Deep Convolutional Neural Networks for Brain Tumor Segmentation: Boosting Performance Using Deep Transfer Learning: Preliminary Results
BrainLes@MICCAI (2)(2019)
摘要
Brain tumor segmentation through MRI images analysis is one of the most challenging issues in medical field. Among these issues, Glioblastomas (GBM) invade the surrounding tissue rather than displacing it, causing unclear boundaries, furthermore, GBM in MRI scans have the same appearance as Gliosis, stroke, inflammation and blood spots. Also, fully automatic brain tumor segmentation methods face other issues such as false positive and false negative regions. In this paper, we present new pipelines to boost the prediction of GBM tumoral regions. These pipelines are based on 3 stages, first stage, we developed Deep Convolutional Neural Networks (DCNNs), then in second stage we extract multi-dimensional features from higher-resolution representation of DCNNs, in third stage we developed machine learning algorithms, where we feed the extracted features from DCNNs into different algorithms such as Random forest (RF) and Logistic regression (LR), and principal component analysis with support vector machine (PCA-SVM). Our experiment results are reported on BRATS-2019 dataset where we achieved through our proposed pipelines the state-of-the-art performance. The average Dice score of our best proposed brain tumor segmentation pipeline is 0.85, 0.76, 0.74 for whole tumor, tumor core, and enhancing tumor, respectively. Finally, our proposed pipeline provides an accurate segmentation performance in addition to the computational efficiency in terms of inference time makes it practical for day-to-day use in clinical centers and for research.
更多查看译文
关键词
Brain tumor segmentation, Convolutional Neural Networks, Support vector machine, Glioblastomas, Transfer learning, Principal component analysis
AI 理解论文
溯源树
样例
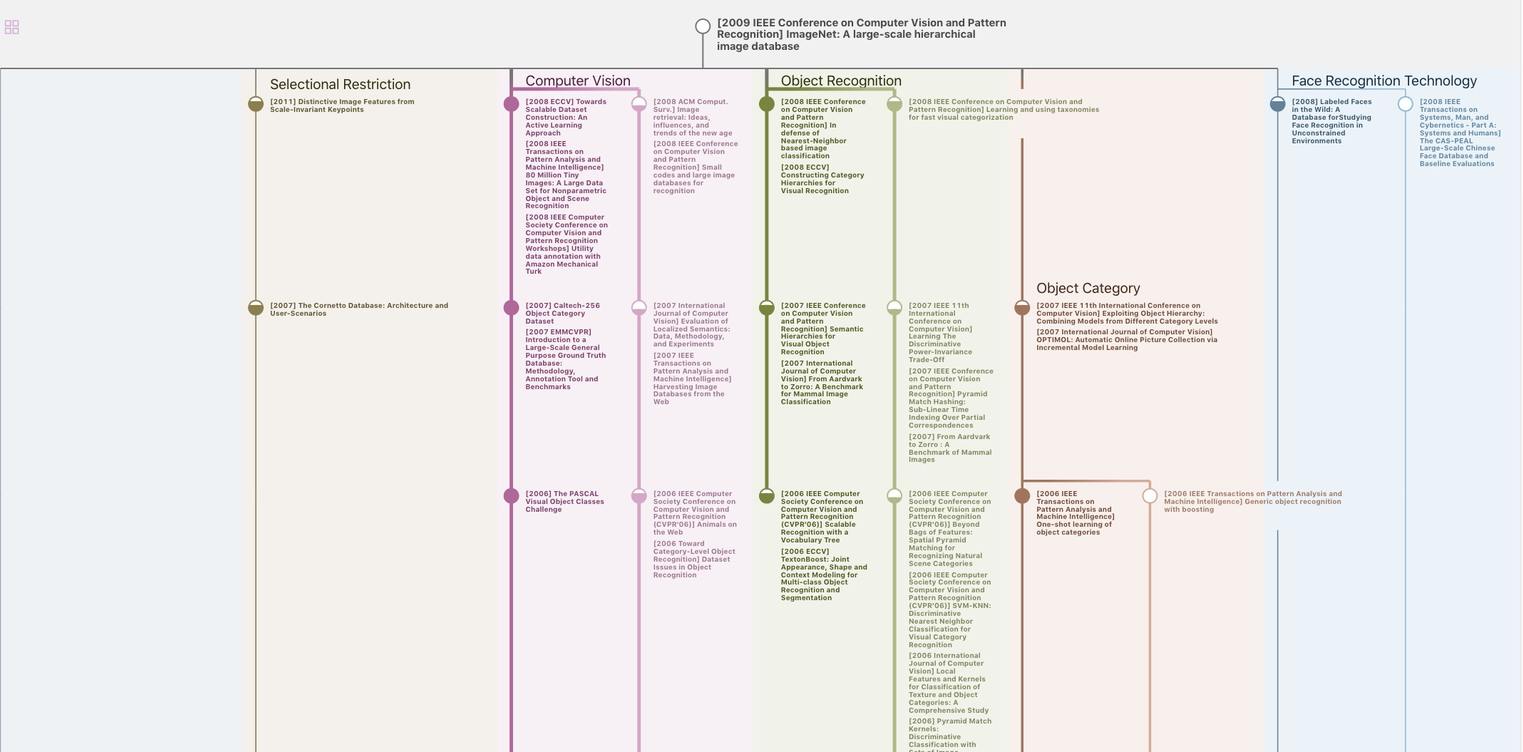
生成溯源树,研究论文发展脉络
Chat Paper
正在生成论文摘要