Deep Convolutional Neural Network Assisted Reinforcement Learning Based Mobile Network Power Saving
IEEE ACCESS(2020)
摘要
This paper addresses the power saving problem in mobile networks. Base station (BS) power and network traffic volume (NTV) models are first established. The BS power is modeled based on in-house equipment measurement by sampling different BS load configurations. The NTV model is built based on traffic data in the literature. Then, a threshold-based adaptive power saving method is discussed, serving as the benchmark. Next, a BS power control framework is created using Q-learning. The action-state function of the Q-learning is approximated via a deep convolutional neural network (DCNN). The DCNN-Q agent is designed to control the loads of cells in order to adapt to NTV variations and reduce power consumption. The DCNN-Q power saving framework is trained and simulated in a heterogeneous network including macrocells and microcells. It can be concluded that with the proposed DCNN-Q method, the power saving outperforms the threshold-based method.
更多查看译文
关键词
Load modeling, Power measurement, Power demand, Data models, Solid modeling, Adaptation models, Manganese, Power saving, deep convolutional neural network, reinforcement learning
AI 理解论文
溯源树
样例
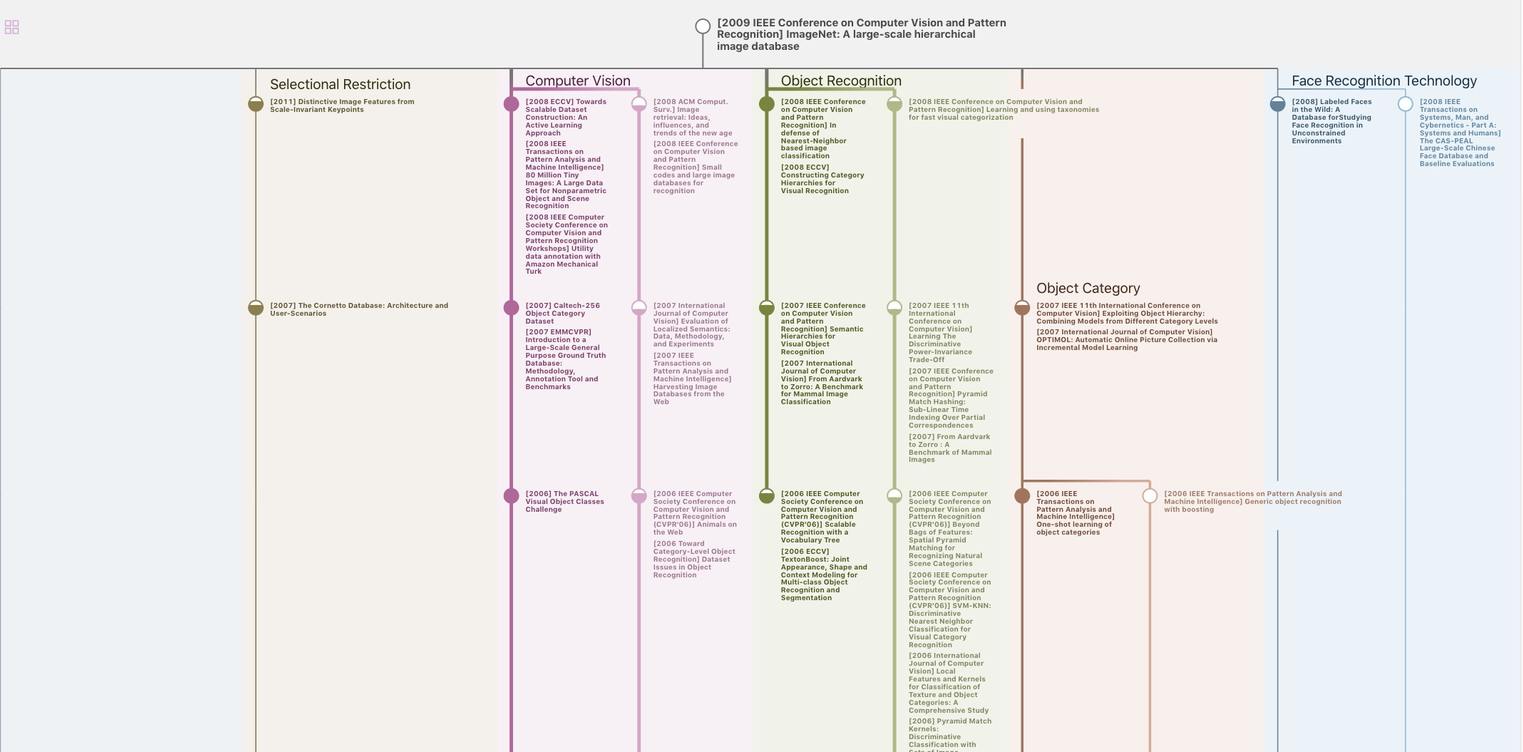
生成溯源树,研究论文发展脉络
Chat Paper
正在生成论文摘要