Context-aware colorization of gray-scale images utilizing a cycle-consistent generative adversarial network architecture
Neurocomputing(2020)
摘要
Converting gray-scale images to colorful ones is one of the challenging tasks in the Computer Vision area, and various approaches based on neural network architectures have been proposed to generate colorful images. However, most of the suggested colorization frameworks use a single model for colorization regardless of the diversity of colors in images of various datasets. We claim that since such a structure is responsible for generating all types of images, it results in producing either faint-colored images or some artifacts in the images. We addressed this issue by proposing parallel colorization models, each of which is customized for generating images with similar contexts or color themes. Our proposed architecture consists of two stages as follows. The first stage includes a single colorization network that generates an initial colored image and simultaneously selects one of the specialist networks of the second stage for each image. The second stage consists of several specialist networks, whose tasks are improving the quality of the initial colored image and produce real-looking, plausible images. Our goal is to assign images to specialist networks in a way that each one of the specialist networks deals with contextually similar images so that the networks become more confident in generating vibrant colors and high-quality images. Cycle-Consistent Generative Adversarial Network structure is utilized in our architecture since it has demonstrated great potential in creating plausible images in the literature. The proposed model is evaluated on the ImageNet dataset, and the results stand for the effectiveness of employing context-aware specialists for colorization.
更多查看译文
关键词
Context-aware specialist network,Generative adversarial network,Image colorization,Two-stage architecture
AI 理解论文
溯源树
样例
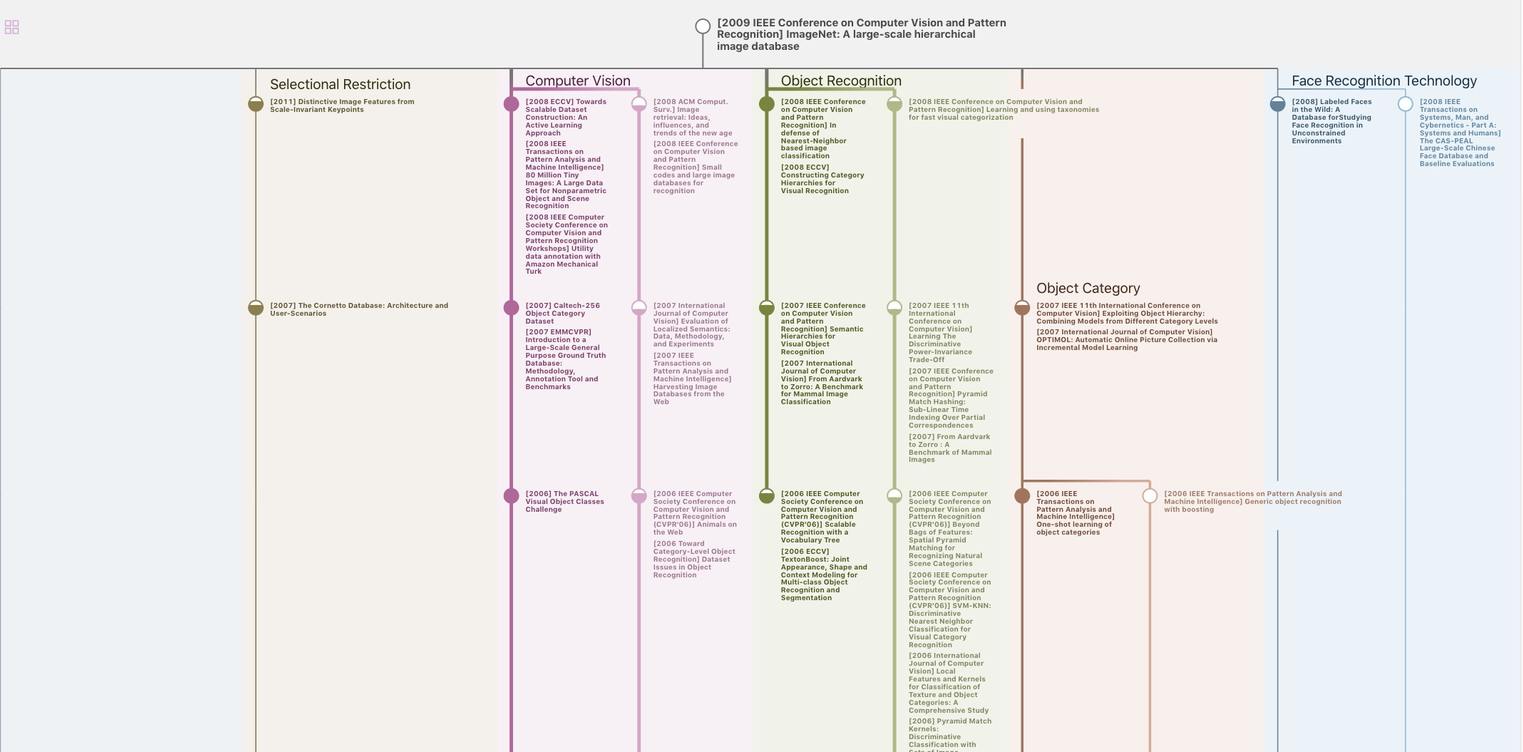
生成溯源树,研究论文发展脉络
Chat Paper
正在生成论文摘要