Assessing Information Quality And Distinguishing Feature Subsets For Molecular Classification
PROCEEDINGS OF 2020 10TH INTERNATIONAL CONFERENCE ON BIOSCIENCE, BIOCHEMISTRY AND BIOINFORMATICS (ICBBB 2020)(2020)
摘要
A feature reduction scheme is developed in this study. The proposed scheme is a hybrid of unsupervised and supervised methods. The unsupervised process is designed to exclude the irrelevant and useless information before executing feature selection. Fuzzy clustering algorithm will categorize all features into different groups. Then, the supervised process using the target class information will purify the relevant and informative factors from the approved feature clusters. This purifying procedure is particularly suitable for high dimensional datasets with erroneous information. This proposed strategy is to retain the advantage of simplicity and lower selecting time consumption in filter methods while the disadvantage of high computational cost due to the exponential quantity of feature subsets in wrapper methods is completely avoided.
更多查看译文
关键词
Feature selection, Relevance analysis, Fuzzy c-means, Microarray analysis
AI 理解论文
溯源树
样例
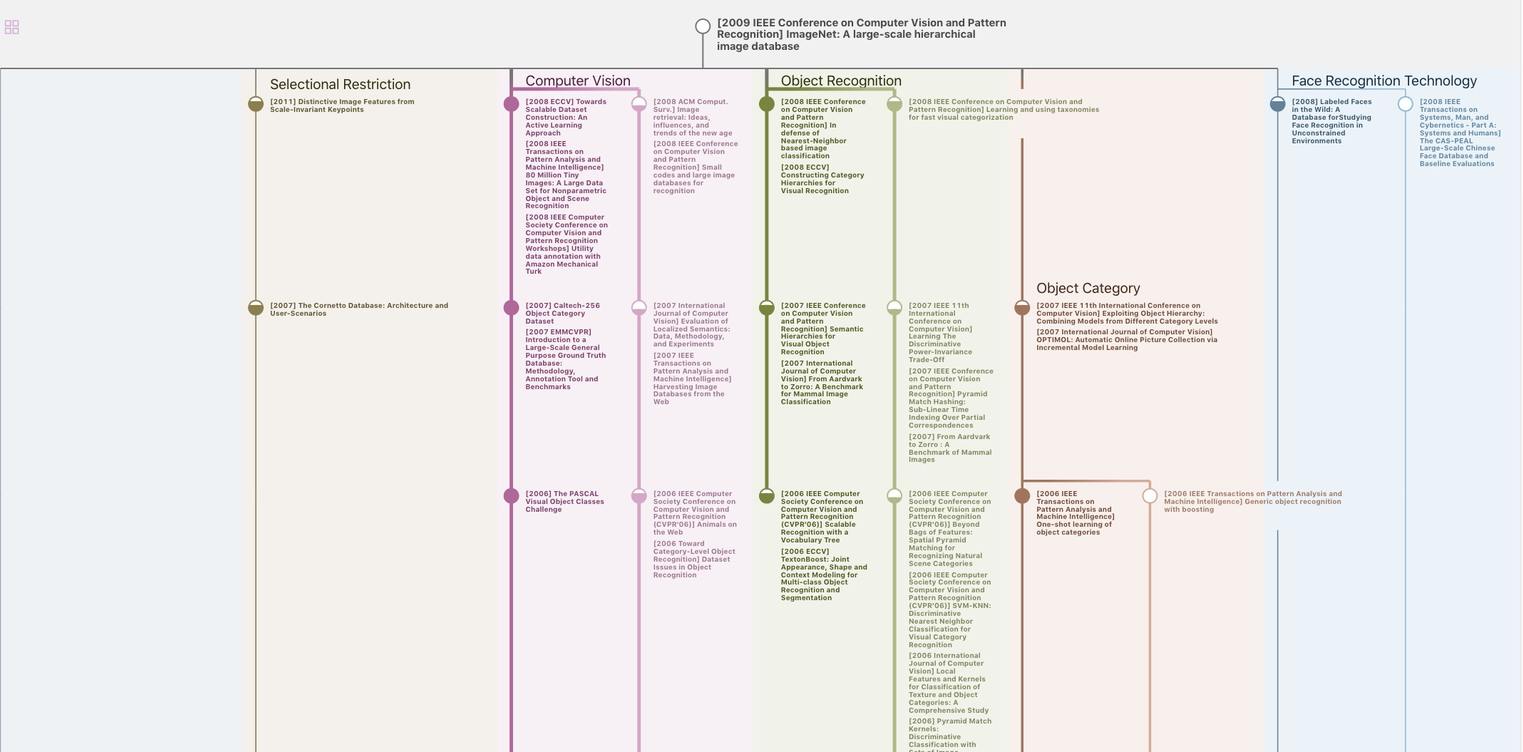
生成溯源树,研究论文发展脉络
Chat Paper
正在生成论文摘要