Application-Oriented Scheduling for Optimizing the Age of Correlated Information: A Deep-Reinforcement-Learning-Based Approach
IEEE Internet of Things Journal(2020)
摘要
Recent advances in communications technologies and the proliferation of connected devices have spawned a variety of information-centric Internet-of-Things (IoT) systems, where timely information updating is normally required. Age of Information (AoI) has recently been introduced to quantify the freshness of the knowledge the controller has about the remote information sources. With the development of IoT applications, it is becoming increasingly common that the application-level services, e.g., status updates, rely on the timely delivery of fresh information from a number of information sources. In this article, we study the application-oriented scheduling for optimizing information freshness in the presence of correlated information sources. To this end, we adopt the concept of Age of Correlated Information (AoCI) and formulate the scheduling problem as an episodic Markov decision process (MDP) problem with an application-oriented policy. Given the complexity of the above problem, we develop a learning-based approach that not only leverages the emerging deep reinforcement learning (DRL) techniques but also exploits diverse-domain knowledge. The numerical results show that the proposed approach achieves better performance in terms of AoCI, compared to some typical baseline methods.
更多查看译文
关键词
Age of Information (AoI),deep reinforcement learning (DRL),information correlations,scheduling
AI 理解论文
溯源树
样例
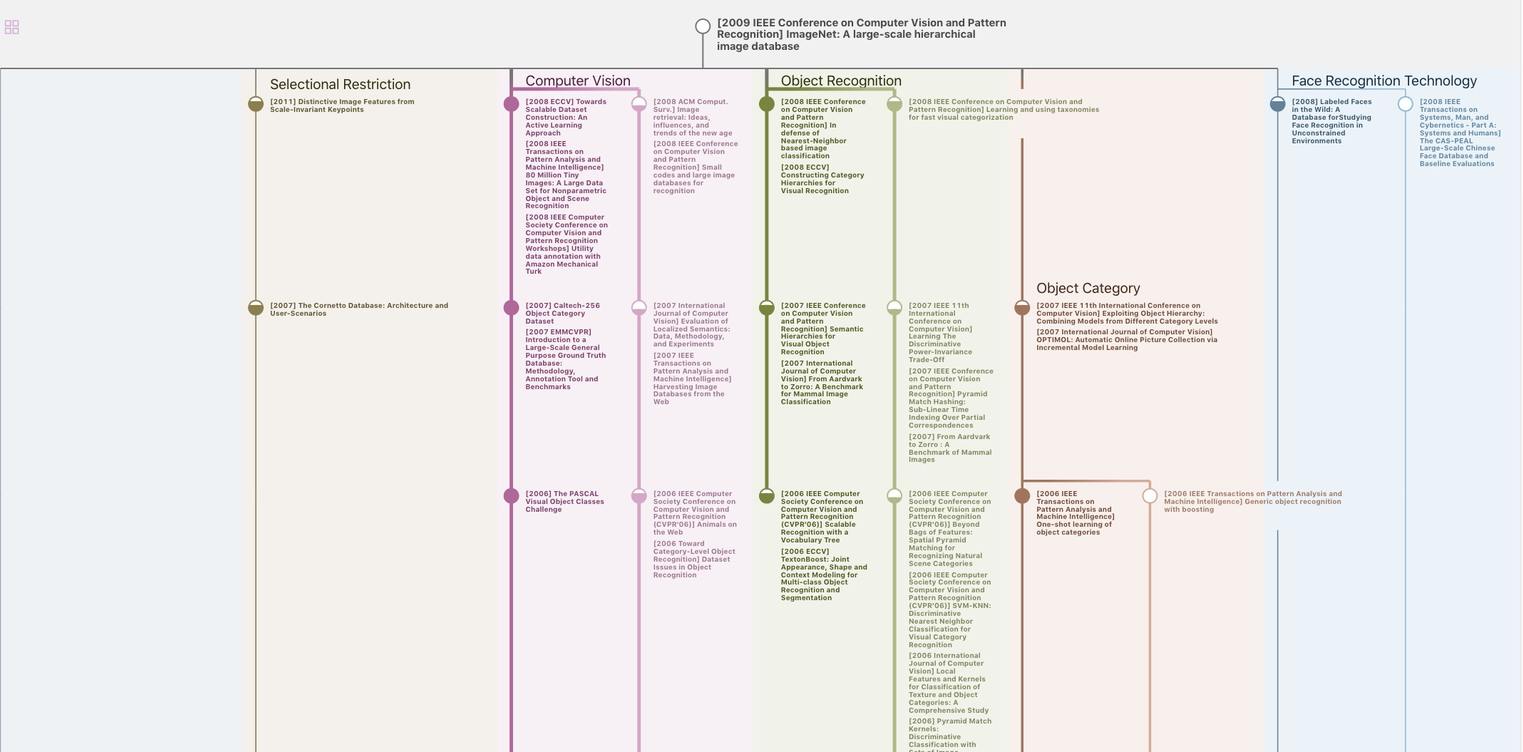
生成溯源树,研究论文发展脉络
Chat Paper
正在生成论文摘要