographs for the development of the segmentation-based approach. The classification-based method was trained with 65k standard chest radiographs with image-level labels. For both approaches, the best models were found through hyperparameter searches where architectural, learning, and regularization related parameters were optimized systematically. The resulting models were tested on a set of 367 held-out images for which cardiomegaly annotations were hand-labeled by two independent expert radiologists. Sensitivity, specificity, positive predictive value, negative predictive value, and area under the receiver operating characteristic curve (AUC) were calculated. The performance of the segmentation-based system with an AUC of 0.977 is significantly better for classifying cardiomegaly than the classification-based model which achieved an AUC of 0.941. Only the segmentation-based model achieved comparable performance to an independent expert reader (AUC of 0.978). We conclude that the segmentation-based model requires 100 times fewer annotated chest radiographs to achieve a substantially better performance, while also producing more interpretable results.
更多查看译文
关键词
Image segmentation, Heart, Lung, Radiography, Training, Standards, Machine learning, Deep learning, chest radiographs, anatomy segmentations, cardiomegaly
AI 理解论文
溯源树
样例
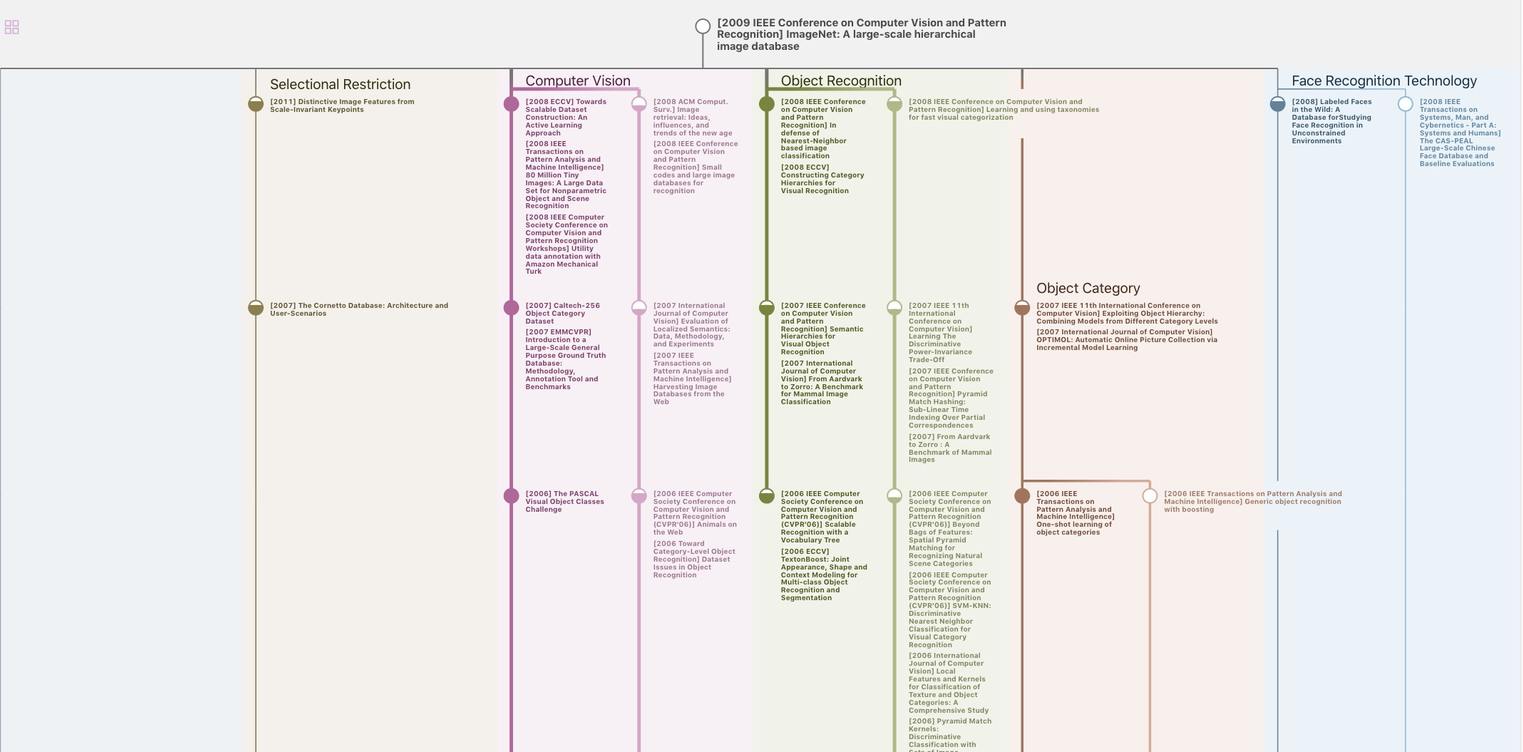
生成溯源树,研究论文发展脉络
Chat Paper
正在生成论文摘要