Efficient Low-Resolution Face Recognition Via Bridge Distillation
IEEE TRANSACTIONS ON IMAGE PROCESSING(2020)
摘要
Face recognition in the wild is now advancing towards light-weight models, fast inference speed and resolution-adapted capability. In this paper, we propose a bridge distillation approach to turn a complex face model pretrained on private high-resolution faces into a light-weight one for low-resolution face recognition. In our approach, such a cross-dataset resolution-adapted knowledge transfer problem is solved via two-step distillation. In the first step, we conduct cross-dataset distillation to transfer the prior knowledge from private high-resolution faces to public high-resolution faces and generate compact and discriminative features. In the second step, the resolution-adapted distillation is conducted to further transfer the prior knowledge to synthetic low-resolution faces via multi-task learning. By learning low-resolution face representations and mimicking the adapted high-resolution knowledge, a light-weight student model can be constructed with high efficiency and promising accuracy in recognizing low-resolution faces. Experimental results show that the student model performs impressively in recognizing low-resolution faces with only 0.21M parameters and 0.057MB memory. Meanwhile, its speed reaches up to 14,705, 934 and 763 faces per second on GPU, CPU and mobile phone, respectively.
更多查看译文
关键词
Face recognition in the wild, two-stream architecture, knowledge distillation, CNNs
AI 理解论文
溯源树
样例
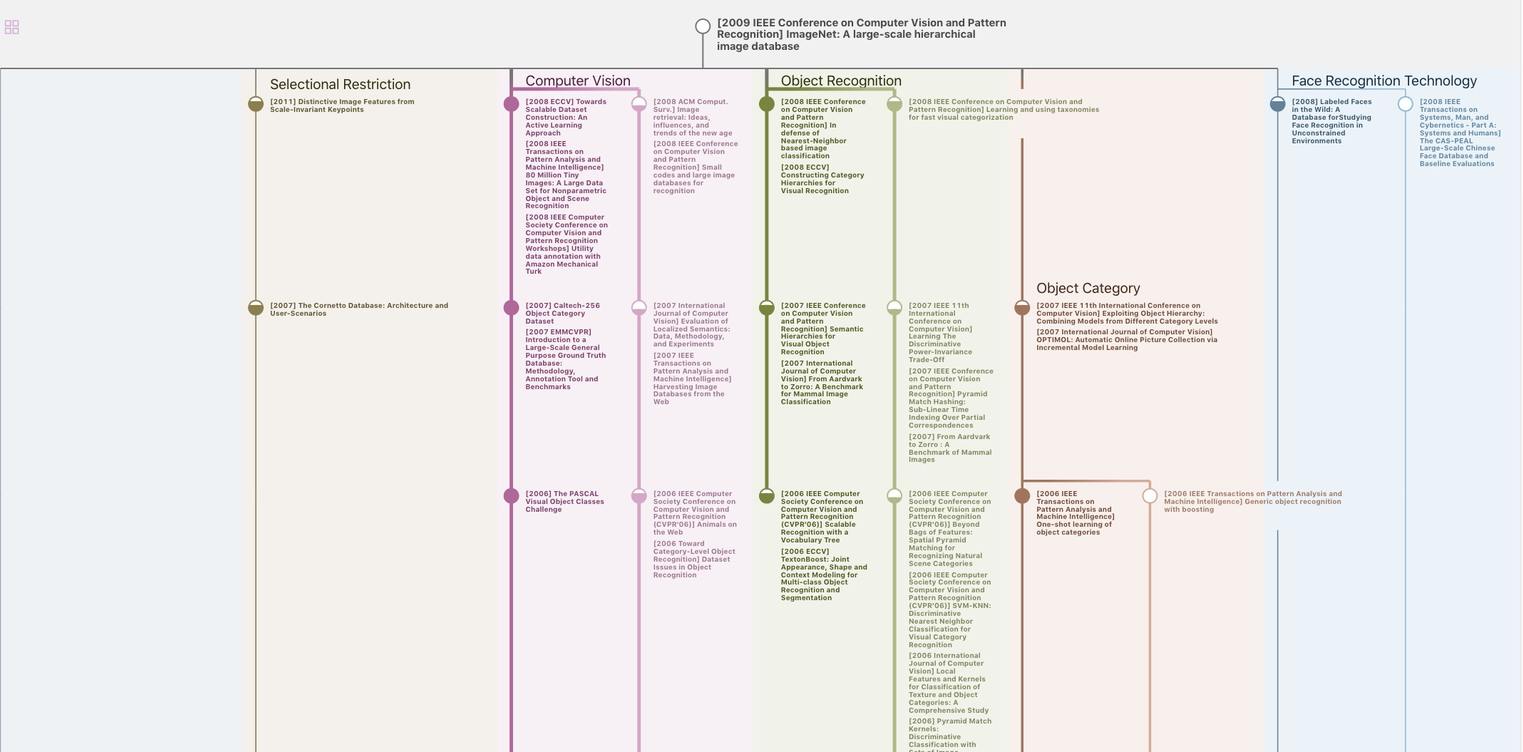
生成溯源树,研究论文发展脉络
Chat Paper
正在生成论文摘要